Social Media Monitoring with Toloka
We'll talk about how crowdsourcing helps the Yandex support team on social media.
Social media monitoring is no news: the first services that were catching online mentions appeared as much as a decade ago. But there is still a problem: how can we automate mention filtering to only read what's important and ignore whatever is irrelevant?
If a company is mentioned not that much, say, up to ten mentions a day, you can filter them yourself. This usually happens like this: you read a mention, analyze it, and make a decision.
If it's important, you show it to the Customer Care team, if not, you mark it as insignificant in the monitoring system. If there are several hundred mentions a day, you'll need a staff of assistants. But a company might be mentioned thousands of times a day, mostly in passing or to draw attention to a post.
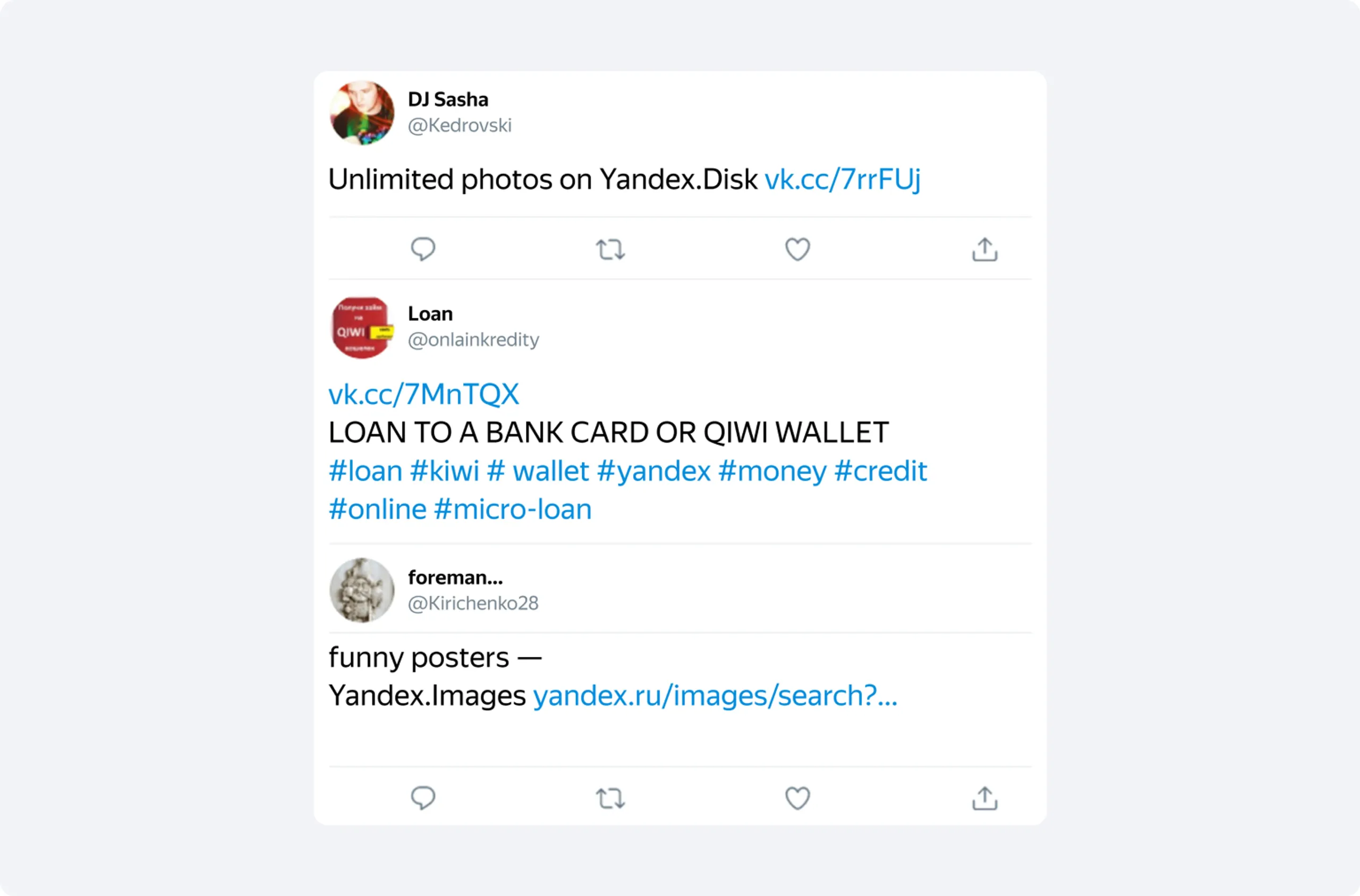
Post examples
Yandex is mentioned in social media more than 80,000 times a day. That is, every second something is written on the web about Yandex or some of its services. Our goal is to analyze this flow within 15 minutes. A quarter of an hour after the content gets into the monitoring system, the social media communication service operators should receive mentions that need to be responded to.
Crowdsourcing
This analysis requires an average of about 30 full-time employees. This is expensive and not very efficient, so we built our own system for filtering mentions. At the heart of this system is the Toloka crowdsourcing platform. Over 40,000 Tolokers complete simple tasks and earn money everyday. For example, the task can be to identify what is shown in an image, choose the most suitable option from multiple ones, or go to a specific location and take a picture of a certain object.
Filtering mentions in general is too difficult a task for tolokers. But it can be decomposed into a number of simple tasks: to identify the service, to evaluate the tone (positive, negative, or neutral), and to determine the importance of a message. Tolokers can accomplish tasks like this if they're given short and clear instructions.
Task 1: separate the important from the unimportant
All references are divided into three groups:
Useless. These are spam and off-topic messages like "Read our channel on Yandex Zen...", "Here is my Yandex.Wallet... ", or "My dog Yandex did a funny thing today...".
Insignificant. These are mentions on the fly and those which do not require your attention or response. For example: "I read on Yandex that Kai Metov released a new album...", "Did Yandex ban you?", and so on.
Important. This includes reviews, suggestions, problem reports, and other mentions that require a response. For example: "I like Yandex search much more...", "I am deleting all my Yandex apps...", and so on.
Here's how this task looks for a Toloker:
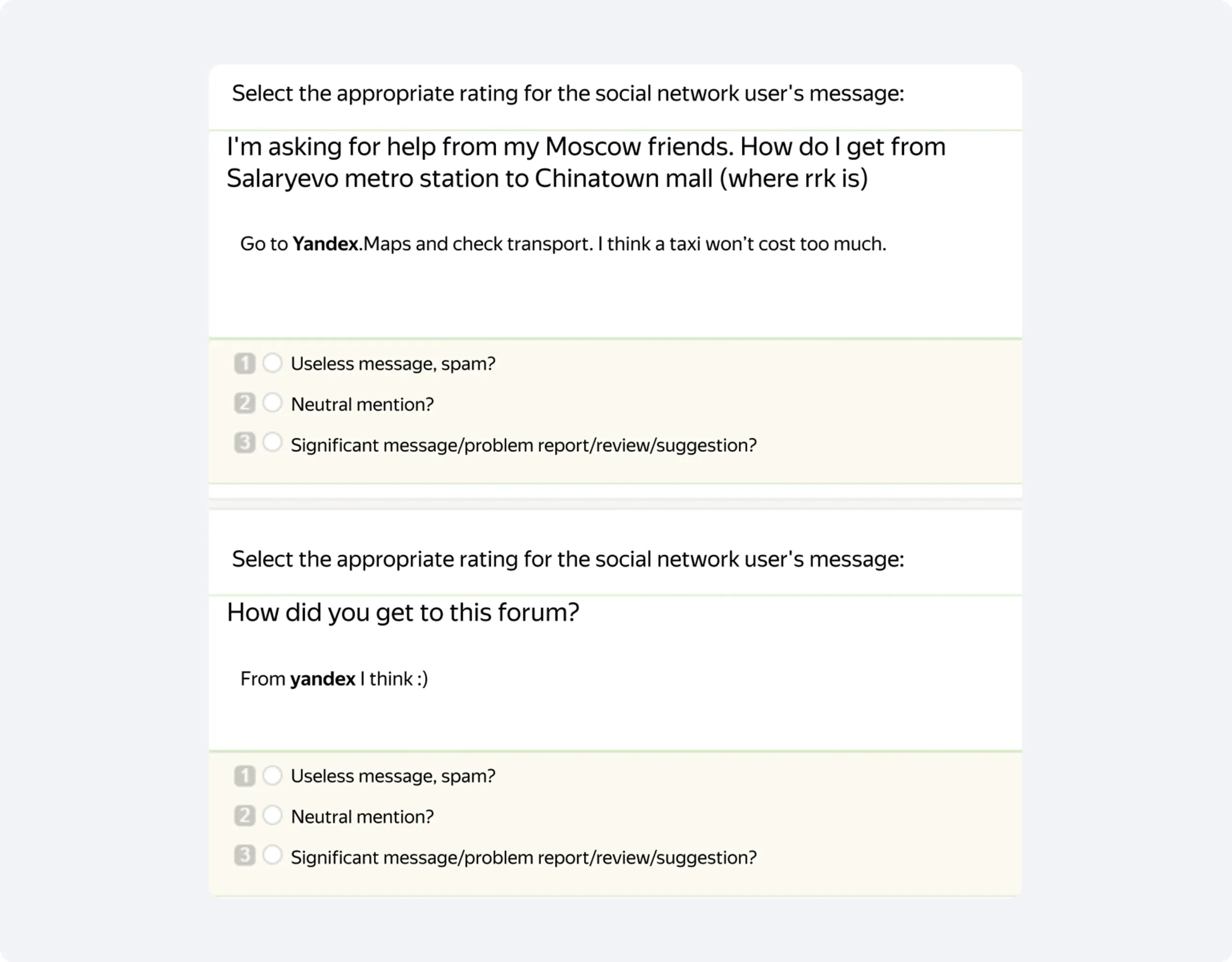
Content relevance task
Task 2: understand what service or product is in question.
Often this question can be answered automatically. Often, but not always.
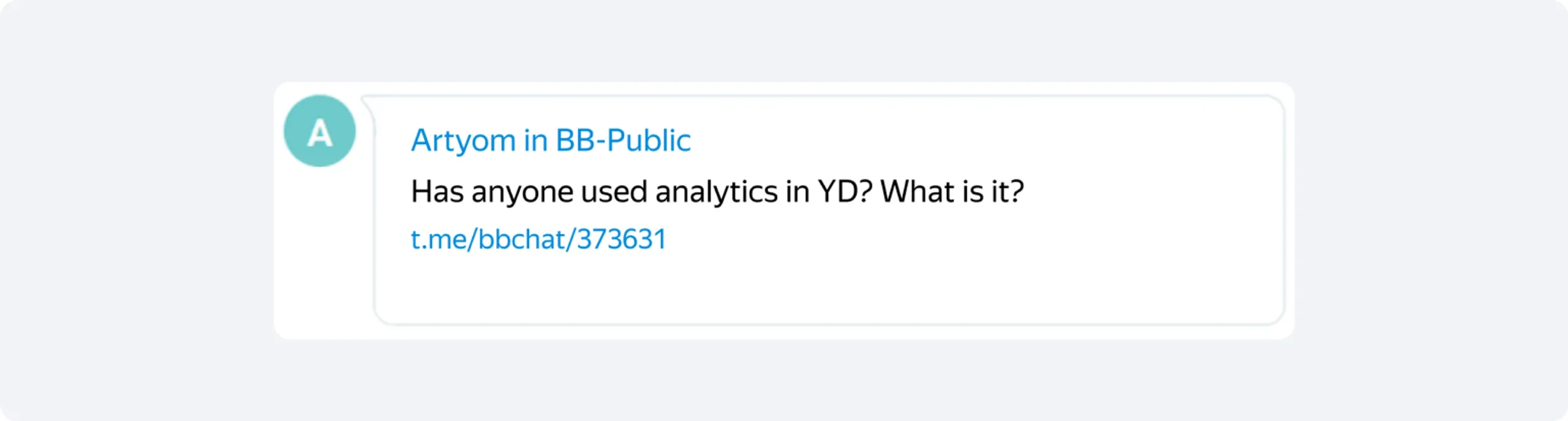
Question
What analytics are they talking about? This could be Yandex.Drive, Yandex.Disk, Yandex.Direct. Here the context will help the toloker, such as the name of the chat or the topic of discussion. By the way, the correct answer in this case is Yandex.Direct.
Task 3: identify the tone
Mentions can be positive, neutral, or negative. Social media monitoring systems can determine the tone automatically, but do not always do this correctly. Here's an example:
In a chat of Yandex speaker users, a video leaked of a working speaker with gesture control
Yandex officially announced the release of a new mini version of Yandex.Station
The monitoring system will treat the first message as negative and the second one as positive. However, it's not the news itself that is important, but the attitude of users to it, so in fact the tone of both messages is neutral. Here's another example:
Yandex.Traffic shows 10 congestion points! AAAAAAH, what bad timing :( It's just awful!
The monitoring system will mark this mention as negative. However, tolokers are human beings and they understand that the person is unhappy with the traffic conditions rather than the service performance.
Task 3: Quality control
To make sure that mentions are filtered efficiently, you need to train and control tolokers.
For each task, you should write instructions and only allow those who have read them and passed online testing to do the task: you can implement testing directly in Toloka.
To minimize the impact of individual errors, you can use an overlap when each mention is marked up by multiple performers.
To filter out dishonest performers whose work is just hit and miss, you'll need honeypots. These are verification tasks with a known response, which are mixed in with mentions that are not yet marked up.
Variable loads
Tolokers work around the clock, so filtering is going on day and night. If things are calm on social media, they will do other tasks. And when a storm begins and the number of mentions increases dramatically, you simply involve more tolokers and mentions are still filtered within the same 15 minutes.
A tweet that once caused a very heated discussion:
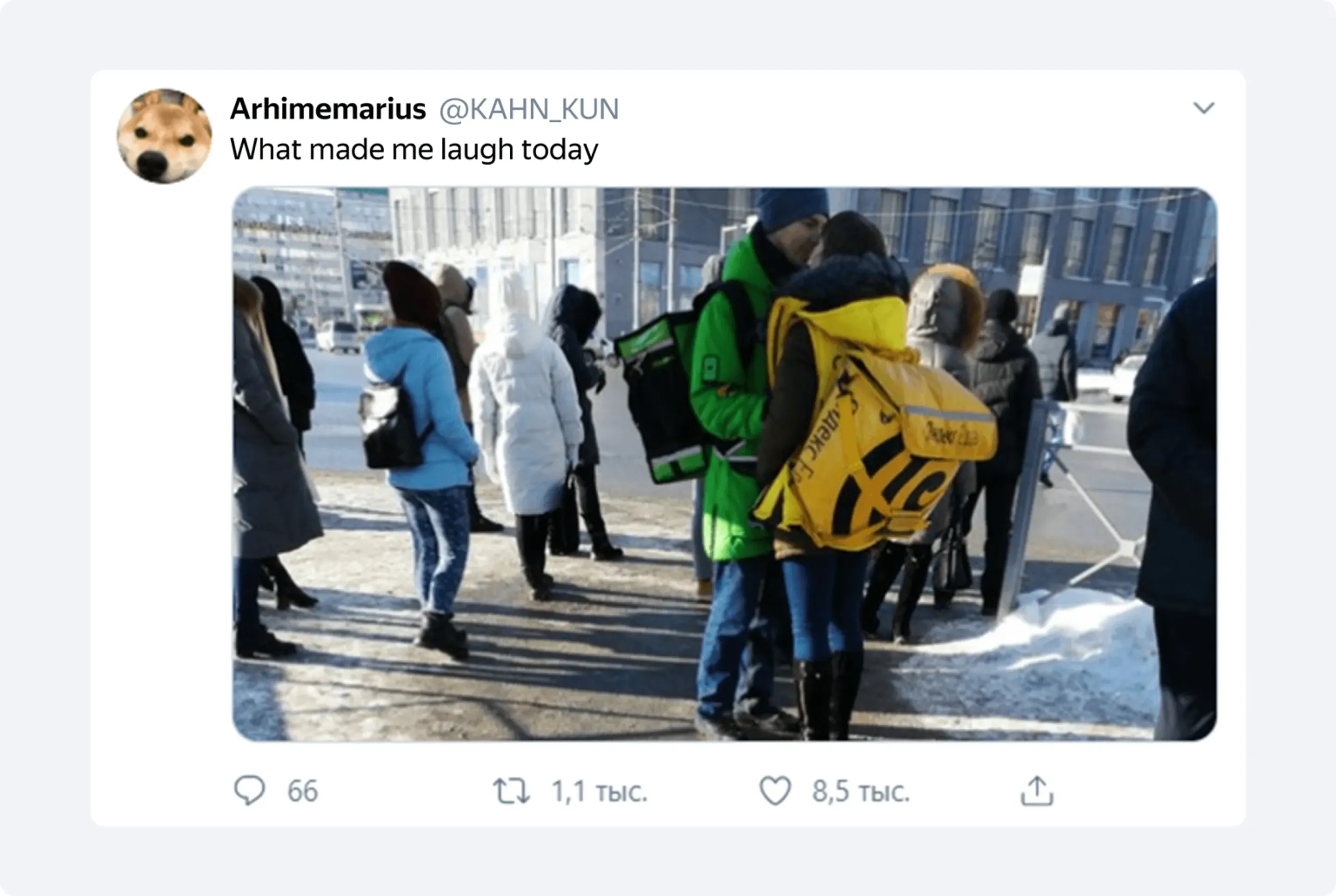
Tweet that caused a discussion
Results
Thanks to the markup done in Toloka, operators are only shown significant mentions, which make up just 9% of the total. Another 14% do not require any attention, and 77% are spam or offtopic messages in the first place.
Quick response. An operator receives posts and comments with markup 15 minutes after they are posted on social media. Within 30-40 minutes, they may already provide a response.
The company knows what users are saying about it. Monitoring provides general data (where something is posted, the number of subscribers, the gender and age of the post author), and markup in Toloka adds information about the tone and topics of mentions.
The most important thing is that you can monitor the mood of users and how it changes. To do this, the tone of each mention must be measured twice: the first time when it gets into the monitoring system and the second time after the operator communicates with the user. In one out of three cases, they manage to get a change of heart as a result of their chat.
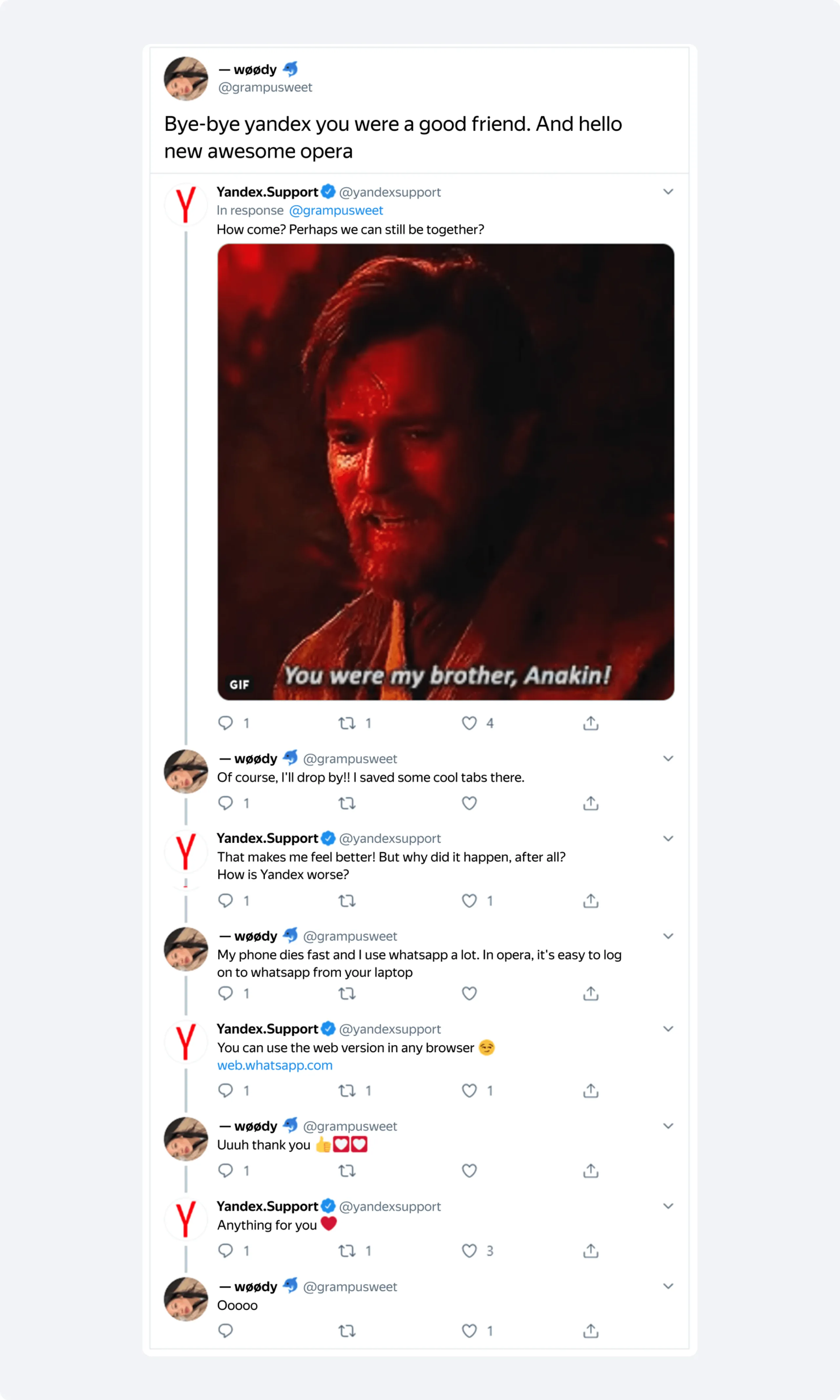
Successful chat