Ad image moderation for a news aggregation service
About Taboola
Taboola is one of the world's largest content recommendation platforms, curating online content for users based on their personal interests. Their two main types of stakeholders are content providers such as CNN, NBC, and MSN.com and advertisers who pay to have their ads displayed in the newsfeed.
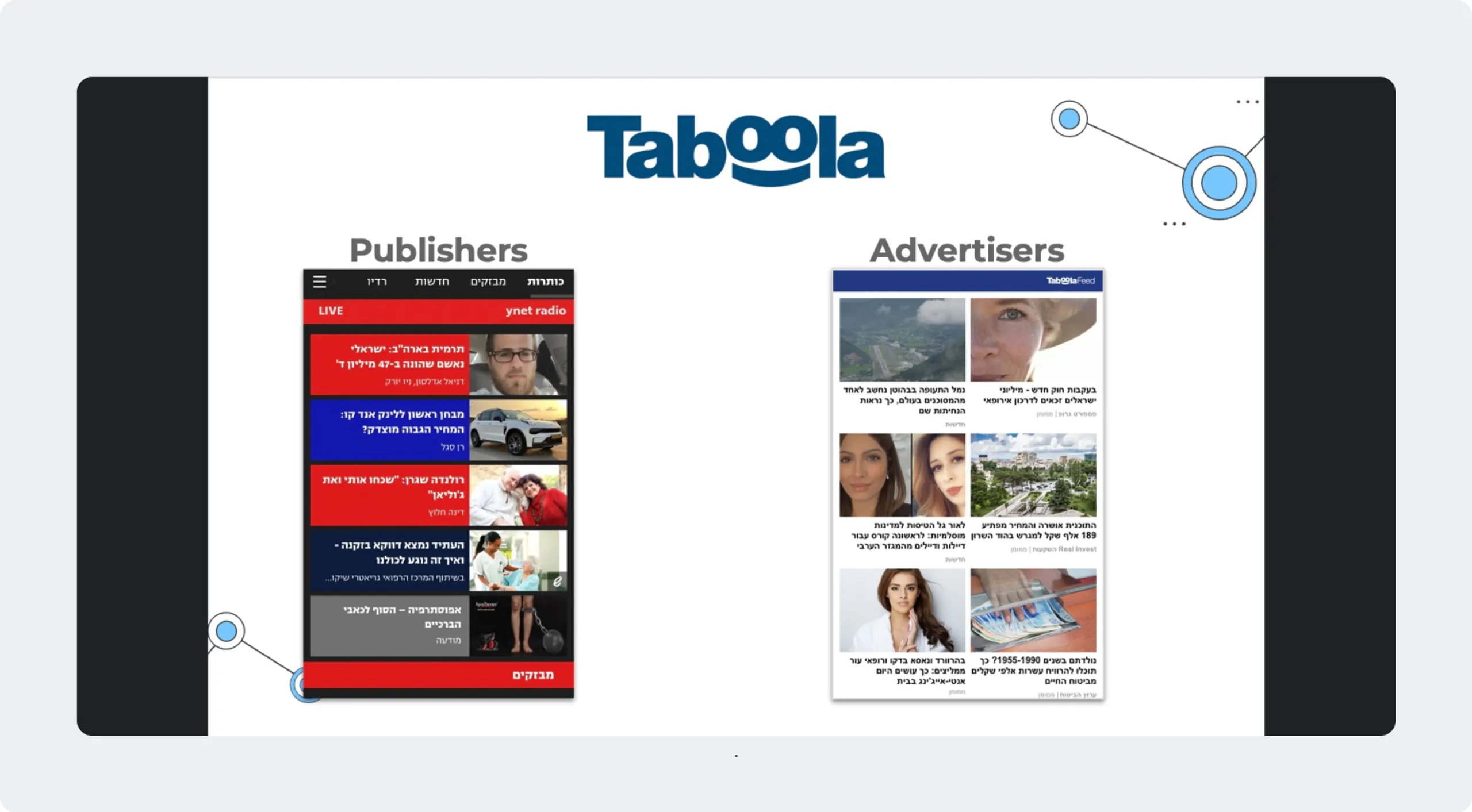
One of Taboola’s priorities is to regulate the ad content on their website. They have an in-house team of content moderators dedicated to this task, but they face several challenges. With Toloka, they were able to scale an AI moderation solution and reduce the need for manual review.
Challenge: Policy rules and content moderation
Major publishers do not want their newsfeeds to include disturbing, fake, or adult content. Therefore, as a service provider, Taboola’s first goal is to prevent the display of these images on the network.
The second goal is to label ads by category. Because Taboola partners with advertisers across a wide variety of ad categories and quality tiers, news publishers can choose which types of ads will appear in their feeds. For instance, companies whose content is targeted at children, like Disney, may want to exclude ads for alcoholic beverages. It’s important for the content moderation team to label ads correctly so that they don't end up in the wrong feed.
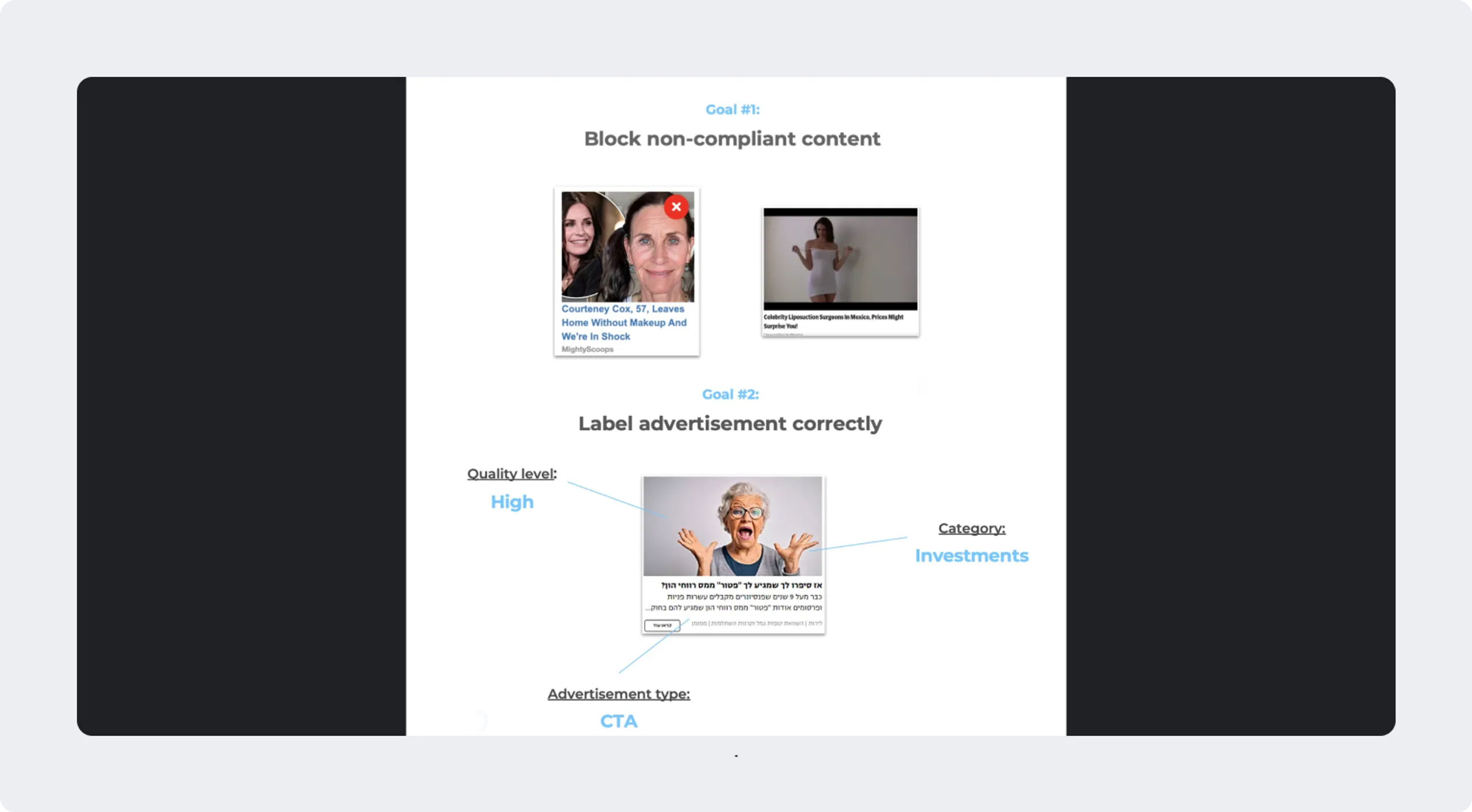
Taboola’s moderation pipeline
To overcome its main moderation challenge — an in-house team receiving too many images for manual review — and to make the process easily scalable, Taboola employs a combination of technologies, including automation, AI, and human labeling. Let’s take a closer look at each of them.
Automation works well when there are predefined rules, and the decisions are relatively straightforward. For example, if an image that has been rejected by human moderation shows up again, automation will easily identify it as non-compliant and reject it.
The second stage uses ML models, which have previously proven effective in making decisions on standard issues like image safety. How does it work? When an image is uploaded to the model, it first detects and highlights problematic areas, such as adult content, and then decides whether the image is compliant. If it isn't, it's "red flagged."
On top of these technologies is the human evaluation performed by the Content Review team, who deal with the most difficult, vague, and risky cases. To optimize the manual review process, the Taboola team identified an area where AI could help reduce the workload: identifying similar images.
In Taboola’s experience, rejected images tend to come back to the platform repeatedly. Some advertisers try to trick the system by submitting slightly modified versions of the same image over and over again, which means the image is returned for manual review multiple times. To address this issue and improve the efficiency of ad moderation, Taboola created an AI-powered image similarity model that can recognize repeat images and reject them without manual review.
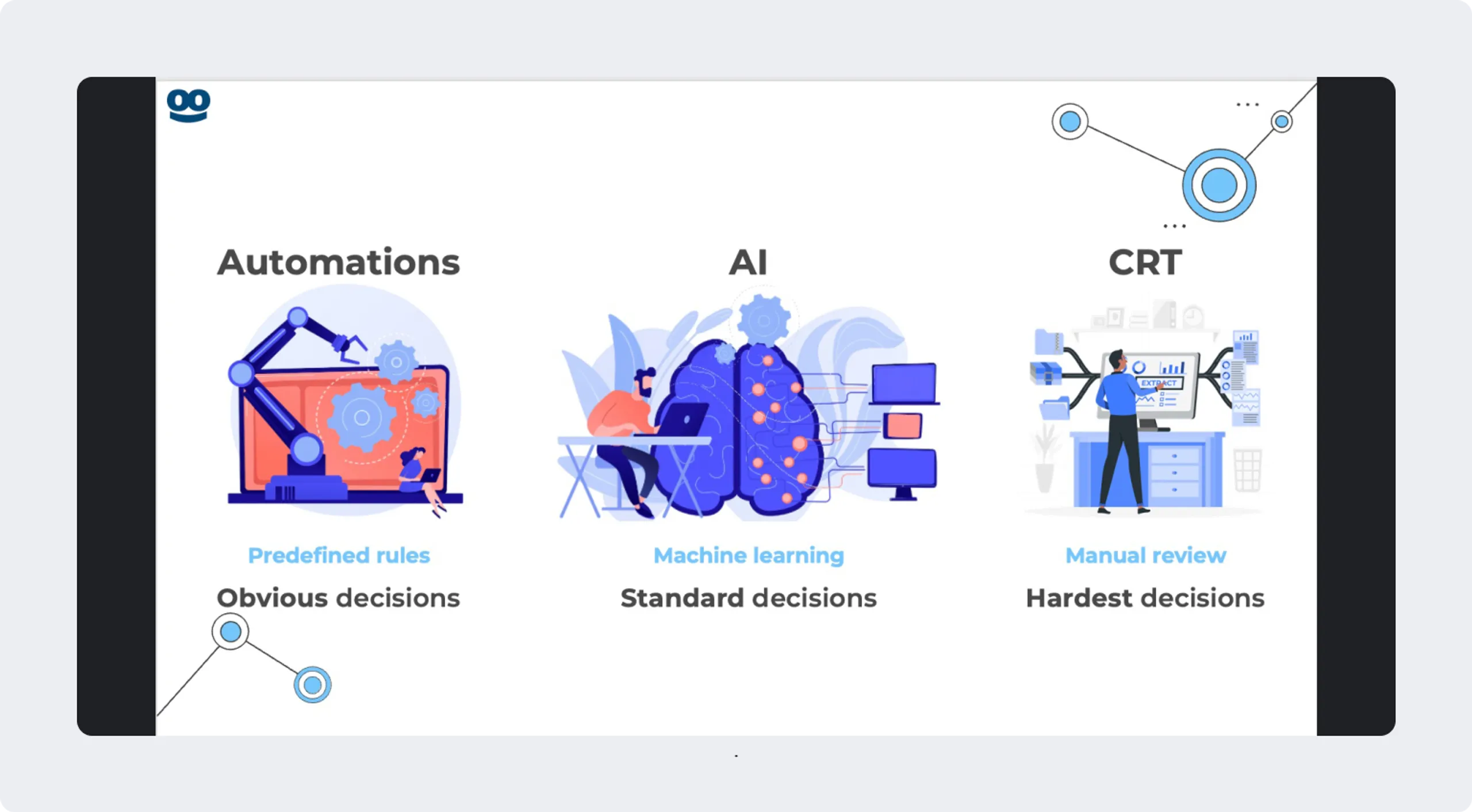
Solution: Automation powered by AI
Basically, the AI-powered automation model was designed to find similarities in images, labeling them as "similar" or “not similar."
The operating principle of the model is based on calculating the distance, or length of a line segment, between the two image elements being compared. Images are considered "similar" by the model if their distance is less than the set threshold. If the distance is greater than the threshold, the model will label them as "not similar."
However, certain images, such as resized images or images of two people who appear to be identical, were still difficult for the model to identify. Before moving on to the next steps, the team needed to establish ground truth to define the concept of similarity and use it to establish the threshold value for the model.
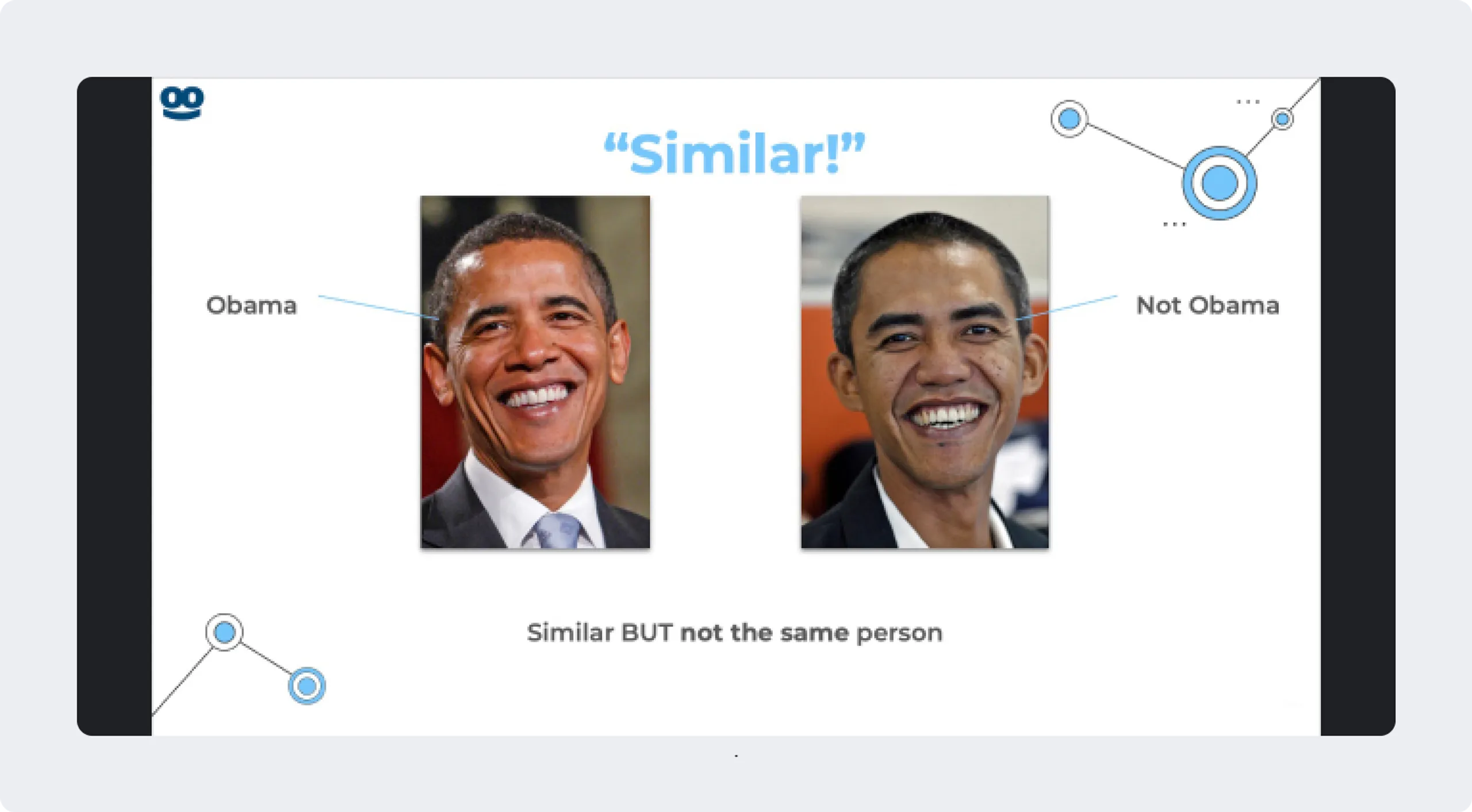
Toloka’s huge crowd provided the large volume of human evaluations needed for training the model. Each pair of images was given to several trained content reviewers to evaluate, and the majority vote (consensus) was used to determine the ground truth. The next step was to experiment with different threshold values and test the model on a few images to get the best results.
Results
The image similarity model proved to be effective and the results of the work quickly paid off. With this model in place, AI-powered automation processes now cover 11% of all ads, compared to 4% before. Manual review was reduced from 31% to 20%, freeing up time for in-house content moderators to focus on more important tasks such as providing better customer service.
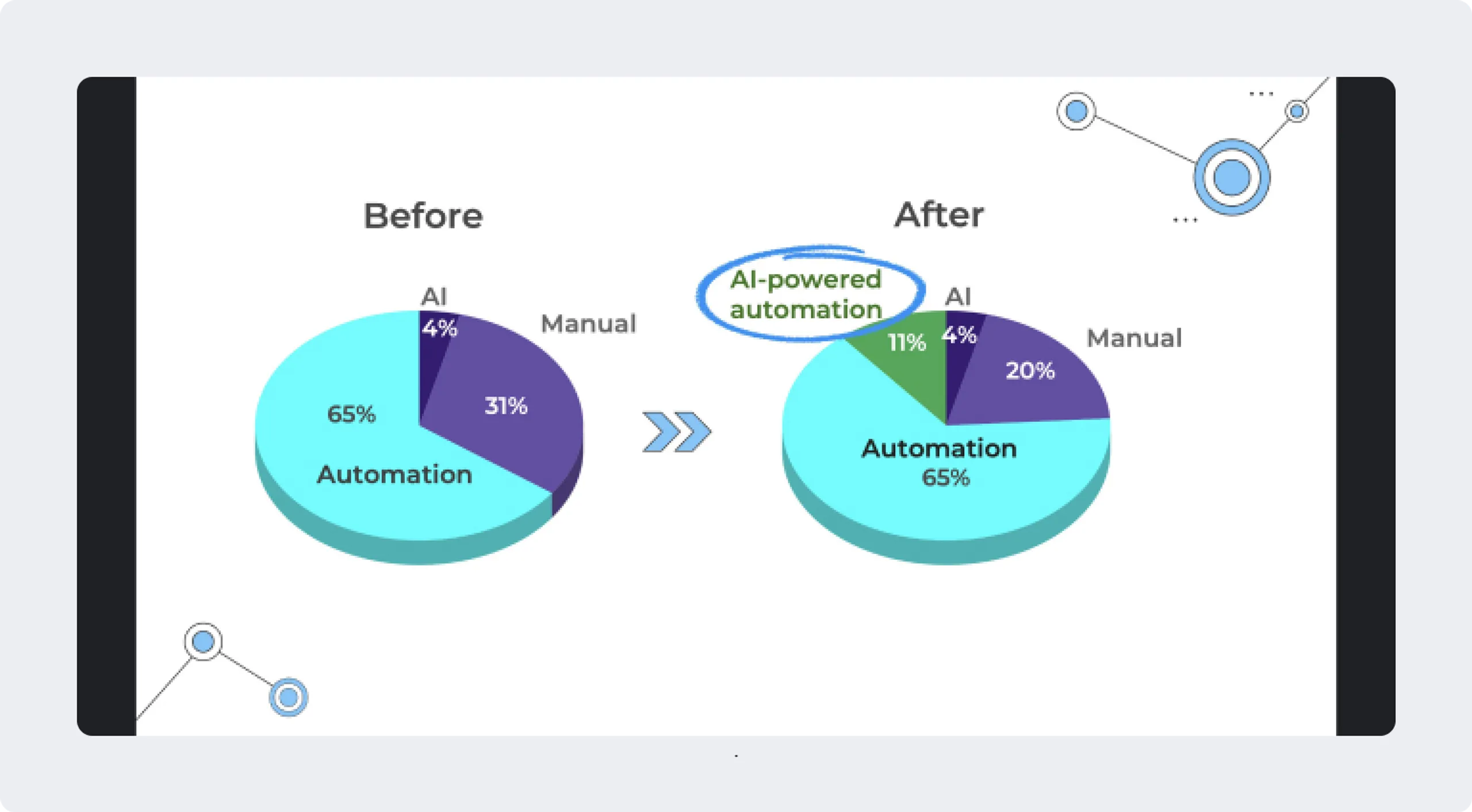
The case was presented at the Data-Driven AI meetup by Gal Cohen, Product Manager, Taboola. The full video is available here.