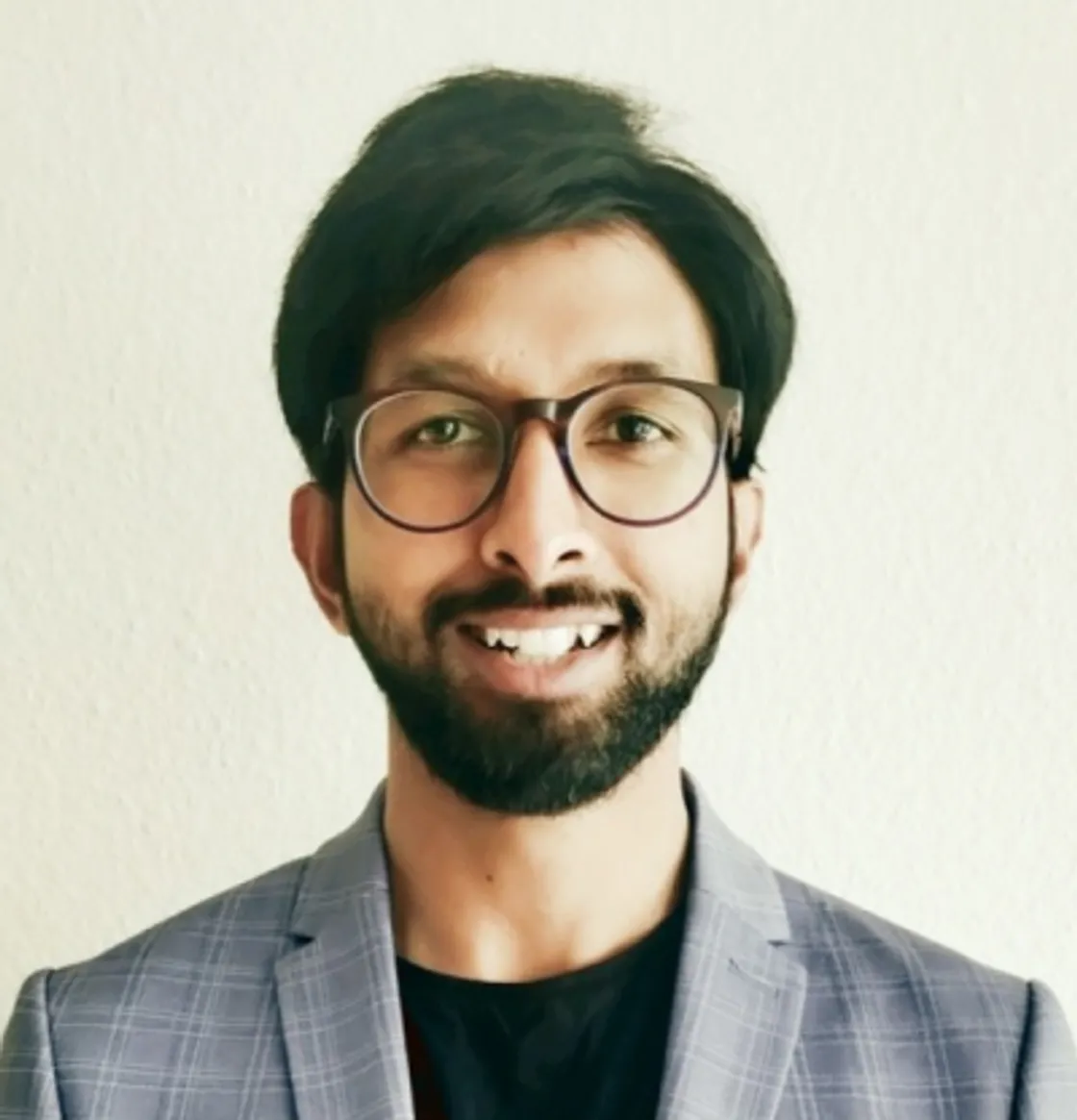
Ujwal Gadiraju
Claim Check-worthiness in Podcasts
It was only over a decade ago that the virtues of “Big Data’’ echoed in hallways and homes alike. The proliferation of mobile devices, concomitant technologies, social media platforms with user-generated content, and the world becoming more connected than ever before — have collectively led to a deluge of information (in terms of volume, velocity, and variety). We’re now in a world that is gripped by the extreme narratives surrounding AI boons and banes, but in a reality that lies somewhere in between. A consequence of advances in generative AI and the democratization of large language models is the growing ease with which more information can be generated and consumed. This has critical implications for the propagation of misinformation with potentially damaging ramifications.
Claim Check-worthiness
To ensure the veracity of information that is created, generated, propagated, and/or consumed by users, automatic fact-checking systems have been developed over the last few years. Claim check-worthiness is an important component of such systems to reduce costs and optimize the use of resources. For instance, it would be computationally expensive to fact-check every single claim in a sea of information on one hand, and it would be rather rudimentary to squander the time of expert fact-checkers to serve this purpose on the other hand. As a result, understanding which claims are worth checking is the first step that is necessary, and this is a task that has gained prominence in recent years. Researchers and practitioners in the natural language processing (NLP) and machine learning communities continue to build systems capable of automatically detecting claims that are checkable and check-worthy, and warrant further inspection for their factual accuracy [1–3]. A vital ingredient in this process is the availability of labeled data in the context of interest — be it news articles, social media posts, or discussions on forums. Crowdsourcing has emerged as a scalable means to acquire labeled data by leveraging human input through existing marketplaces such as Toloka AI.
Fact-checking Podcasts — A New Frontier
Ina recent collaboration between Toloka AI and Factiverse, a Norwegian company in the fact-checking business, we explored the claim check-worthiness task in the unique context of podcasts. Podcasts are becoming increasingly popular and are estimated to become a 4 billion dollar industry by 2024 [4]. Considering the widespread popularity of podcasting, the growth in platforms, and the increasing amount of content available on a multitude of topics, fact-checking podcast content is extremely important. Limiting the propagation of misinformation via podcasts, safeguarding listeners, and empowering them with the opportunity to make informed decisions by providing factually accurate information is a valuable goal to strive for in this day and age.
Claim check-worthiness of Podcasts Data on Toloka
There are a number of intriguing questions surrounding how we can augment information pertaining to the factual accuracy of claims made in podcasts without hampering the listening experience of users. However, the first step remains the effective identification of check-worthy claims. To this end, we considered a random subset of podcasts from the genres of “News & Politics” or “Health & Wellness.”
Toloka AI provides a useful set of tools to design and deploy annotation tasks on the platform. It supports the recruiting of crowd workers (referred to as ‘Tolokers’) from various parts of the world. For the scope of this project, we built a custom annotation interface that Tolokers were directed to use to complete the claim check-worthiness tasks (see Figure 1).
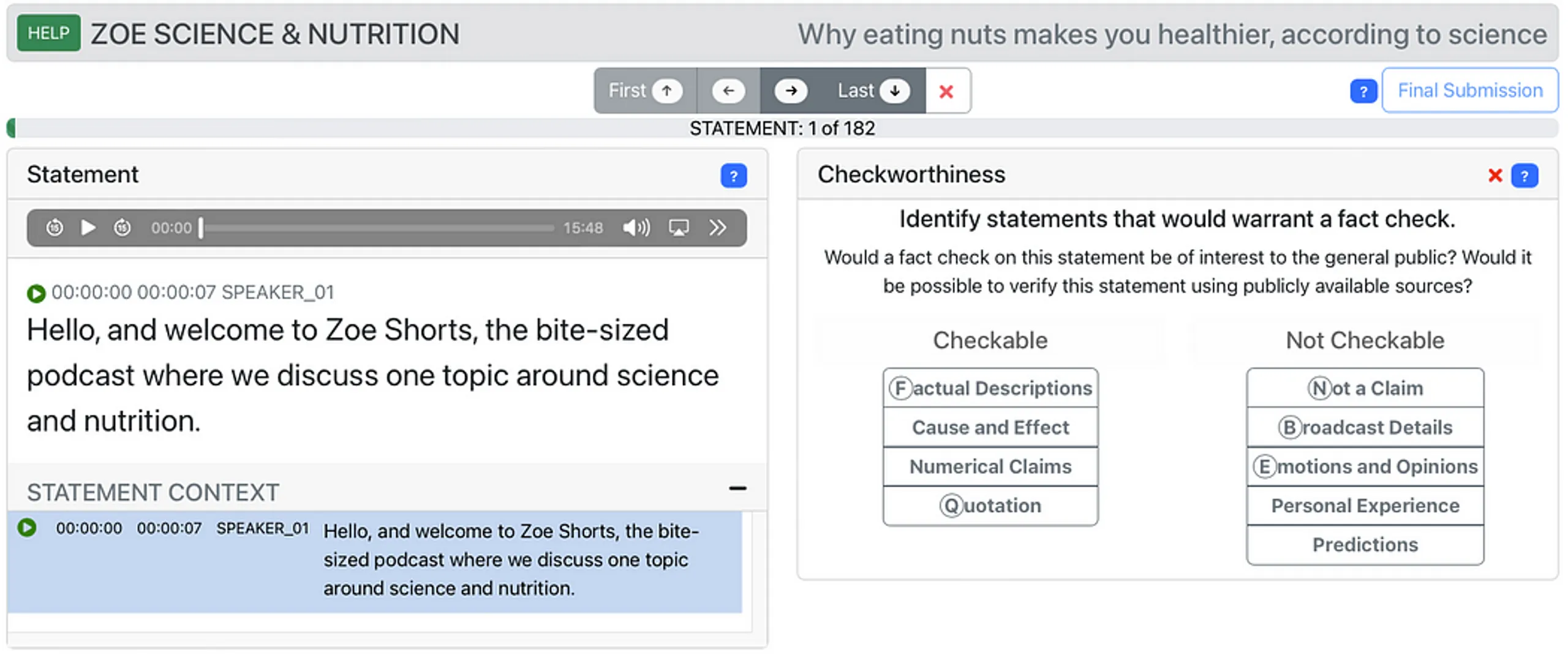
Figure 1: The annotation interface used to assess the check-worthiness of statements in the podcasts considered in this pilot project. Source: Screenshot image by authors.
On starting the task and after reading through the instructions, Tolokers are presented with the annotation interface. A panel on the left-hand side of the screen presents the “statement” that needs to be assessed for checkworthiness. Tolokers can listen to the audio snippet of the statement from the podcast in addition to reading it if they wish to do so. At the bottom of the panel, Tolokers can scroll through the preceding context (when it exists) to help obtain more context and make an informed assessment when in doubt. The right-hand side of the screen presents the checkworthiness assessment panel.
“Checkable” versus “Not Checkable” Statements
The first decision that a worker has to make is whether or not the statement is verifiable using publicly available sources (i.e., determine whether the statement is checkable or not checkable). Following this decision, the worker needs to provide a rationale for their assessment of check-worthiness. Checkable statements follow one of the four following characterizations:
Factual Descriptions: Claims about the existence or characteristics of notable people, places, things, events, or actions, which are possible to verify with public sources.
Examples: “She won the London Marathon last year.”
“Rival groups were involved in a gunfight on the outskirts of the city.”
“The money laundering scheme violated both national and international law.”
“Italians drink beer with their pizza, not wine.”
“The budget deficit is the overall amount the government currently owes to its creditors.”
“Photosynthesis is the process in which plants and some other organisms use sunlight to synthesize foods.”
Cause and Effect: *Claims asserting one thing is caused by or linked with another, which can be checked against reputable sources.
Examples: “The company collapsed after a rogue employee was discovered to be embezzling funds.”
“Smoking causes cancer.”
“Obama only got into Harvard because his parents are rich.”
“The new law has led to a rise in crime.”
Numerical Claims: Claims which involve specific statistics or would require counting or analysis of numerical data to verify.
Examples: “The average Mexican consumes more sugar per day than the average American.”
“The latest poll shows that 80% of people are unhappy with the current government.”
“The drug was found to improve the symptoms of 57% of patients.”
“There are 14 hospitals in the southern region, two more than a decade ago.”
Quotation: Repeating the words of another notable person or entity which can be verified in public sources.
Examples: “The mayor was clear when he said, ‘All flooded households will receive emergency assistance after a damage assessment.’”
“President Roosevelt famously said, ‘Ich bin ein Berliner.’”
“The company’s CEO announced they are committed to reducing their carbon footprint 50% by 2030.”
Statements that are deemed to be “Not Checkable” follow one of the following five characterizations:
Not a Claim: Not making any sort of claim, including questions not including some factual assertion.
Examples: “Hello, how are you?”
“How old are you?”
“Thanks for chatting with us today.”
“I’m sorry, I didn’t know.”
“Let’s get into detail.”
“Stop doing that.”
Broadcast Details: Introducing the speakers, describing the program, or giving details related to the episode contents.
Examples: “Welcome to the show, I’m your host, John Smith.”
“Today we’re going to be talking about the history of the internet.”
“This is episode 3 of our series on the history of the internet.”
“Our guest, Dr. Jane Doe, is joining me in the studio to share her expertise.”
Emotions and Opinions: An emotion that is being felt or expressed, or an opinion that doesn’t contain a checkable factual assertion.
Examples: “I love how the tulips look early on a spring morning.”
“He’s really upset about the way things are going at school.”
“These politicians are the only ones who have half a clue.”
“I’m so excited to see you, it’s been too long!”
“Everyone around here loves that restaurant.”
Personal Experience: Claims a person makes about their own experience, but which cannot be verified in public sources.
Examples: “I passed four empty buses on my way to work yesterday.”
“My grandmother used lard in her pie crusts.”
“I’ve never seen a bluebird in this part of the country.”
“My daughter caught 3 huge trout in that stream last summer.”
Predictions: Claims and predictions about future events or plans that can’t be confirmed at present.
Examples: “Elon Musk will visit Mars.”
“The sun will rise tomorrow.”
“New car sales will increase every month going forward.”
“The company will be profitable by the end of the year.”
“We’ll all be dead in 100 years.”
The distinctions between ‘Checkable’ and ‘Not Checkable’ statements stem from the practices employed by professional fact-checkers. In this endeavor, we collaborated closely with fact-checkers from Faktisk.no, who regard this classification as the initial step in selecting claims for fact-checking. Faktisk.no AS is a non-profit organization and independent newsroom for fact-checking the social debate and public discourse in Norway. The purpose behind this approach is to effectively sift through sentences and identify those that may not qualify as claims or present difficulties in verification, such as opinions and predictions made by the speakers. By doing so, we ensure that the fact-checking process focuses on statements that can be objectively validated by providing evidence.
When Tolokers determine that a particular statement is ‘Checkable’, they are asked to (a). highlight the part of the podcast statement that is worth fact-checking on the panel on the left-hand side, and then a new panel appears underneath the assessment panel on the right-hand side, asking the worker to (b). explain the motivation behind why one would want to fact-check the statement by choosing one of the following five options:
Potential to Cause Harm: I think this statement could cause harm if false.
Said by a Prominent Person: I want to check if this prominent person actually said this.
Public Interest: I believe the fact-checking of this claim is for the public interest.
Surprising: I find this statement surprising, shocking, or otherwise hard to believe.
Learn More: I would gain new knowledge about this topic by fact-checking this statement.
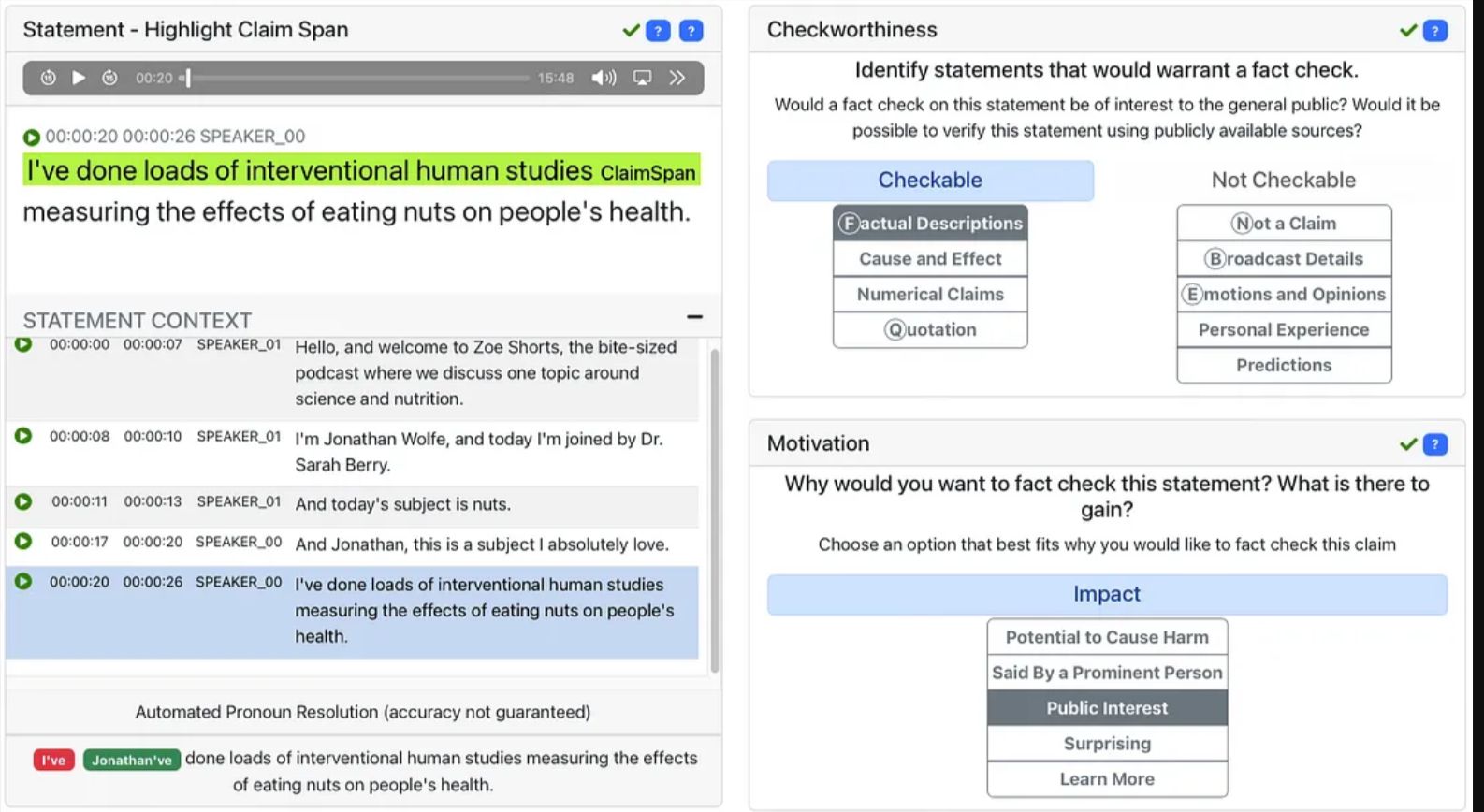
Figure 2: Highlighting the span of the statement worth fact checking (top-left), specifying the motivation behind the fact checking of the highlighted claim (bottom-right). Source: Screenshot image by authors.
Why is this Useful Anyway?
In total, we gathered 1,766 annotations from 33 unique Tolokers. We found that the Tolokers annotated approximately 3 statements per minute. The annotations spanned 10 different podcasts, of which 805 statements were found to be checkable and 961 statements were found to be not checkable.
These annotations play a crucial role in Factiverse’s efforts to enhance and refine their check-worthy claim detection models in their fact-checking pipeline. Additionally, Factiverse serves numerous media customers with podcasting platforms in the Nordics, and these annotations are instrumental in training models to identify check-worthy claims within podcasts. The ultimate goal is to quickly identify podcasts that might contain potentially inaccurate information, along with the claims that are inaccurate and the supporting rationales. This will also solve challenges that fact-checkers such as Faktisk.no face when having to fact-check lengthy podcasts.
Given that these annotations are fine-grained, including the rationale for considering a claim as check-worthy, they also serve as valuable resources for understanding the model behavior from an explainability perspective. Through the creation of diagnostic datasets, Factiverse can gain insights into the performance of its production models. For instance, they can determine if fact-checking models for numerical claims exhibit any underperformance, enabling Factiverse to take necessary actions to enhance the models’ robustness effectively.
This article was written with an equal contribution from the CTO of Factiverse, Vinay Setty, and input from other members of the Factiverse and Toloka AI.
References
Guo, Zhijiang, Michael Schlichtkrull, and Andreas Vlachos. “A survey on automated fact-checking.” Transactions of the Association for Computational Linguistics 10 (2022): 178–206.
Wright D, Augenstein I. Claim check-worthiness detection as positive unlabelled learning. arXiv preprint arXiv:2003.02736. 2020 Mar 5.
Opdahl, Andreas L., Bjørnar Tessem, Duc-Tien Dang-Nguyen, Enrico Motta, Vinay Setty, Eivind Throndsen, Are Tverberg, and Christoph Trattner. “Trustworthy journalism through AI.” Data & Knowledge Engineering 146 (2023): 102182.
Article written by:
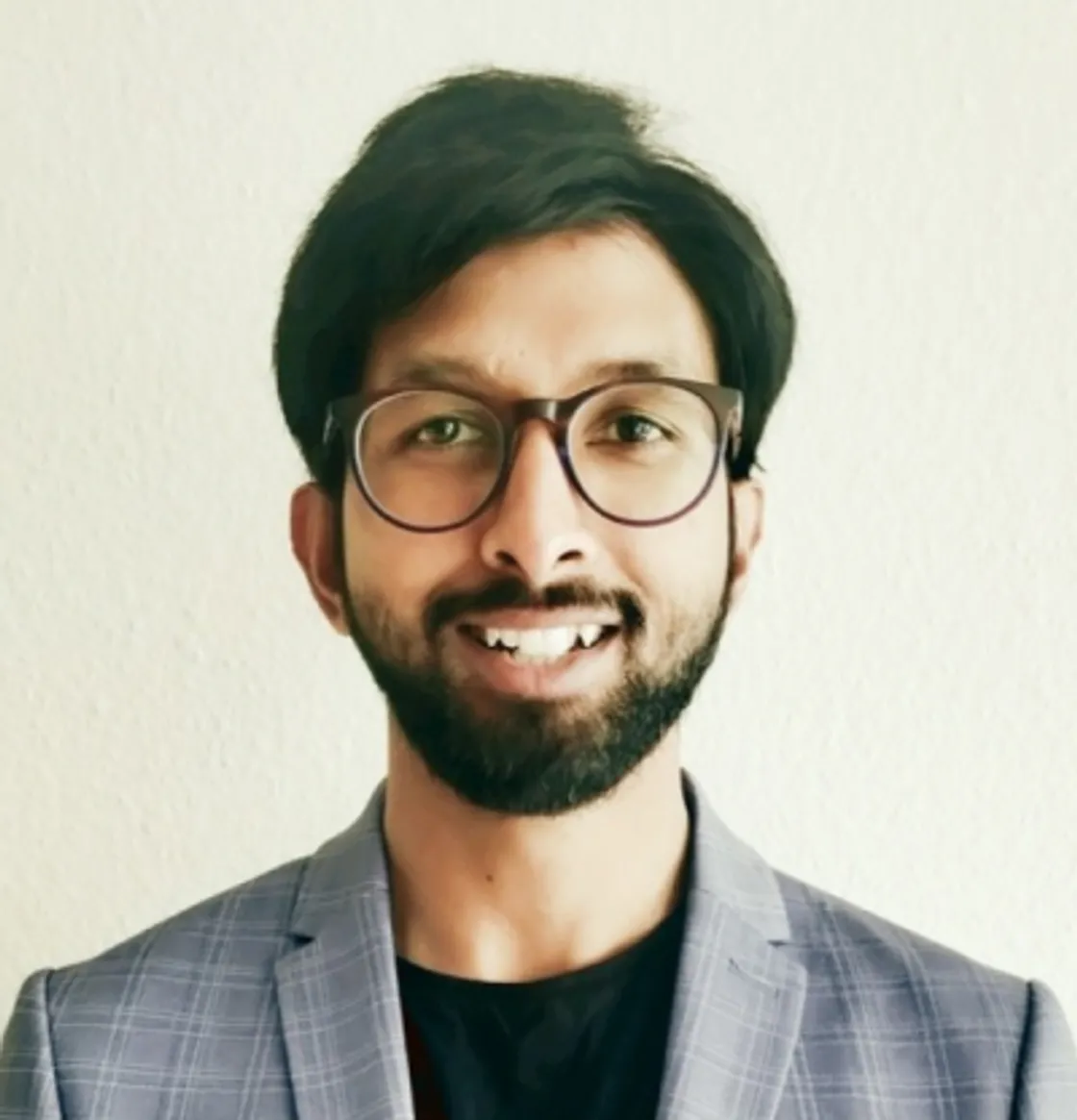
Ujwal Gadiraju
Updated:
Sep 8, 2023