Fact-checking LLM-generated content
Introduction
With the explosion of Large Language Models (LLMs) onto the scene, many people have been wondering how accurate this new technology is — and will it outpace us humans? For now, at least, professionals across industries can breathe a sigh of relief since these AI-based automated tools still have quite a way to go in terms of the accuracy and reliability of their responses.
One of the main challenges of working with LLMs is that the content they generate isn’t always trustworthy and needs to be verified. Even well-known systems like ChatGPT aren’t always correct and must be fact-checked. That’s where human input comes into play. Humans play a vital role in authenticating content generated by LLMs and making sure that it is accurate, reliable, and safe for consumption. In this article we’ll dive deeper into everything you need to know about fact-checking LLM-generated content, including why it’s important to fact-check content, how to verify accuracy, what to pay attention to, and more.

Why it’s important to fact-check AI-generated content
While increasing numbers of people are using artificial intelligence tools to generate content, whether in their jobs, at school, or for personal use, there’s a glaring problem that arises: the issue is that AI-based tools comb the Internet for all kinds of sources of information (many of which are unreliable or even harmful) to create content. If you fail to verify that content, you could open yourself up to all sorts of risks. To protect your business, your network, and your reputation, you need to be sure to fact-check the content that an AI-based LLM has generated.
Moreover, artificial intelligence is only as good as its sources of information, so if the training data isn’t correct, the model isn’t going to be able to confirm the accuracy of the data it’s processing. The model can also unwittingly take on the judgments and biases found in that source of information. That’s why oftentimes the text generated by these LLMs isn’t correct — and in some cases, completely made up. See Hallucinations below.
Besides, LLMs don’t have any idea of what the language being used is describing in real life. A lot of what we know as humans isn’t recorded either online or in written form. Instead, it’s been passed on to us through our cultures, traditions, and beliefs. So, while artificial intelligence systems that generate text may sound perfectly fine, grammatically speaking, they don’t have any other objective other than to adhere to the statistical consistency of the prompt they’re given. In other words, they don’t have the background context that we as humans do, and need to be fact-checked for accuracy, reliability, and safety.
Limitations of large language model-generated content
Artificial intelligence content refers to content generated by LLMs such as ChatGPT and Google Bard that create content founded on human-based input like keywords, queries, or prompts. LLM-generated content is a lot faster to create, which makes it a great tool for scaling content production at large. But there are some significant drawbacks:
Inaccurate or misleading content
While AI content can be grammatically correct, it can also be semantically incorrect or misleading, at the same time. This is an issue in high-risk fields such as law or medicine.
Introduction of biases
LLMs can introduce bias and prejudices into their text from harmful sources that they’ve inadvertently mined through the Internet.
Lack of creativity
Generative AI isn’t fully capable of delivering the voice, tone, and personality to fit specific audiences.
AI hallucinations
An AI writer, chatbot, or tool can sometimes generate unexpected, strange, or fictitious content. Without possessing logic or reasoning abilities, the tool can spout contradictions or inconsistencies, known as “hallucinations”.
Moreover, hallucinations can be a significant issue for LLMs because they can lead to the spread of misinformation and fake news, expose confidential data, and create unrealistic expectations about LLM capabilities. Even worse, they tend to broadcast nonsense confidently.
As you can see, it’s important to recognize the inaccuracies and biases present in LLM-generated content. That’s why human oversight and fact-checking is crucial.
What to look for in the fact-checking process
It’s important for human annotators to follow the steps outlined below when evaluating an LLM’s output and reviewing other AI fact-based content:
1. Identify the author of the source material — Check for bylines as most credible authors will clearly indicate that they wrote the material. However, it’s worth noting that bylines can also be fabricated, so this isn’t a foolproof solution by itself.
2. Verify the website or author’s credentials — Reliable sources list their credentials in a visible way, so you don’t have to guess as to whether or not you're using credible sources. To investigate further, you can cross-reference social media posts and accounts, About us pages, and more.
3. Return to the original context — It’s super easy to take things out of context. Think clickbait, sensational or fake news. Go to the original source and consider the context in which something was written.
4. Determine the purpose of the information — Why has the information been published? Shared content isn’t always altruistic; it can be shared for the wrong reasons. Do some investigating into why the source material was created in the first place.
5. Consider the underlying biases — Is the source material prejudiced against a certain group or does it introduce false narratives or spread fake news articles? Take steps to limit any non-objective information.
6. Check if the material is current — Look at how far back the source material dates. Older publications may not be as applicable since new information gets published all the time. Try to avoid sources that are older than three to five years.
7. See if others have referenced the material — Generally speaking, the more individuals who have cited the source, the more reliable the claim is. But be sure to use your own judgment on this. Not everything the majority cites is true.
Fact-checker AI as a tool
AI isn’t a reliable fact-checking tool — at least not yet anyway. It doesn’t have the knowledge that humans have and isn’t always able to discern context or keep perspective. Basically, it tells you what you want to hear and not what you need to know. However, researchers today are aiming to enhance AI’s fact-checking capabilities to counter widespread misinformation and fake news in our increasingly digitalized world.
Let’s take a look at the case of ChatGPT as an example.
ChatGPT case study
An LLM system that produces a series of probabilities to predict the next word in a sentence, ChatGPT absorbs an enormous amount of data to comprehend language within a given context and then replicate it in new and innovative ways for the benefit of its users.
A team of fact-checkers and reporters from PolitiFact at the Poynter Institute decided to conduct an experiment to see how often ChatGPT was correct. Out of 40 different tests performed, it faltered in about half of them; it either made an error, failed to provide a response, or arrived at a different conclusion than the team. Even though it wasn’t entirely incorrect in those instances, the minute differences resulted in larger inaccuracies and discrepancies, which lead the team to believe that ChatGPT isn’t a reliable source of news information.
Moreover, the team concluded that this innovative AI tool is subject to several limitations, including:
Dated knowledge and a lack of real-time information — The free version can’t access data after September 2021. Most people are interested in current information, so this presents a significant drawback. However, other chatbots can scour the web for the most up-to-date sources — which is the course that future LLMs will likely take anyway.
Inconsistency — One query may elicit a helpful and accurate response, while another similarly phrased one will not. It all depends on how a question is posed and the order in which it is asked. Even the same question on the same topic asked twice could elicit different responses. The way in which the prompt is worded is everything. Not only that, but the system also oscillates between being overly confident and unsure.
Lack of nuance — Basically, ChatGPT takes everything literally and can’t understand the subtleties and nuances of certain queries. Even though it can sound authoritative, it often fails to understand the underlying context around a particular topic, such as how theories about mRNA codes on streetlights relate to COVID vaccine anxieties or whether George Washington ordered smallpox inoculations among his Continental Army soldiers.
Incorrect answers — Sometimes, it would get things totally wrong. Whether collecting the right scientific data for a particular experiment, yet performing incorrect calculations, or making up nonexistent sources, this AI tool simply cannot always provide the right answer. In other words, it’s unaware of when it doesn’t know something.
Here is a list of all of ChatGPT’s responses to the team’s.
It’s particularly hard to get any AI tool — not just ChatGPT— to recognize the truth or be 100% accurate in its responses. There’s still a lot of work to be done for generative AI to be considered a reliable fact-checker. So, while human fact-checkers can rest assured that their careers aren’t yet at stake, it’ll be the ones who know how to use these AI tools to their advantage that excel — just like many other careers. Those who can assess the accuracy of the model’s output are the ones who will have the easiest time with this new technology. In other words, if you already know the answer, you can rely on these tools — not very helpful for the moment! The danger lies in the public relying on these tools for answers.
Current methods for detecting LLM-generated text
Modern enhancements in Natural Language Generation (NLG) technology have dramatically improved the quality of LLM-generated texts. It’s almost impossible to tell the difference between text written by humans and text generated by an LLM — although the latter may actually be better! You can imagine the havoc this could cause in terms of the spreading of false information, fake news, phishing, social media and social engineering scams, and even cheating on exams. To build public trust in these systems, we have to find ways to identify LLM-generated text and factually verify the content.
Current detection methods for LLM-generated content can be grouped into two categories: black-box detection and white-box detection.
Black-box detection — confined to API-level access; these methods depend on text samples collected from humans and machines to train a classification model to differentiate human versus machine-generated text.
How does it do this? LLM-generated texts tend to have certain linguistic or numerical patterns that differentiate them from those written by humans. Unfortunately, black-box methods have the potential to become somewhat obsolete as LLMs evolve.
Examples: data collection, feature selection, and classification model design.
White-box detection — Typically carried out by an LLM developer or a senior data scientist, the detector has complete access and control over the LLM and can trace the model’s generative patterns.
Examples: post-hoc watermarks and inference time watermarks.
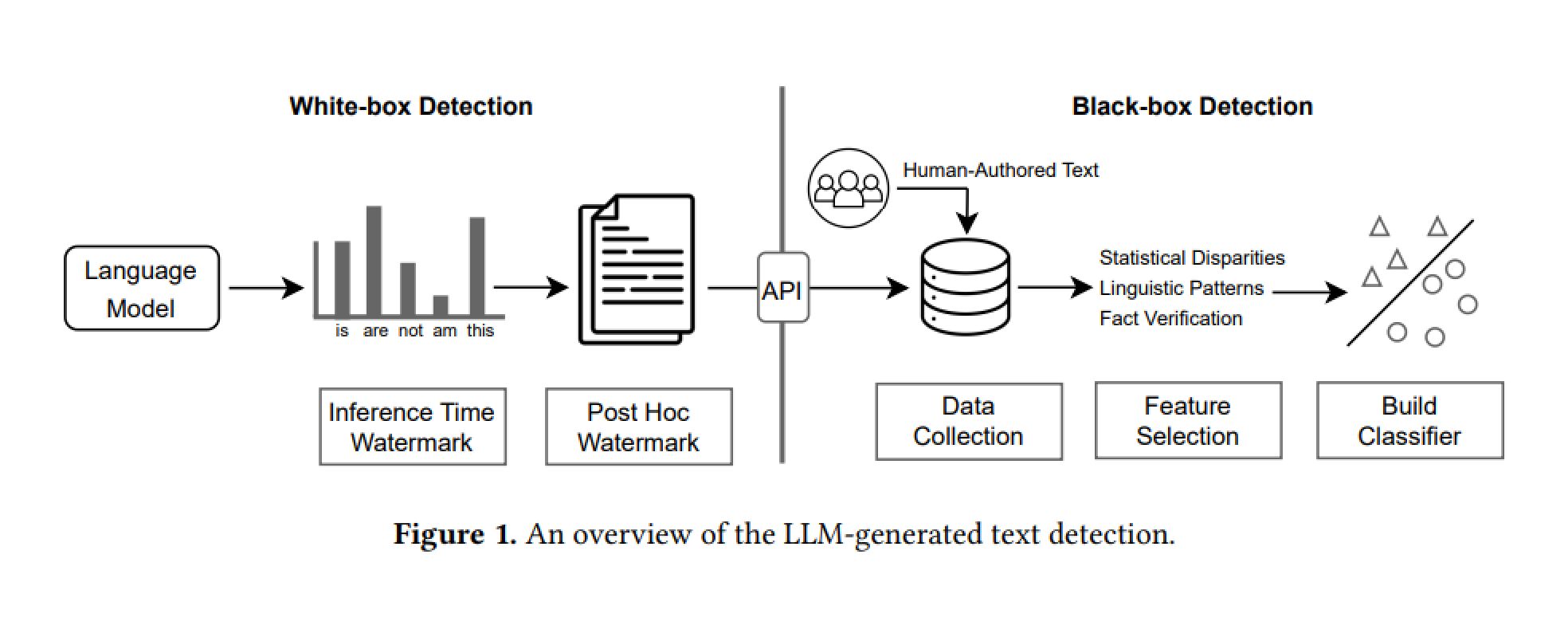
Best practices for fact-checking AI-generated content
We’ve outlined seven steps you can take to verify the content generated by an LLM:
Cross-reference information — Use multiple sources to verify the content produced by an LLM; in particular, cross-referencing numbers, results, claims, dates, names, media outlets, and more. Use a search engine like Google Search to perform a simple search of these facts to see if they add up.
Employ fact checkers — They can help verify facts and figures, which saves you time and energy. Consider fact-checking tools like Bing Chat, Google Fact Check Explorer, Claimbuster, and more. Don't just trust one source or news article.
Verify the source’s credibility — Make sure the content is coming from a reliable source, and don’t buy into skewed statistics that promote a company’s products or interests. Avoid referencing dubious studies and check author bylines.
Evaluate believability and context — Use your best judgment to determine the plausibility of statements or claims made. Are they sensational or controversial? That’s a red flag! Consider context and cross-reference expert opinions. And remember to always question your sources.
Check the date — The original date of publication is another good indicator of the content’s credibility. Always try to find more recent sources to avoid using outdated sources, which may not be as relevant or factually correct anymore.
List original sources — Especially when referring to anything that isn’t commonly known. This way, you can avoid any misunderstandings or lost-in-translation incidents.
Separate facts from opinions — Facts are facts, and opinions are just that — opinions. Be sure that each is reflected as such in the content. Otherwise, you could be spreading misinformation online or fake news. Remember to question, question, question!
Improving LLM-generated text with guardrails
Human input plays a key role in making large language models — and the text they generate — safer and more reliable. One example of the main ways in which human input is valuable is in the establishment and enforcement of guardrails.
In short, guardrails are defenses — policies, strategies, mechanisms — that are put in place to ensure the ethical and responsible application of AI-based technologies. By making sure that humans are involved in key decision-making processes, guardrails can help guarantee that AI technologies stay under our control and that their outputs follow our societal norms and values.
Here are some of the key benefits of guardrails:
Protect against the misuse of AI technologies
Promote equality and prevent bias
Preserve public trust in AI technologies
Comply with legal and regulatory standards
Facilitate human oversight in AI systems
When it comes to the importance of guardrails in AI systems, transparency and accountability go hand-in-hand. You need transparency to ensure that responses generated by these systems can be explained and mistakes can be found and fixed. Guardrails can also ensure that human input is taken into account in regard to important decisions in areas like medical care and self-driving cars.
Fundamentally, the point of guardrails is to make sure that AI technologies are being used for humanity's betterment and serve us in helpful and positive ways without posing significant risks, harm, or danger. When applied to the text generated by LLMs, they can be a saving grace!
Key takeaways
It’s critical to fact-check LLM-generated content for a number of reasons, namely, for safety precautions, elimination of biases, protecting personal and brand reputation, and mitigating AI hallucinations, among others. AI systems are known to “hallucinate” or fabricate content given their implementation of natural language processing models to predict what comes next in a sequence of text.
While LLM-generated content can be a helpful tool for many professionals, especially those involved in writing, it’s only as good as the information it collects. Given that our digital world is full of inaccurate, biased, and otherwise harmful sources, you need to take precautions when leveraging AI-based text. Ensure that the information you’re using is from reliable sources, accurate, and valuable to a wider audience.
Furthermore, be sure to always cross-reference and question your sources. Remember, AI models are known for confidently spouting opinions or inaccuracies as hard facts. Check for any red flags. Consider the following when fact-checking LLM-generated articles and text: the author and website’s credibility, the original source, the context, potential biases, the goal of the source, and how current the information is, among other factors.
We invite you to read through our blog to learn more about large language models and how Toloka can help in their fact-checking and evaluation.
About Toloka
Toloka is a European company based in Amsterdam, the Netherlands that provides data for Generative AI development. Toloka empowers businesses to build high quality, safe, and responsible AI. We are the trusted data partner for all stages of AI development from training to evaluation. Toloka has over a decade of experience supporting clients with its unique methodology and optimal combination of machine learning technology and human expertise, offering the highest quality and scalability in the market.
Article written by:
Updated:
Aug 4, 2023