Finding Moderation Solutions to Meet Your Needs. Why Not Every ML+Human Content Moderation Will Suit Your Business
The rise of user-generated content makes it essential to apply a blended strategy that combines machine learning (ML) algorithms with human intervention. However, we need to recognize that the application of ML+human algorithms for content moderation is not a one-size-fits-all approach for every business context.
Here, we examine the challenges associated with content moderation solutions and find out the reasons why a fine-grained approach is paramount to meet the specific needs of different businesses.
What Content Moderation Solutions Are Available in the Current Digital Landscape
Comprehensive moderation strategy often requires implementing a combination of content moderation tools tailored to the specific needs and characteristics of a platform or business. Some common content moderation services and strategies used by platforms to moderate different types of content including text, images, videos, etc. are outlined further below.
Using In-House Human Moderators
No one, or should we say nothing, is better at moderating the subtleties of language or context-sensitive content than a human. Automated systems have difficulty accurately assessing nuances in text or visual data.
In-house moderators are in charge of screening user-generated content (text messages, imagery, and videos) to ensure that it complies with community rules. They decide whether to approve, restrict, or remove content. Take a look at the advantages and disadvantages of such moderation to figure out a better way to use it.
Pros
Human Judgment: Human content moderators can exercise nuanced judgment and cultural understanding in content evaluation;
Adaptability: They can quickly adapt to emerging trends, unique or unforeseen situations and effectively moderate context-specific content.
Cons
Scalability and Costs: Employing a team of in-house moderators can be expensive and challenging to scale with growing content;
Subjectivity: Humans may introduce bias, and their judgments can be inconsistent.
Instead of employing a team of moderators and managers, our clients usually keep just 2 or 3 moderators on board to check the model's performance and run quality control. Model's verdicts are always consistent and edge cases are sent to our experts.
Use cases: In-house moderators are well-suited for platforms where context and cultural sensitivity are paramount, such as niche forums, educational platforms, or support communities. In niche gaming online communities, using an in-house moderation team may be effective in addressing subtle cases of online harassment, inappropriate comments, or off-topic discussions.
When not to use: In most cases, human content moderation is not suitable for popular mainstream social media platforms, because moderators can struggle to handle the sheer volume of comments. And, recruiting a substantial team of full-time content moderators can be expensive. Allocating the resources for it can be a challenge for smaller platforms.
Furthermore, if your user community perceives content moderation judgments to be either inconsistent or unjust, it could potentially undermine trust in the platform and result in decreased engagement or even a change of platform.
Reviewing Only User Reports
This method of content moderation relies solely on reviewing user reports. It requires members of the community to flag or notify content moderators of content they find inappropriate. They then examine such reports to take action on the flagged content. Sometimes content moderation services are fully entrusted to site contributors, in which case a kind of ranking system is implemented, hiding the most unpopular content among users.
Pros
Efficiency: This approach focuses on problematic content identified by users, making it efficient;
User Involvement: Users become active contributors to platform safety.
Cons
Incomplete Coverage: Relying solely on user reports can lead to overlooking harmful content that goes unreported;
Potential Abuse: Users might misuse reporting mechanisms to silence others or flood moderators with false reports.
A Toloka solution enables customers to analyze not only reported content, but also any UCG. User protection begins even before the material is reported, as this helps detect inappropriate materials faster.
Use cases: User report-driven moderation works well for larger platforms with large numbers of monthly active users and with limited resources. Or for those wanting to involve their community in maintaining quality standards and community guidelines. Examples include online forums, discussion boards, and content-sharing platforms that are focused on the community.
Reviewing user reports as a content moderation tool should not be employed, for instance, on a review site. In case a user posts falsified negative reviews about a competitor's business, these may not be reported, and they could unfairly harm the competitor's reputation.
Outsourcing Human Moderators to a Company
Outsourcing content moderation to a third-party company involves engaging a dedicated service provider to handle content moderation efforts on the platform's behalf. Such businesses tend to have more expertise in the content moderation field. They can adjust to changing client needs, resize their expert team, and handle different types of moderation, such as image moderation, video moderation, or text moderation. Outsourcing companies may also have in-house experts who deal with brand reputation protection, and so on.
Pros
Cost-Efficiency: Outsourcing can be more cost-effective than maintaining an in-house moderation team;
Scalability: External companies often have the capacity to scale up or down as needed.
Cons
Communication Challenges: Outsourced moderators may not fully grasp the platform's culture and nuances, leading to inconsistent results;
Data Privacy Concerns: Sharing user data with external companies can raise privacy concerns.
Toloka offers a combination of machine learning and human moderators that both saves budget and covers corner cases. Data security is Toloka's primary concern, so we are committed to best practices and compliant with industry standards when working with client's data.
Use cases: Outsourced moderation is suitable for platforms of varying sizes, especially those with limited budgets and fluctuating moderation needs. Therefore, almost any platform can make use of outsourcing services to moderate content. For instance, dating apps, live streaming and online gaming platforms, news websites, online marketplaces, and so on.
When not to use: This type of content moderation service will not work for platforms that deal with niche content requiring a deep awareness of specific industries, cultures, or traditions. For instance, if an outsourced moderation team is not familiar with a local dialect, they may fail to recognize the nuances of sarcasm or humor, leading to the removal of harmless content.
Automatically Filtering Content Using Keywords
For this method to work, you need a set of keywords or phrases that are unacceptable on the platform. If the profanity filters detect at least one word from the list in the material published on the site, they may remove, hide, tag it for manual review, or warn the user.
The list is compiled by website administrators and moderators based on community guidelines, terms of service, or content policies. Surely to filter content efficiently, such a list should be updated and improved appropriately to keep up with the rapidly growing amount of user-generated content. This can also be achieved with the help of community members.
Pros
Speed: Keyword-based filtering is rapid and can instantly block or flag content containing certain terms;
Consistency: Keywords ensure consistent filtering without subjective interpretation.
Cons
False Positives: It may inadvertently filter out content that is not actually harmful but contains flagged keywords;
Evasion: Users can easily find ways to bypass keyword filters with slight modifications.
Toloka ML-models consider broader context, learn from new data and stay up-to-date adapting to the evolving nature of online content.
Use cases: Keyword filtering is ideal for platforms with a large volume of user-generated content, such as social media sites, where immediate blocking of explicit or harmful material is crucial. It's safe to say that almost all websites with comment sections and chat applications use keyword filtering to automatically moderate user comments.
When not to use: The filter is intended to detect blatant terms and their most common variations. However, users can still incorporate creativity in spelling and use invented words or homophones of the word that sound the same yet have different meanings to beat the filter.
Automated keyword filtering may either flag content that is not really infringing or overlook inappropriate content. So, it may not be as beneficial to use such a moderation process on a forum discussing breast cancer may inadvertently block legitimate user discussions due to automated keyword filters flagging the term 'breast'.
Developing Your Own Machine Learning Models for Moderation
When you develop your own ML models for content moderation, you understand exactly how to tune your algorithm and what types of content need to be detected or filtered. This may include identifying hate speech, graphic content, spam, or other inappropriate content.
Once you have chosen a fitting content moderation algorithm, you need to compile a diverse and representative data set of labeled examples to develop your own ML model. Such a training set should include both acceptable and inappropriate content. Labeling should indicate whether each piece of content conforms to or violates the platform's guidelines. The trained model can be subsequently fine-tuned to perform certain moderation tasks more efficiently, e.g., recognizing adult or suggestive images or videos, discriminative comments, etc.
Pros
Customization: Building your ML models allows for fine-tuning to match the platform's specific needs;
Scalability: Once developed, the algorithm can handle large volumes of content.
Cons
Initial Investment: Developing, training, and maintaining ML models can be costly;
Ongoing Monitoring: Models require constant monitoring and updates to stay effective.
Toloka's AI-only solution is available for free for a trial period. After you can discover the benefits of AI and human moderation for $1199 per month. That will solve most of your moderation challenges. Technical support and updates are also included.
Use cases: Custom machine learning models are best for larger platforms with sufficient resources to invest in development, such as video-sharing platforms or e-commerce websites.
When not to use: Websites featuring creative or artistic creative work, like poetry, literature, or visual art, may face challenges when using machine learning models for content moderation. ML models may have a harder time discerning the artistic intent behind certain art pieces. For instance, it may struggle to differentiate between user-generated art depicting nudity and explicit content, leading to the unintentional removal of legitimate artwork.
Using an External Moderation Solution with Machine Learning
Implementing external content moderation software with ML means integrating third-party services or software specializing in content moderation into your website or application. Many such solutions possess multimodal capabilities offering the opportunity to analyze a range of content types, including text, images, and video. Their deployment can be a significant time saver, as the development of such large models typically takes a considerable amount of time and resources.
External content moderation tools are configured with predefined moderation criteria that include community rules, policies, and machine learning models trained to detect certain types of undesirable content. The regular model updates ensure that they remain effective in detecting new forms of offensive content.
Pros'
Expertise: External solutions are often developed by experts and continuously improved;
Resource-Efficiency: Outsourced solutions save time and resources compared to developing your own models.
Cons
Lack of Customization: External solutions may not be tailored to your platform's specific needs;
Dependence: You may have limited control over changes and updates made by the external provider.
Flexibility and customization are the key features of our platform. In addition to basic classes and a limited number of languages, the Toloka team can create new classes for your platform within 2 weeks. Self-service features are also available on our platform for customers to block certain phrases instantly and change model confidence thresholds for banning content.
Use cases: External ML solutions are suitable for platforms seeking an efficient and reliable moderation solution, especially for e-commerce, news sites, or social networks.
When not to use: External ML models might not be able to identify unique, platform-specific slang or memes, potentially leading to false positives. Fine-tuning such a model may be impossible due to its configuration and parameters not being available for modification by external parties or it may simply be overly expensive.
Using an External Moderation Solution Combining ML and Human Moderators
Combining machine learning with human moderation is perhaps one of the most advanced and effective ways of content moderation currently available, blending the best features of both approaches. It lets online platforms benefit from the efficiency and speed of automated processes while retaining the ability of human content moderation experts to make nuanced decisions.
The combination of human labor and ML approaches to content moderation certainly requires more careful planning of the operations. However, an off-the-shelf external solution from a third party that specializes in processing digital content can be a great choice. The business would not need to go through all the steps of developing this solution, except customizing it to their preferences and implementing it.
Pros
Best of Both: Combining human and ML content moderation leverages automation and human judgment for higher accuracy;
Quality Improvement: Humans can fine-tune the automated system and handle complex, context-specific cases.
Cons
Cost: This approach can be costlier than using ML alone;
Coordination Challenges: Effective collaboration between humans and machine models requires careful planning and management.
"Startup" plan users get all the benefits of both AI and human moderation without additional charges. This means that your moderation budget will account for $1199 per month. This involves moderation in 10 classes & 9 languages, customer support with 24-h SLA and quality control. All the technical difficulties are sorted out by our team of AI-experts.
Use cases: This approach is ideal for platforms that need a comprehensive solution, such as social networks, where speed and accuracy are both important. It's also beneficial for platforms where cultural nuances and context play a crucial role.
External content moderation providers often specialize in content moderation and can offer their expertise and experience. For platforms that don't have the resources or expertise to build and maintain their own moderation system, this can be particularly useful.
When not to use:
It is not a good idea to implement such a solution on a photo-sharing platform, because combining ML with human content moderation is effective in detecting explicit images but may miss nuanced cases that require cultural context or understanding. The ML algorithms may also, for example, misinterpret or incorrectly process sensitive content on platforms dedicated to therapy that deal with particularly sensitive issues. This can cause damage or misunderstandings, which is why moderators with specific knowledge are better suited for these types of platforms.
Finding the Right Content Moderation Approach
Each type of moderation, as we found out, can be applied to different situations and platforms. Some of their uses, advantages, and disadvantages may overlap, but it is hard to know exactly which type will work best for you without understanding the exact specifics of your platform. Businesses have to evaluate their unique requirements, types of content that should be reviewed, and their resources first and only then embark on a journey to choose the right moderation services.
Though other combinations are possible, recently the combined ML and human effort to identify inappropriate content has gained popularity. This happened due to this approach being more versatile and incorporating the better parts of the two approaches to moderation.
However, enterprises have to realize their limitations. A stand-alone ML approach may not be able to tackle the subtleties of specific contexts, cultural references, and emerging trends on the Internet. But for sure it is fast, scalable, and consistent.
Human moderation, on the other hand, is slower and has limited scalability. But it offers the degree of control needed to complement the effectiveness of ML algorithms because it is equipped to interpret complex scenarios and consider cultural aspects.
Excessive dependency on ML with no human oversight may lead to a loss of user trust. Engaging humans to moderate your content, or allowing users to participate in moderating their favorite platform, may help maintain a human touch and build up trust in your community.
Also, it is worth keeping in mind that implementing pre-built content moderation tools or fine-tuning them with external experts can greatly simplify the process of incorporating moderation on your platform. With this in mind, you will be able to determine what type of moderation is best in your case.
Toloka Moderation Solution
Toloka is an example of an ML+human moderation solution. Toloka moderates texts, images, audios and videos in 10 languages and 10 classes in the starter package and offers 50+ languages and classes customisation for enterprises.
We detect content across multiple model classes
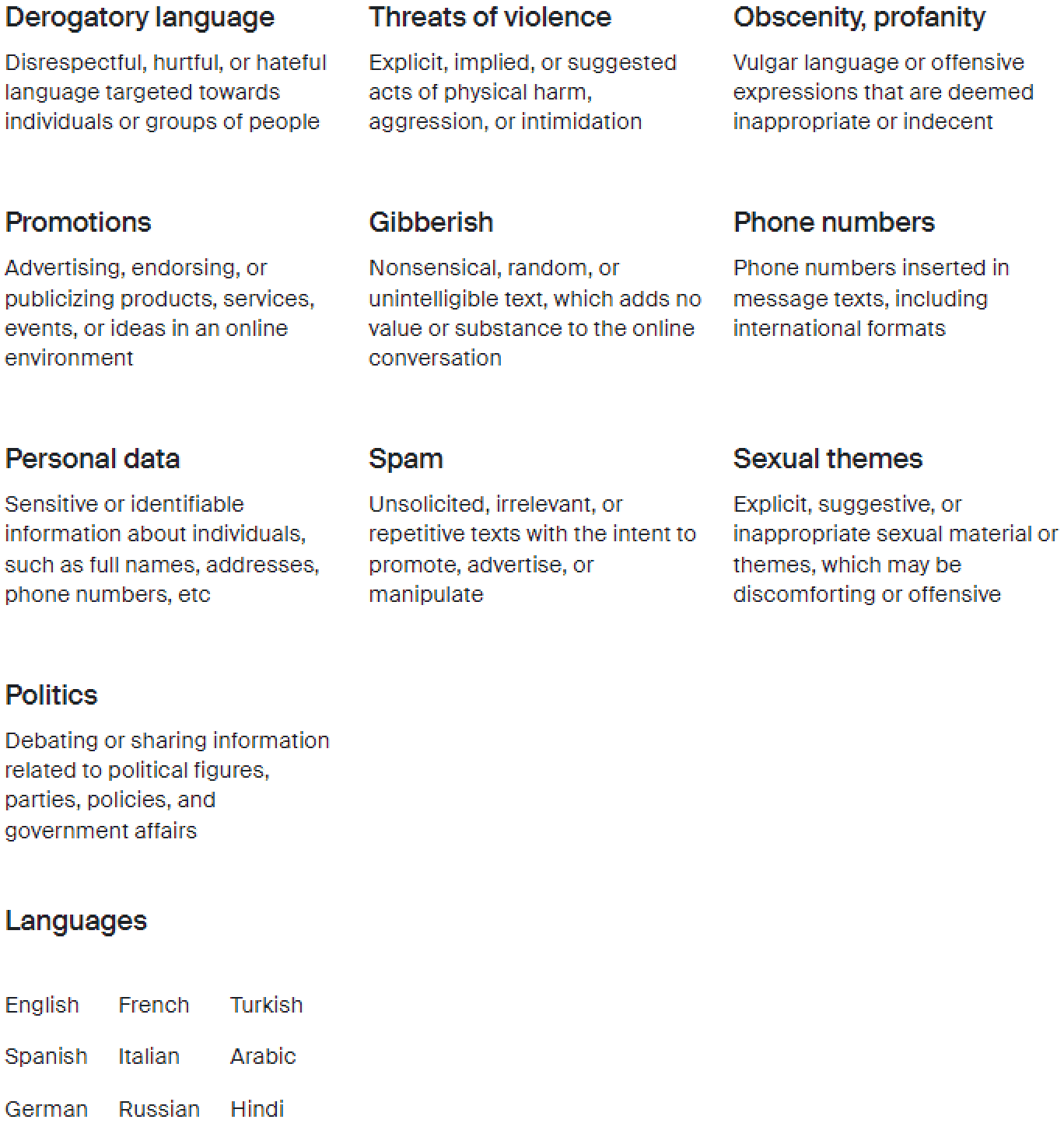
We rely on 100k+ MAU Toloka crowd connected to ML models to review unconfident items to provide accurate verdicts and retrain models in case of inconsistencies.
For the price of one human moderator Toloka provides a machine learning solution that delivers verdicts in less than 2 seconds ( or 7 minutes using human crowd), works 24/7 and does not decrease in quality.
At the same time we adapt rapidly to changing guidelines and instructions not only with 24/7 support but also providing self-service features:
black lists to filter specific words
statistics dashboard for big picture
item log to dive in specific examples
moderation rules adjustment interface
model testing