Data pipeline for e-commerce price matching: a case study
Competitor pricing information is a strategic weapon in e-commerce — and inaccurate information may ultimately lead to revenue loss. With the goal to sharpen their pricing strategy, Yandex.Market turned to Toloka for help with their large-scale price matching.
Challenge
Yandex.Market is a large online marketplace with a huge assortment of products. They need to continually collect information on the catalogs and prices from other major retailers in order to meet the following business goals:
Perform market analysis to learn about competitors’ product assortment and pricing.
Estimate the overlap in product categories.
Analyze competitor prices, find the market equilibrium, and dynamically adjust pricing where necessary.
Automated algorithms match items on retailer websites, but they don’t consistently perform well enough to achieve the business outcomes set out by Yandex.Market. Human-labeled data is needed to improve match quality and cover missing matches. For instance, human annotators are better at handling issues like matching identical items that have different names.
In pursuit of high-quality data, the company started off with an in-house labeling team, but it proved to be an expensive asset. After considering other available options, they chose Toloka for its quality, speed, and affordability, with an important deciding factor — the API allowed them to integrate with existing internal pipelines.
Tasks in Toloka were designed to serve three purposes:
Assess the quality of automatic matching.
Improve the quality of existing matches by removing incorrect matches.
Increase match coverage by finding more URLs of matching items on competitor sites.
Solution
When Toloka stepped in, there were two task components for the crowd performers to tackle:
Find and save a URL link – Tolokers identify specific products on various e-commerce websites.
Check and compare – Tolokers decide whether a pair of products with different URLs are the same.
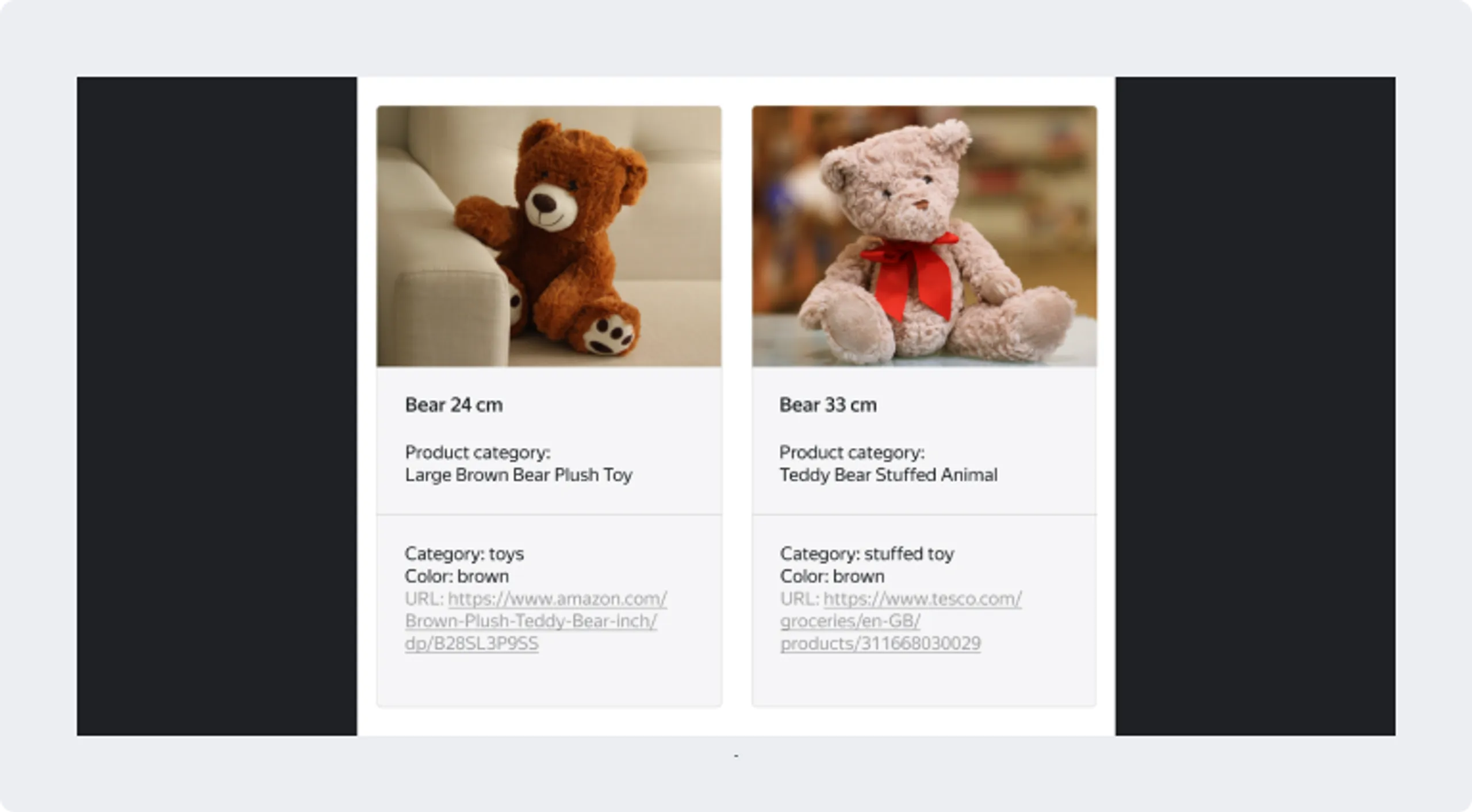
An efficient pipeline was designed to be compatible with the company’s internal processes. The pipeline includes two directions of interaction, from the dynamic pricing system to Toloka and back, with data labeling in the middle.
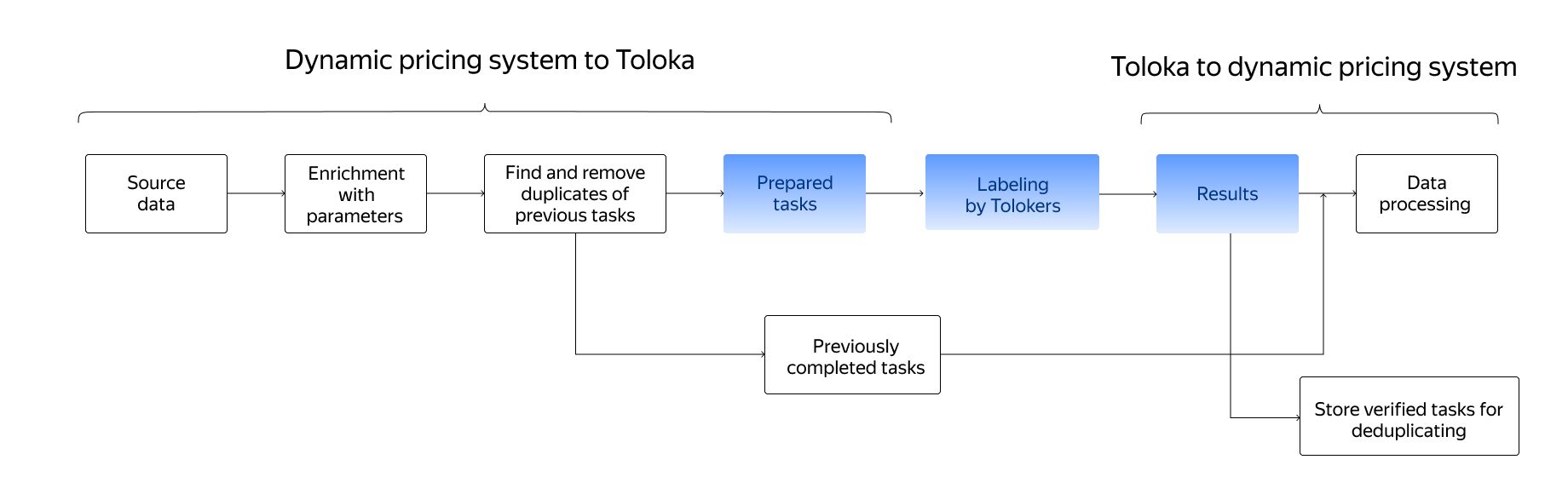
Four major steps are embedded within the pipeline:
The preparatory stage
Data collection
Quality control
Labeling and accuracy check
The company uses automated pre-labeling to prepare and verify each pool of URL links before sending them to Toloka for human labeling. All outdated and visibly erroneous matches are removed. The remaining links with potential matches are left for Tolokers to analyze.
Results
The Toloka pipeline provides 2.5% better coverage of key products compared to using automated solutions and in-house annotators. Tolokers are used on demand to find competitor products in priority categories — groups of products that contribute the most to GMV and have the greatest business value for Yandex.Market. As the project is ongoing, labeling accuracy and speed continue to improve as Tolokers build their skills. To maintain quality, the project’s honeypots (control tasks) are updated regularly to make sure that the URL links are active and all of the listed products remain in stock.