U-MATH & μ-MATH: Assessing LLMs on university-level math
Why U-MATH?
U-MATH is the largest university-level math benchmark, designed to address the gap in existing math benchmarks, which are limited in scope and size.
— 1,100 problems from math courses across 6 key subject areas
— 20% of tasks include images to challenge LLMs
— Practical applications for industry and education
What is µ-MATH?
The Meta-Evaluation Benchmark is a set of 1084 meta-evaluation solutions designed to rigorously assess the quality of LLM judges, based on U-MATH problems.
Together, these benchmarks support comprehensive evaluation of LLM proficiency on university-level math.
LLM leaderboard on U-Math
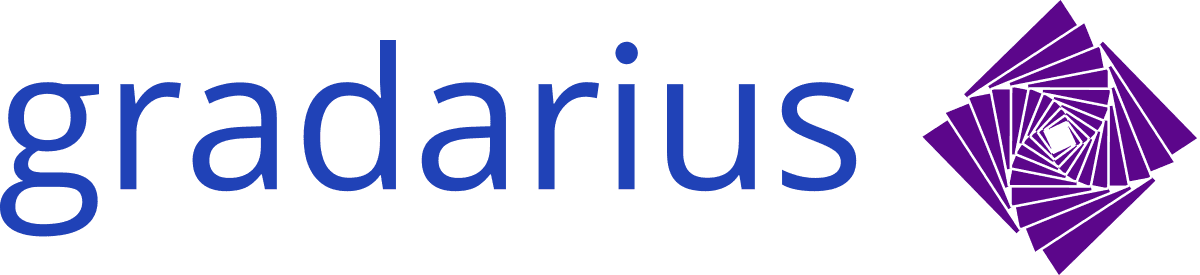
Both U-Math and μ-MATH datasets were collected with the help of Gradarius - a learning platform that helps students master calculus through a step-by-step approach, providing immediate feedback to guide them through problem-solving.
Insights into LLM performance on U-Math
University-level problems pose a challenge to standard-inference models, but reasoning systems boast breakthrough performance.
Open-weight models are rapidly closing the gap on text-only tasks, but continue to lag on multi-modal problems.
Integrating vision proves tough: U-MATHv scores lag significantly behind text-only performance, and adding visual capabilities to a model typically leads to degradation.
Gemini models are most adept at visual reasoning, consistently leading in U-MATHv score within all the model groups.
Continuous training pushes the models forward: the Athene fine-tune of Qwen 2.5 and Nemotron fine-tune of Llama 3.1 deliver improvements across the board.
Specialization trumps size: domain-specific models such as Qwen Math beat models an order of magnitude larger.
Accuracy scores on our U-MATH benchmark and its constituent subject splits. For each category (overall and subject-specific scores) two numbers are provided: separately for text-only (T) and visual (V) problems. Additionaly, an overall score across all the subjects excluding Algebra and Precalculus is shown under U-MATH THard. Asterisks denote small sample sizes (<15). Bold indicates the best result within each group.
All the models are used with their latest versions available as of 2025-03-15. Greedy decoding is employed for all the models (except for OpenAI o-series), with ablations performed to ensure no performance degradation occurs compared to sampling-based inference. Images are not included in the prompt for text-only models, only the problem statements. Free-form solutions are verified against golden labels by an ensemble of reasoning models.
What makes
U-MATH stand out
Challenging problems to test deep understanding
and advanced reasoning skills.
Covers 6 subjects: Algebra, Precalculus, Differential Calculus, Integral Calculus, Multivariable Calculus, Sequences & Series.
Problems and solutions are sourced from real coursework and checked by experts to meet academic standards.
Existing auto-evaluated mathematical benchmarks together with their test set sizes, percentages of visual problems, and percentages of free-form answers. Additionaly, percentages of university-curricula specific problems are provided. Some of the problems in U-MATH and μ-MATH are sourced from OpenStax under CC BY 4.0.
U-Math Dataset Collection Process
To identify the most challenging problems from tens of thousands of examples available for our benchmark we used a multi-stage selection process:
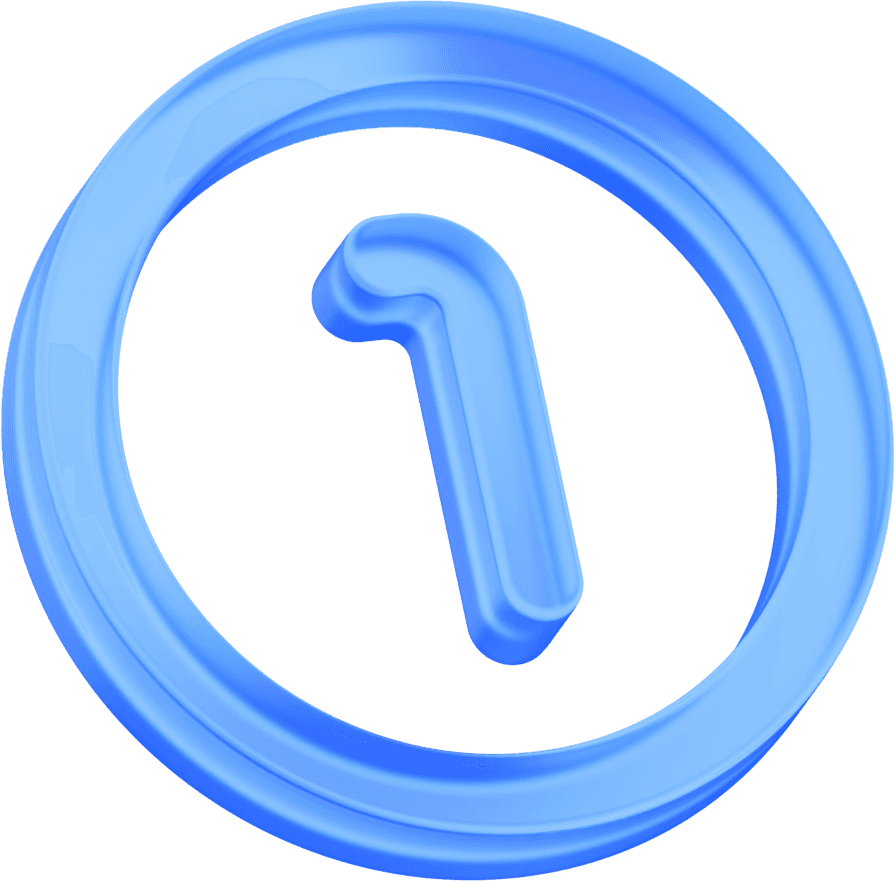
Filter out
unsuitable problems
We exclude easy problems, those requiring extensive calculations, and multiple-choice problems
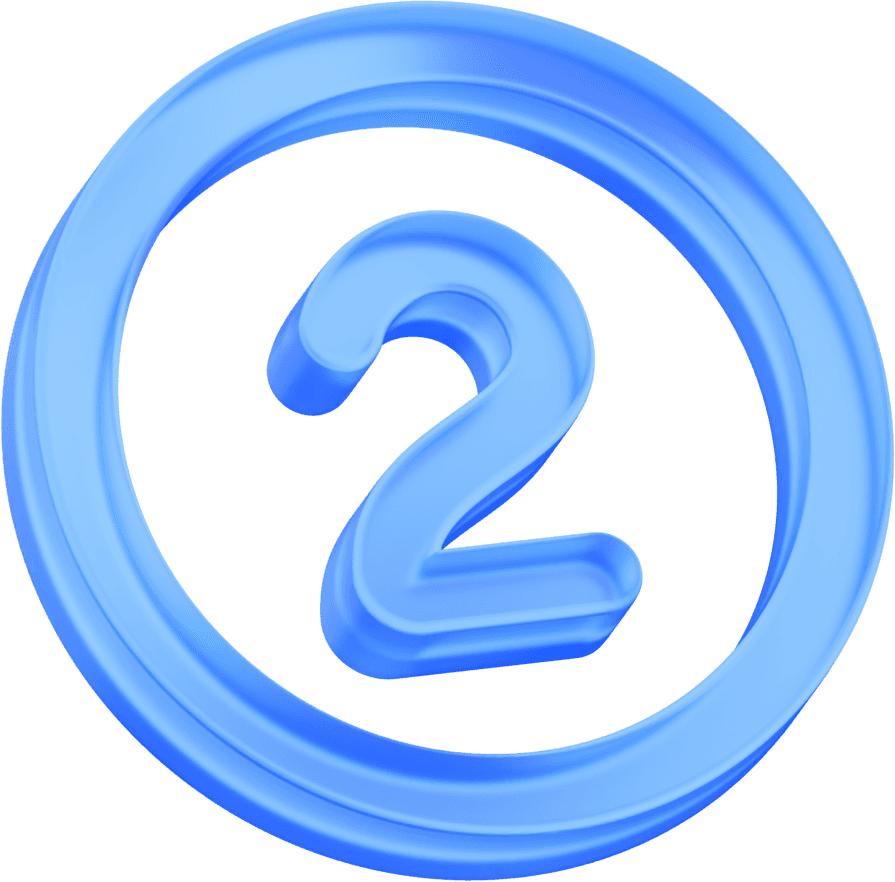
Test LLMs
Solve the selected problems using a number of popular SLMs
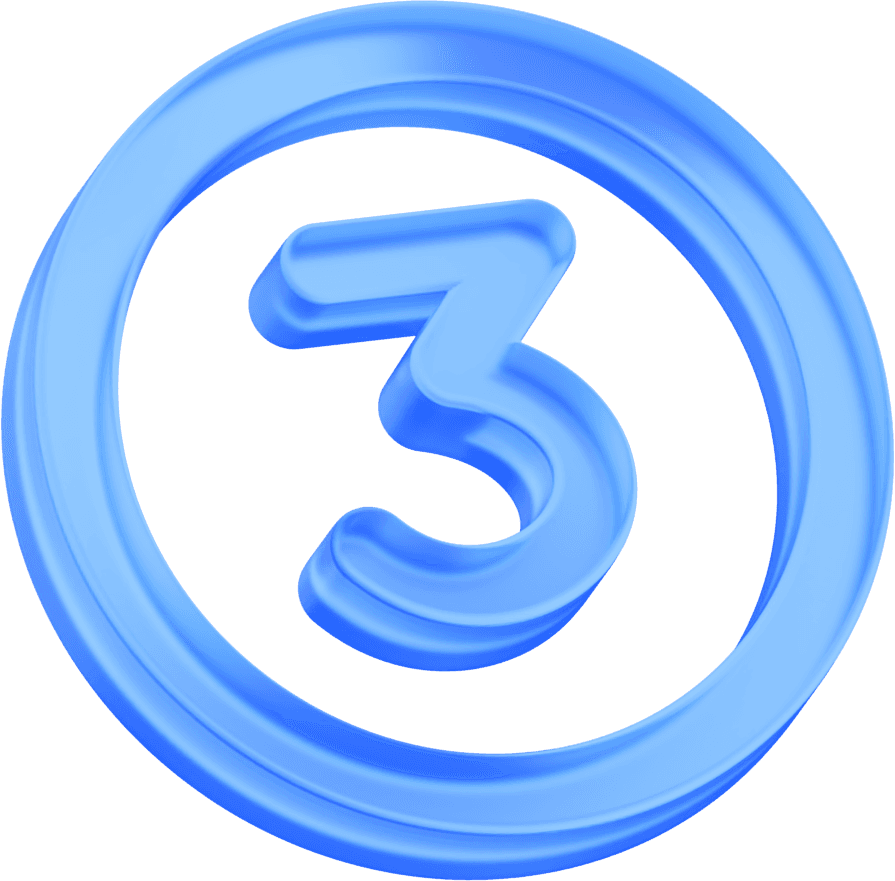
Analyze results
Choose the most challenging problems in each subject area
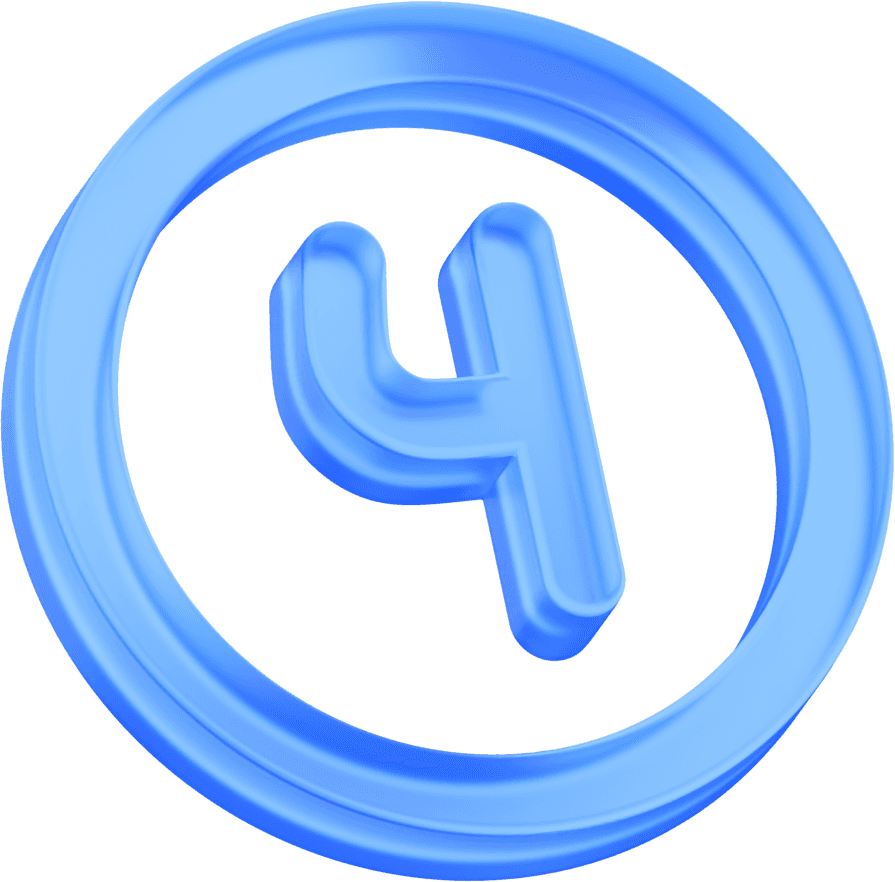
Expert validation
The final set of problems is verified by domain experts from Stevens Institute of Technology
U-Math Data Samples
μ-MATH Meta-Evaluation Benchmark insights
Problem-solving performance ≠ judgment performance. A tradeoff between these skills emerges in non-reasoners.
The tradeoff yields distinctive judgment styles: proprietary models are more conservative, minimizing false positives, while Qwens tend to be more lenient.
Reasoning models push through the Pareto frontier, typically inducing substantial imbalances. o1 pushes even further and exhibits a far more weighted performance.
Judging is a non-trivial skill. The maximum attainable performance is imperfect, even with reasoners, so we need to account for errors when using auto-evaluation.
A balanced mix of training data leads to well-rounded performance, as evidenced by Qwen2.5 and Qwen2.5-Math.
Reducing the model size, on the contrary, exacerbates bias. Balanced performance requires a model capable enough to properly generalize over the training mixture.
Which LLMs excel at judging math solutions?
U-MATH shows accuracy in solving math problems. μ-MATH reflects accuracy in judging solutions
μ-MATH Dataset Collection Process
Robust test for LLM judges
Judgment errors and biases in evaluations are often overlooked, creating uncertainty and unreliability. Meta-evaluations are essential to identify, quantify, and address these issues, yet they remain scarce, especially in math.
Dataset construction
— Hand-picked ~25% of U-MATH problems (271 in total), selected for their asessment complexity and overall representativeness of university-level math problems
— Generated 4 solutions for each: using Qwen2.5 72B, Llama-3.1 70B, GPT4-o and Gemini 1.5 Pro, resulting in 1084 problem-solution pairs
— Supplied each pair with a correct judgment verdict via a combination of Toloka's math experts labeling and Gradarius formal autoverification API
Testing and metrics
During testing, a model is provided with a problem statement, a reference answer, and a solution to evaluate, and tasked to determine whether the solution is correct or not. We treat this as a binary classification task
.
Primary metric:
Macro-averaged F1 score, so as to minimize the effect of class imbalance
Fine-grained metrics:
Positive Predictive Value (PPV, or Precision) and True Positive Rate (TPR, or Recall) for the positive class
Negative Predictive Value (NPV) and True Negative Rate (TNR) for the negative class
Data Sample
A sample problem from the µ-MATH meta-evaluation benchmark, illustrating a comparison between the golden (reference) answer and an answer generated by an LLM.
Frequently Asked Questions
Toloka’s U-MATH dataset is tailored for working with advanced mathematical problems. It provides structured data to simplify problem-solving processes and analyze complex mathematical ideas, supporting a wide range of applications in academic research, education, and AI training with an emphasis on measuring mathematical problem-solving skills for both models and students.
Here are answers to some common questions about the math dataset concept and Toloka's U-math dataset.