
Multimodal AI: Collaborative Emotion Labeling in Audio Data
In recent years, Toloka has been developing datasets to help Large Language Models (LLMs) gain expertise in various domains and engage with users more effectively. The majority of the data has been texts for chatbot interfaces with a didactic or bureaucratic tone that feels like a conversation with a teacher or a consultant. Now we’re experimenting with something new: multimodal data that can make AI more empathetic and aware of nuanced human emotions.
The latest voice AI assistants sound surprisingly human. They’ve made huge strides in detecting and expressing emotions through subtle cues like voice inflection and pauses. For many people, it’s like a breath of fresh air after years of chatbot interfaces that feel dry and didactic.
We believe more AI teams want to pursue the emotional development of AI systems, but training data is limited. To reduce the data barrier, the Toloka Research team is excited to share our latest work in this area and contribute a dataset to the public domain.
Multimodal LLMs and personal assistants
Recent advancements in GenAI have made models multimodal, capable of processing and generating speech, images, and videos. These capabilities are currently being integrated into personal assistants like Siri, Alexa, and Google Assistant, as well as digital avatars such as Synthesia and applications like Character.AI and NPCs in gaming. They will significantly improve the realism, fluency, and context sensitivity of conversations, making interactions with assistants more natural and effective. However, there is still room for improvement. The next step in making these interactions even more realistic is Emotional AI, which will enable models to express, recognize, and respond to emotions.
Emotional AI
More multimodal models will reach customers in the coming years, but their success depends on achieving satisfactory performance, which is closely tied to the quality of their training data. This article focuses on the importance of audio annotation in this AI race. While much research has been devoted to creating reliable text-to-speech and speech-to-text systems, including work on accents and pronunciation, emotion analysis from speech is still under-researched. Emotions are a key component in human conversations, and recognizing and expressing them efficiently leads to trustful engagement in conversations. Teaching AI to understand and express emotions would take us another step closer to AGI (Artificial general intelligence).
Our recent work set out to cost-effectively annotate audio with emotions, providing data to train and improve relevant models further. Our additional goal was to source the annotation from diverse communities and understand if we could include communities with diverse cultural and linguistic backgrounds in speech annotation projects. The dataset we have created is valuable for training and evaluating both types of models, ones that recognize emotions, and generative models that produce speech with desired emotion characteristics. Additionally, the same methodology could be used for annotating more speech samples and amplifying and revising the range of emotions or speech characteristics found in the recordings.
Toloka’s audio annotation project
As a part of the project, we annotate a dataset of short recordings (a few seconds long) with different emotional characteristics. The annotation is divided into two parts. The first part focuses on annotating scalable voice characteristics such as pace (slow, normal, fast, variable), pitch (low, medium, high, variable), and volume (quiet, medium, loud, variable). The second part asks annotators to identify emotions and unique voice features by choosing appropriate adjectives from a large pool of 200+ words.
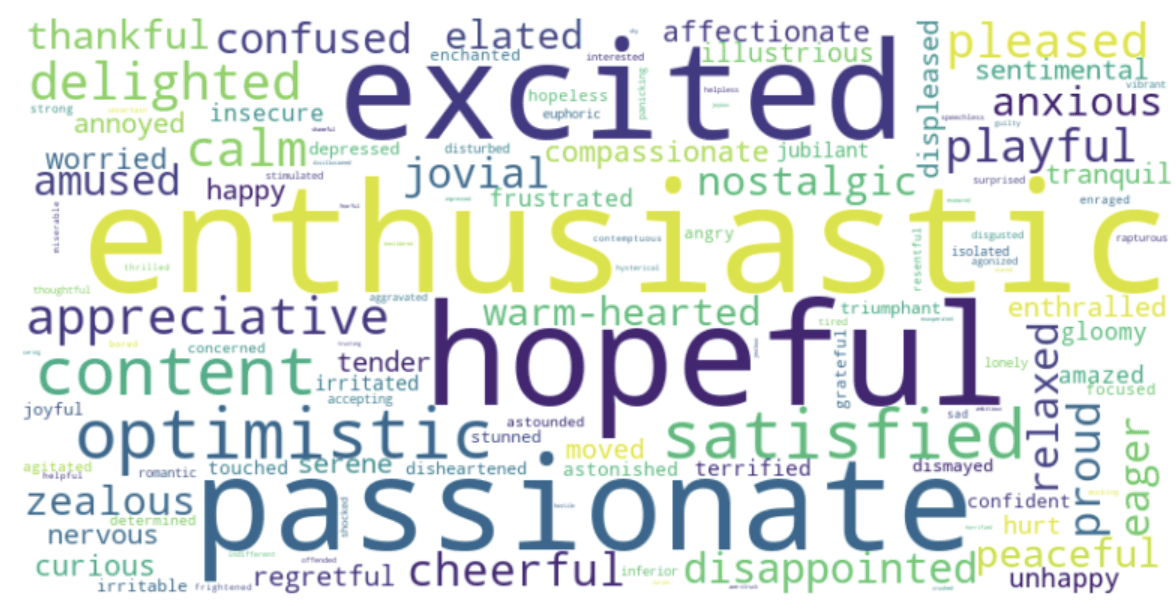
Word cloud representing the most frequent emotions present in the recordings
Here is a sample of a typical recording that we aimed to annotate. We can hear a woman talking with a happy, excited, optimistic voice.
During our project, we tried two different approaches: an in-house team of 19 experienced trained annotators, and a global crowd of 171 Tolokers with diverse cultural backgrounds. The advantage of the first one was potentially higher quality of annotations, whereas the second approach had the advantage of cultural diversity, better scalability and affordability.
Each data sample was annotated by three randomly assigned in-house experts and three annotators from the general crowd. All labelers had been previously trained on a variety of examples of correct annotations and demonstrated sufficient quality. To control the quality of the process, we used automatic post-acceptance checks with LLM (Mixtral 7x8). Its aim was to examine if there were any contradictions in emotional descriptions, do a logical majority vote over the emotional description of 3 labelers, and check the basic majority vote over pitch/volume/pace. Based on its verdict, some labels could have been rejected.
As a result, the example recording included above has been annotated by the majority of annotators as medium-pitch, medium-volume, and medium-pace. The annotators have also identified the following emotions and voice characteristics:
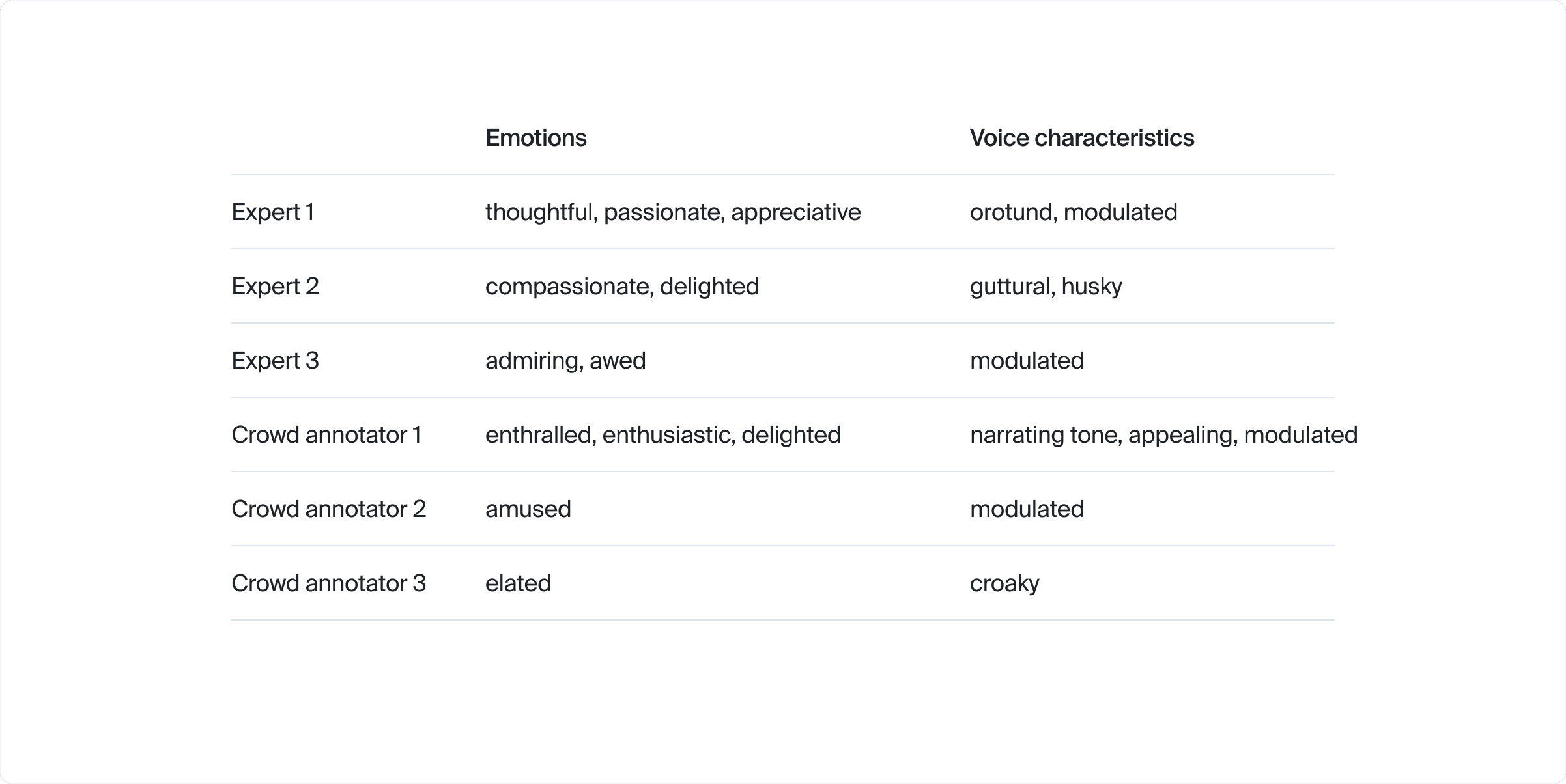
Conclusions
After assessing the responses and quality of both groups, we have drawn the following conclusions:
The global crowd was able to reliably annotate pace, pitch, volume (achieving inter-annotator agreement up to 86%), and even emotions at the same level as expert annotators. Voice characteristics were more difficult to reliably annotate for both groups and require further research.
Crowd and experts described our dataset similarly from semantic perspective, but experts’ vocabulary and words per annotation appeared to be on average 1.5-3x broader.
Crowd annotations were easier to source, three times less expensive, and almost two times faster than experts, having comparable annotation quality in this setup.
At the same time, aggregation for open-input labeling appears to be challenging (especially for voice characteristics) and needs to be investigated.
Dataset details
We are sharing 500 annotated samples from the dataset we created using our annotation methodology in the CLESC dataset (Crowd Labeled Emotions and Speech Characteristics) by Toloka. We hope this will help with research and innovation in emotional AI. The voice recordings come from two open-sourced datasets: Common Voice and Voxceleb, and are enriched with detailed emotional annotations by both in-house experts and the global crowd. The dataset can be downloaded here and is under the CC BY 4.0 license.
Summary
Crowdsourcing can not only help to capture complex topics like emotions but also does so across multiple cultures and languages. However, this is just the beginning as crowdsourcing can efficiently annotate important noises, such as sighs, pauses, sobs, and chuckles, evaluate the realism and trustworthiness of generated audio, and even extend to assessing the quality of generated images or videos, analyzing infographics, and more.
Need to scale your annotation process for recordings, videos, or other types of multimodal data? Reach out to Toloka, and let us help take your project to the next level.
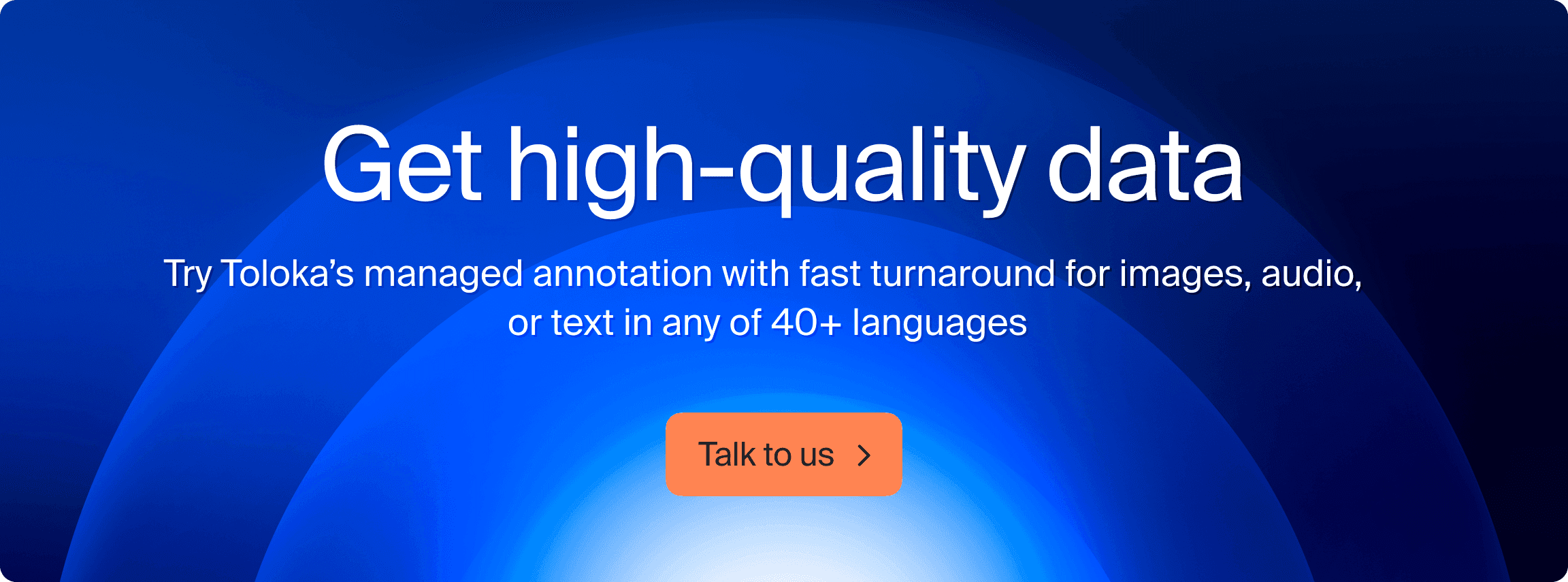
Article written by:
Updated:
Nov 18, 2024