The GenAI frontier and the quest for high-quality SFT data
As large language models (LLMs) transform the AI landscape, AI teams are navigating the GenAI frontier with new approaches to model training and new expectations for fine-tuning data.
Supervised fine-tuning (SFT) is an essential method for training large language models (LLMs) to solve complex problems in niche domains. But to be successful, SFT datasets must meet stringent requirements for quality, expertise, compliance, complexity, and diversity.
Obtaining the appropriate fine-tuning data for your model can sometimes feel like a quest for the holy grail. Let’s look at what a high-quality SFT dataset entails.
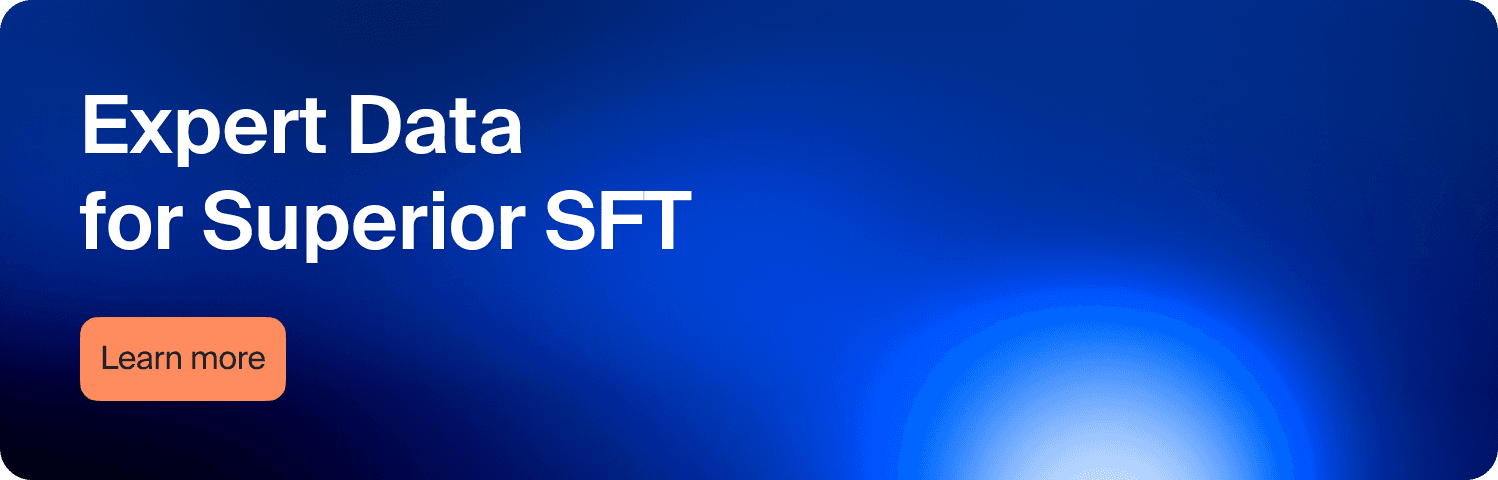
Why is high-quality SFT data so important?
In the age of generative AI, most models are pre-trained on copious amounts of generic data. Since foundation models are already good at handling basic questions, general human knowledge is not enough to make an LLM stand out. Consider an LLM based on a foundation model — it can handle a wide range of tasks, but it probably can’t answer a user’s questions about a nuanced topic, like the intricate details of EU law. This is where fine-tuning comes into play.
Supervised fine-tuning uses a curated dataset focused on a particular skill or area to adapt the LLM to a downstream task and achieve better performance. The model learns from positive examples of desirable answers to prompts, so for a model focused on law, this would entail a large set of complex legal questions and well-written answers. SFT datasets must include specialized, domain-specific knowledge, and the data must be original and unique.
Level 1: The quest for expertise
Expert-level fine-tuning data requires input from knowledgeable subject matter experts. Going back to the example of law, if we’re building a dataset to train an LLM to answer legal questions in the European Union, we want to collect contributions from professionals with a law degree and some experience practicing in the EU. These experts can apply their real-world industry experience to write conversations between an AI agent and a user, sharing their knowledge and showing the model what a truly helpful answer looks like.
To recruit and vet expert talent, we established the Mindrift platform in 2023. Building on Toloka’s rich 10-year history developing scalable data labeling technologies, the new platform supports a global community of freelance writers, editors, and domain experts — our AI Tutors. Their expertise covers more than 20 domains, including law, healthcare, STEM, compliance, coding, physics, humanities, and more.
Mindrift attracts a global network of highly educated professionals to work on complex tasks in a collaborative environment. In contrast to anonymous crowdsourcing, AI Tutors support and mentor each other and are dedicated to seeing a project through from start to finish, ensuring consistent results.
Level 2: The quest for quality
While quality is paramount for SFT datasets, it depends heavily on the qualification of the experts who generate the data. Our AI Tutors are vetted and tested to rate their skills in writing, fact checking, and generating ethical AI responses. They are assigned to projects in their domain of expertise, with project-specific training to prepare them to do their best work. Automated training and onboarding pipelines help experts get up to speed quickly, so they can start completing tasks with confidence.
Data pipelines include multiple stages that involve the whole team for best quality: each dialog is written, then edited, and verified by experts. A portion of the final data is checked by an auditor to monitor quality. Along the way, experts can discuss difficult tasks with the team and get direct feedback on their submitted tasks to hone their skills. Collaboration within the project team helps reduce fraud and cheating and encourages professional growth.
To further support our AI Tutors, Mindrift projects use LLMs and other forms of automation to assist with routine steps, so experts can focus on sharing their unique knowledge. For instance, AI-generated prompts offer suggestions to help the expert start writing a dialog. Built-in tools help check grammar, task requirements, factuality, plagiarism, and more.
Every data production pipeline uses a combination of automated and manual quality checks. But ultimately, it’s the in-depth knowledge and experience of each contributing expert that makes the data valuable for model tuning with SFT.
Level 3: The quest for compliance
Beyond the quality and expertise of the texts, data compliance requirements can be challenging. We need to guarantee that the data submitted by experts is unique and reliable — it is not plagiarized or under copyright, has not been used in other datasets, and is not AI-generated.
The Mindrift platform has built-in tools in the task interface to check for plagiarism and detect LLM-generated content, leveraging a combination of detection methods for best accuracy. To learn more about how and why we do this, read our recent article on detecting AI-generated texts.
In addition, our robust anti-fraud system includes a wide range of proprietary methods to ensure that submitted texts are genuine and original, and our experts are who they say they are.
Level 4: The quest for complexity and diversity
Gains in model performance from supervised fine-tuning depend largely on the dataset used — the size, quality, and diversity of the data, and the length of responses.
Diversity is important for exposing the model to a variety of natural human texts. A balanced dataset includes diverse subdomains, contributors, prompt types, and length and complexity of dialogs.
One way to help maintain diversity is by generating seed topics across a variety of subdomains to guide experts and inspire them. The generated topics also help to vary the prompt type, length, and complexity. In Mindrift, we assign a large pool of experts to each project and track task submissions to encourage diverse contributions and prevent scenarios where a handful of people do all the work.
No two fine-tuning projects are alike. Some SFT projects may focus more on response length, while others depend on prompt variety or response style. The goals for the model dictate the requirements for the dataset. Non-text modalities — image, video, and audio data — are also in demand, along with multi-modal datasets.
How we build SFT datasets
While datasets for GenAI are becoming increasingly complex, data production needs are the same as ever: high-quality data at a large scale, delivered in short timeframes and on budget to accelerate AI development.
The Toloka team develops custom datasets for fine-tuning (SFT) that include human-generated prompts and answers. We also offer data collection for alignment (RLHF) and model evaluation.
To achieve scalable data production, we build highly automated multi-step pipelines that turn implicit human knowledge into structured high-quality datasets. Here are some highlights of our data production pipelines for GenAI:
Synthetic prompts generated as a starting point to inspire domain experts to write detailed dialogs
LLM co-pilots in the task interface help experts work efficiently
Extra eyes on the data: multiple experts involved in writing, editing, and verification for each data point
61 proprietary anti-fraud methods
Over 40 quality control methods
With the right partnerships, the quest for SFT data doesn’t have to be long and difficult. The Toloka team’s experienced dataset architects partner with each client to define requirements and build efficient data production pipelines. With a solid foundation of human insight and technology, we achieve a final dataset that caters to the model’s exact fine-tuning needs.
Talk to our dataset architects about SFT
Article written by:
Updated:
Sep 4, 2024