How to boost content moderation efficiency with Toloka
Content moderation is the job of monitoring user-generated web content to make sure that nothing inappropriate, offensive, or disturbing gets published online. Things like false advertising, fraud, or sexually explicit images can seriously harm the reputation of an online business, whether it’s an e-commerce marketplace, an AdTech platform, or a social media site.
As online content grows exponentially, keeping the web safe is no easy task. Companies today use Machine Learning models to build their automated moderation systems. These systems rely heavily on high-quality, accurately labeled data for training and validating their models — and this is where crowdsourcing can play an invaluable role.
Use case: Yandex Zen
Yandex Zen is a popular recommendation service that provides user-generated content and uses ML algorithms to support automated moderation. The service takes the form of a personalized news feed that shows articles and videos from external digital media sources, as well as original pieces from its own blog. The website has 20 million daily active users, including 5000 daily new authors, the most popular of whom are able to monetize their contributions.
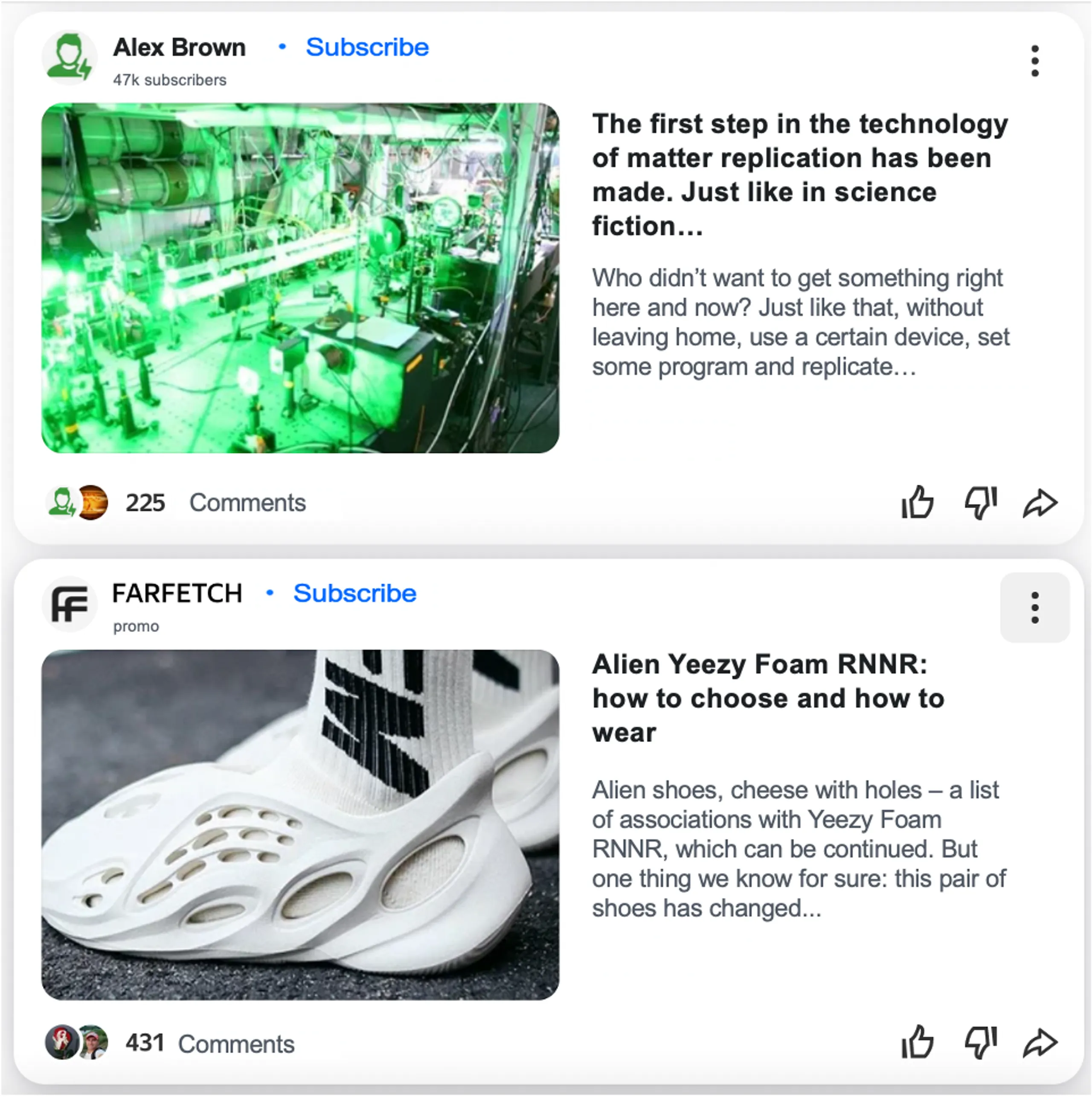
Examples of content on Yandex Zen
Since its launch in 2015, Yandex Zen has encountered 2 major issues:
Even relevant, personalized content on the platform sometimes crosses the line between the acceptable and the inappropriate, going from informative to provocative, sensational, and sometimes even potentially dangerous advice.
Profit-seeking authors try to cheat the website’s algorithm, creating questionable or substandard content in an attempt to increase engagement.
The platform relies on human moderators to cover the grey areas that their ML algorithms can’t handle reliably, as well as to monitor trending content in real time. The team found it was a challenge to keep enough moderators online 24/7, label enough content to train and validate their ML models, and maintain quality of moderation.
Challenge
Toloka was asked to utilize the power of the crowd and help improve moderation at Yandex Zen. Moderators were expected to identify the following types of violations:
Clickbaiting
Hate speech
Poor (and potentially hazardous) medical advice
Spam
Violent content
COVID-19 misinformation
Adult content
Illegal content and scams (information about illegal products and services + attempts to defraud other users)
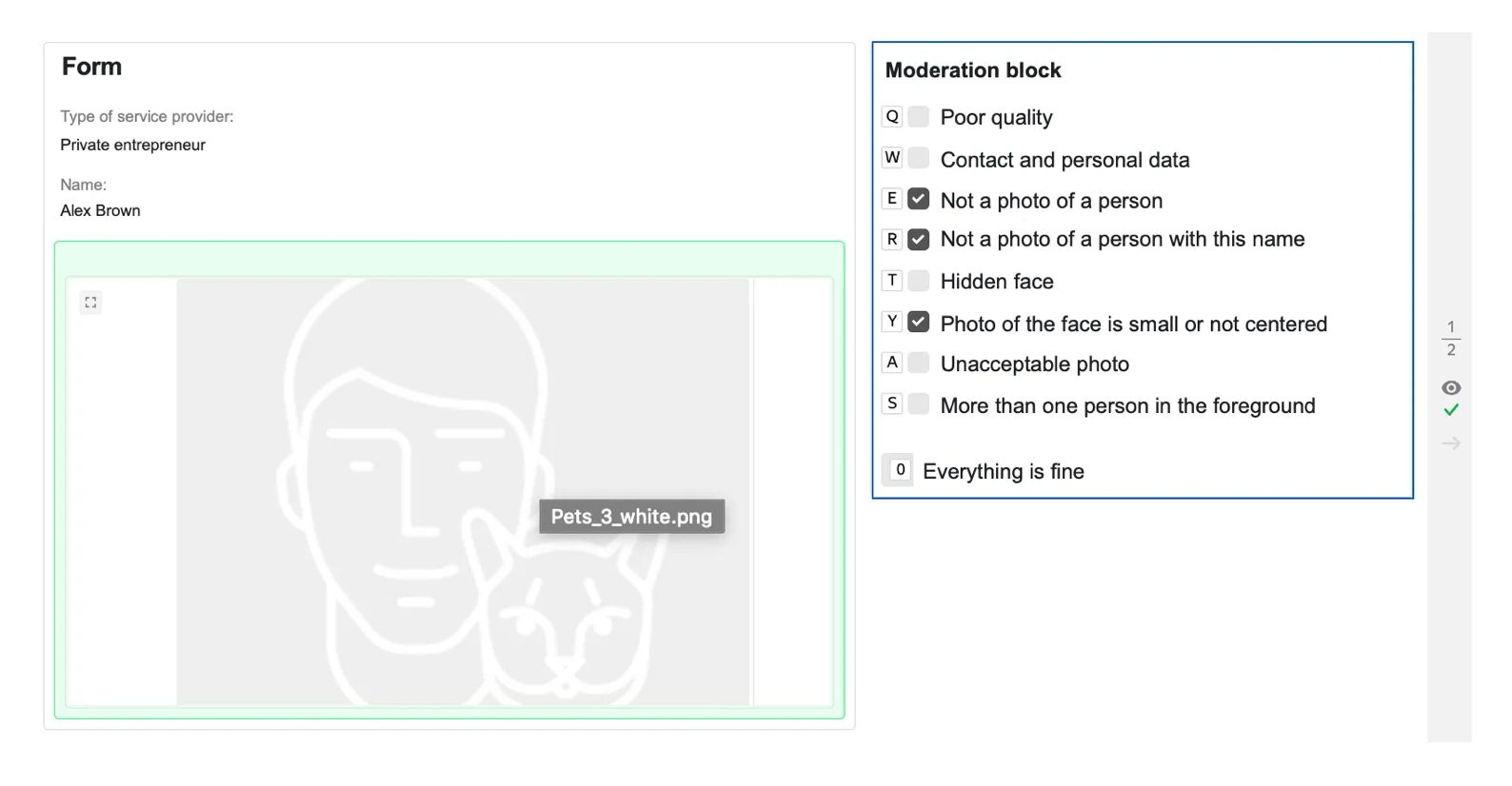
Example of a content moderation project
Toloka’s Crowd Solution Architects (CSAs) isolated 3 classification formats for the project – text, image, and video.
Normally, majority vote is used to improve quality on classification tasks. This means that each item is classified by multiple Tolokers and results are automatically aggregated to use the most popular verdict. However, this moderation project was a long-running continuous process, and majority vote revealed socio-demographic bias that degraded quality over time.
In addition, the website’s fast-paced environment with freshly published hourly content meant that moderation and control tasks had to be regular and frequent, while all trending content required real-time moderation.
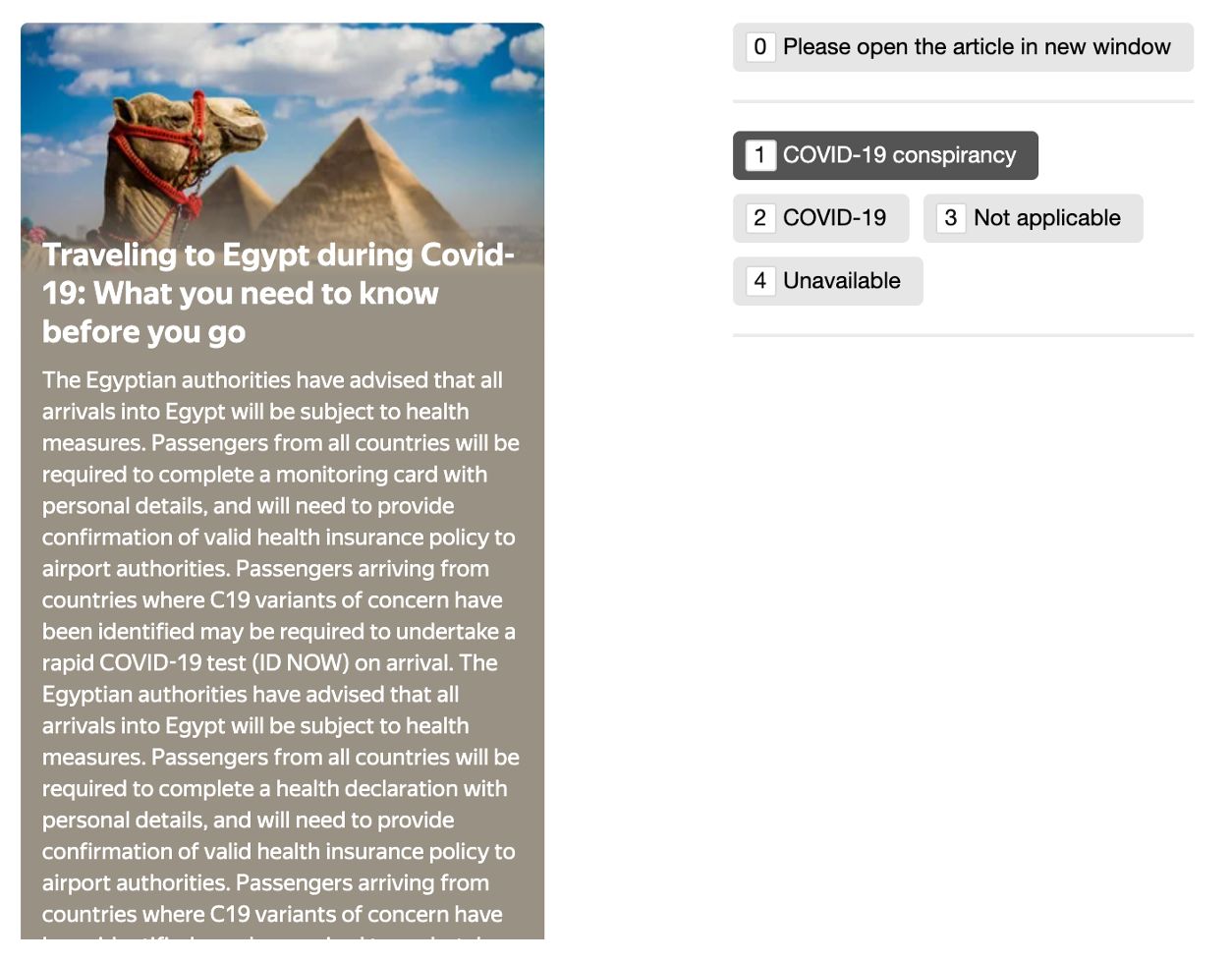
Example of a classification task about traveling to Egypt that was classified as “Covid-19 conspiracy”
Toloka created 10 projects for articles and videos, as well as 4 projects for the comment section. Each project contained around 10,000 daily crowdsourcing tasks.
Solution
The Yandex Zen team came up with a new infrastructure for moderating content efficiently. Initially, the majority of content is handled by automatic classifiers. Anything that falls into a gray area is passed on to the Toloka crowd for classification. This crowd is supported by in-house moderators, who verify the performers’ work and help control quality. A handful of expert moderators create control tasks with true labels, which are used for daily secret exams to monitor quality and reward those who perform well. In-house moderators can focus on trending content that demands careful consideration, while letting the crowd handle the majority of tasks.
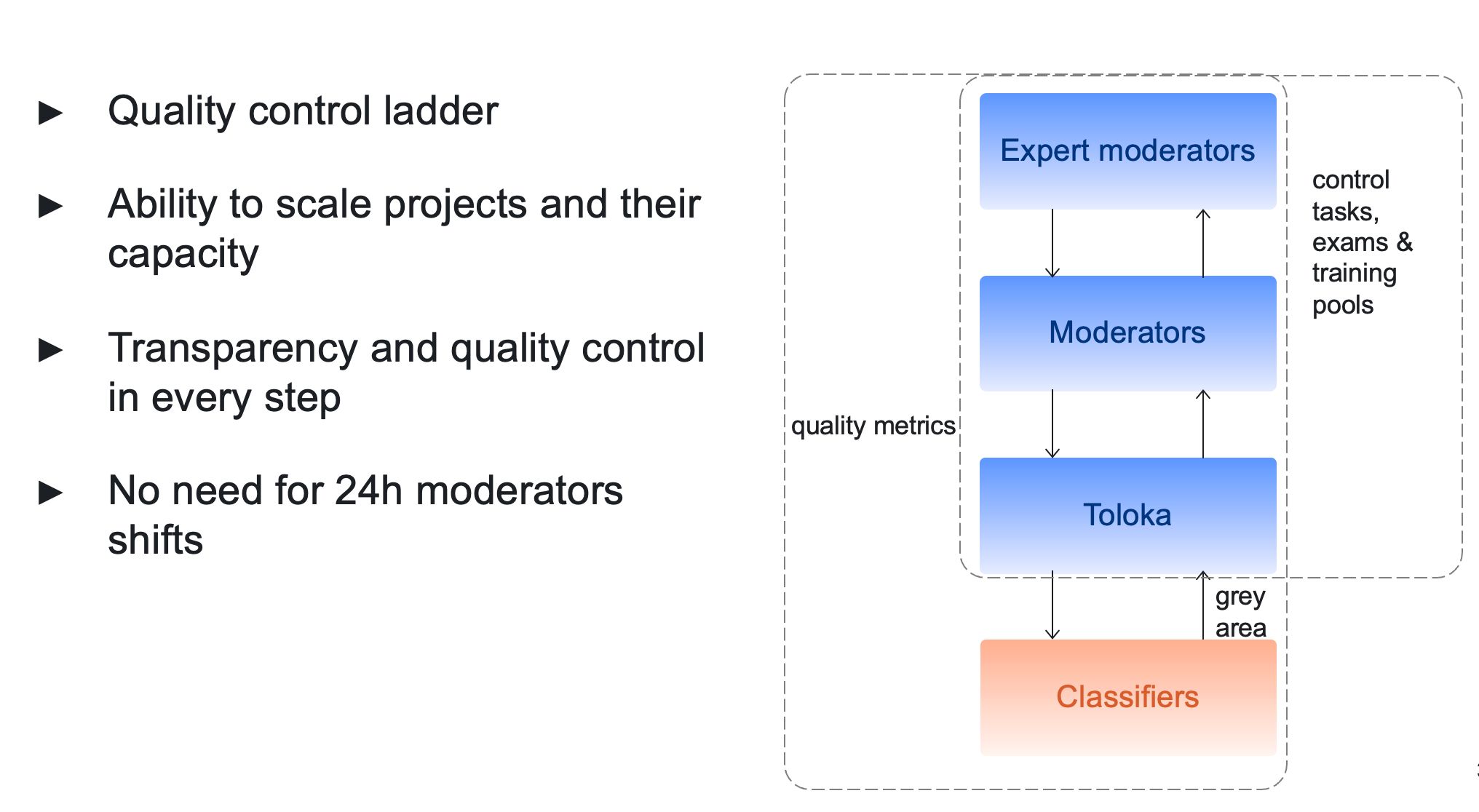
The pipeline enabled effective quality control, transparency, and scalability, and also reduced the need for full-time moderators.
Here’s what the new collaborative process achieves:
Successful decomposition of a large-scale labeling project into numerous simple tasks, which makes the workload manageable and increases the overall quality of moderation while reducing the burden on in-house moderators.
A new quality-based payment scheme that awards bonuses for accuracy and motivates both Tolokers and the in-house staff.
An interactive model that establishes an effective feedback loop among the moderators and the crowd, resulting in higher accuracy and improved task pool management.
As a result, moderation accuracy has risen significantly, reaching figures up to 98 percent. Content classification quality also improved in the first 2,5 months of working together with Toloka.
Results
As a result, moderation accuracy has risen significantly, reaching figures up to 98 percent. Content classification quality also improved in the first 2,5 months of working together with Toloka. If your business requires an affordable team of labelers for large-scale projects, including but not limited to classification tasks, be sure to get in touch with us – we'll be glad to help you build a robust data-labeling pipeline.