Training Persona-driven Chatbots with Toloka
Despite the continuing advancements in AI, having a consistent, lifelike conversation with a chatbot is still the exception rather than the rule. Most chatbots aren't that good at actually chatting and making small talk, but crowdsourcing platforms like Toloka can help fix that. Here's how.
About DeepHack
Among those trying to solve this chatbot conundrum were the contestants of the DeepHack hackathon. DeepHack took place as part of ConvAI2 – an international chatbot competition, whose mission is to develop a global standard for testing and evaluating dialog systems.
DeepHack's participants had to create a chitchat bot with an assigned persona. Each team was given a list of personality traits that could be used as topics for conversation, like "I enjoy jogging" or "Ramen is my favorite type of noodle".
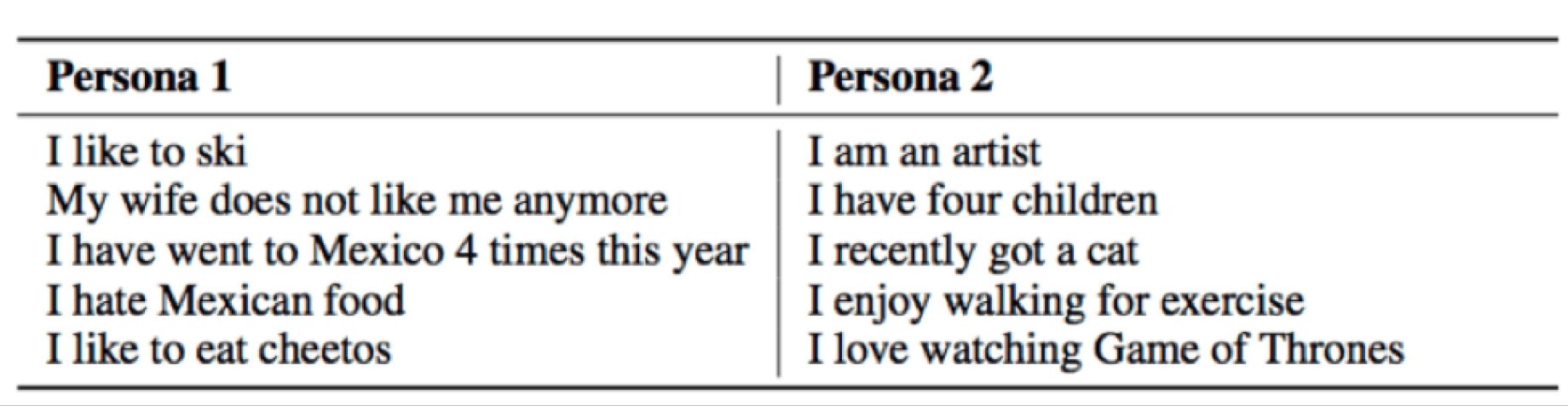
Personality traits
Two metrics were used to evaluate chatbot performance:
Overall quality – to assess whether the bot was making sense and could maintain an engaging conversation.
Role-playing – to judge whether the bot "behaved" in line with its assigned persona.
Making chatbots smarter with Toloka
So, how does Toloka fit into all this personality-driven bot business? Tolokers were the ones who actually engaged in small talk with the conversational agents and rated every response. Each toloker and each chatbot got a personality profile that they had to maintain throughout the dialog. Pretending to be their assigned persona, they had to tell each other about themselves and try to find out more about their peer. Neither saw the other one's profile.
Since all the chatbots at the event were English-speaking, the task was available only to users who had passed the English proficiency test. The dialogs couldn't be held in Toloka and instead took place in Telegram messenger.
Once the conversation was wrapped up, the dialog's ID and rating were put into Toloka as a response. The next step was to make sure the conversations were actually valid. To filter out dishonest users, another task was added to Toloka, where a new group of tolokers would read the dialogs and assess the quality of each conversation with the bot.
Promising results
A typical day at the hackathon went like this:
The teams upload their bots.
Toloka performers test them and rate the quality of the conversation.
The developers adjust their bot's behavior based on that information.
In just four days, the dialog systems got much better at talking to real people. On day one, most of the bots tended to respond with non-sequiturs or repeated the same phrase over and over again. By day four, their answers became more consistent and detailed. They even started asking questions of their own. And if that's not a trait of good conversation, what is?
Here's a dialog from day one:
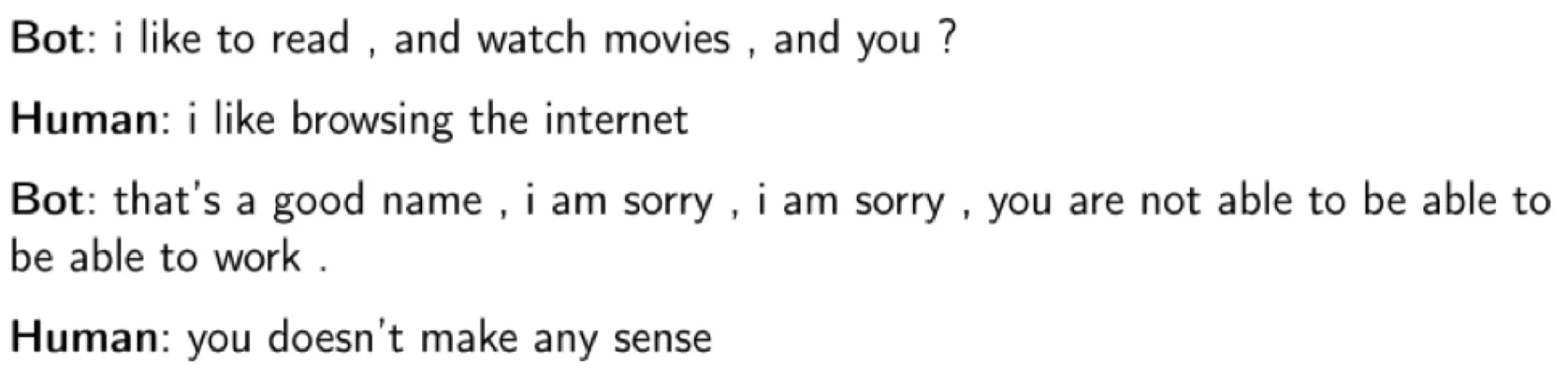
Dialog from day one
And here's one from day four:

Dialog from day four
Dialog evaluation lasted for four days, during which 200 tolokers rated 1800 dialogs. Toloka ultimately provided an effective pipeline for collecting chat data and rating bot quality with more reliable results than could be obtained using volunteers.