What Does a Data Annotator Do? Unveiling the Cornerstone of AI Projects
Data is the new oil fueling the creation and functioning of digital products and services. However, just like oil, unstructured data remains a raw material, even when properly collected and stored. Every stage of its further processing, whether manual or automated, adds value to the original information, helping to draw insights and achieve tangible results from its analysis.
ML projects, particularly those based on supervised learning, heavily depend on meticulously annotated data. By training their models on thousands of data points, engineering teams refine algorithms to extract relevant information from the input data they will process in the future. And they often need a reference to human judgment to do that effectively.
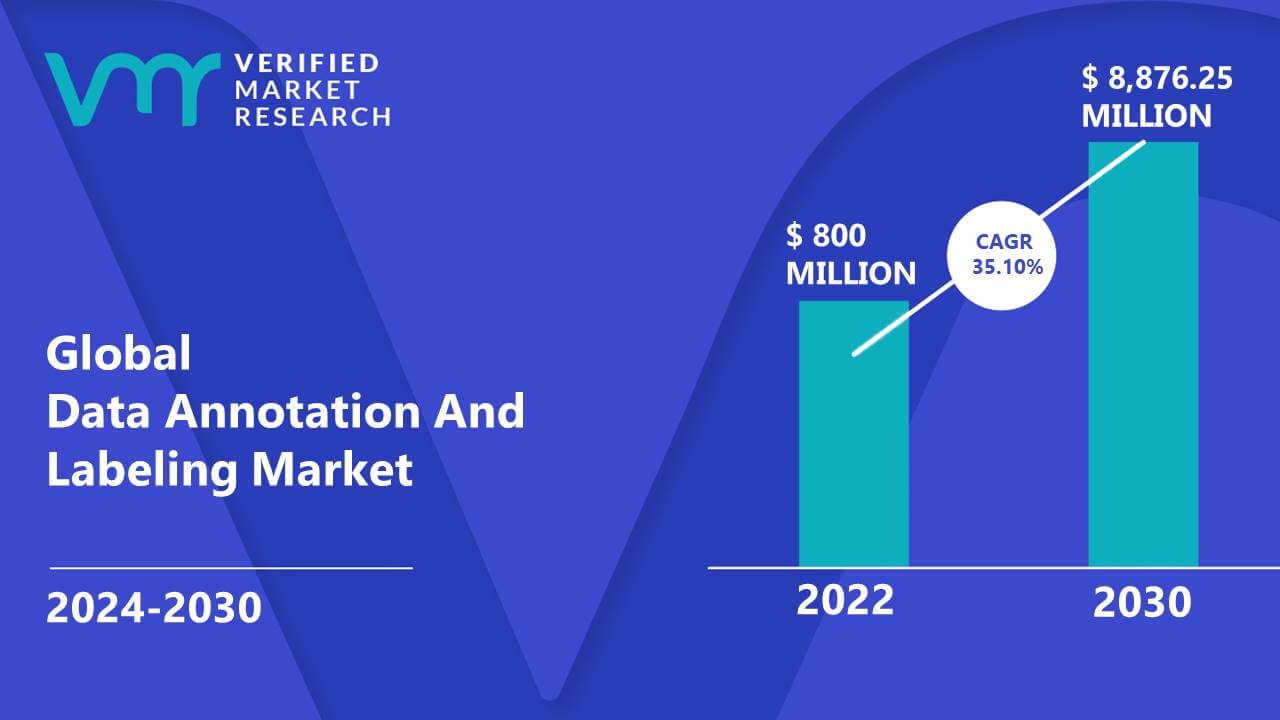
All researchers predict stable growth for the data annotation and labeling market. (Source: Verified Market Research)
Creating labeled datasets is a labor-intensive process, and it falls on the shoulders of data annotators. Working behind the scenes, these people play a crucial role in preparing the data that powers algorithms driving technological advancements and business value.
This article will explore data annotation specialists' responsibilities, working processes, qualifications, and career paths. We'll also review the competition between human data annotation and automated labeling methods, as well as the benefits of their collaboration in annotation workflows.
Data Annotation for Machine Learning models
Data annotation is the cornerstone of supervised machine learning, the most popular type of ML for practical projects. It implies converting raw data encompassing images, text, tabular information, videos, and audio recordings into annotated datasets by assigning a pertinent tag to each data point.
These basic labels may include supplementary textual or graphical information depending on the project's objectives. Manually labeled datasets serve as ground truth data for any supervised machine learning application.
Initially, a machine learning algorithm relies on human judgments to discern patterns and extract relevant insights from unstructured datasets. Data annotation bridges the gap between computer understanding and human cognition, enabling ML-based applications to identify objects, classify entities, and detect anomalies effectively.
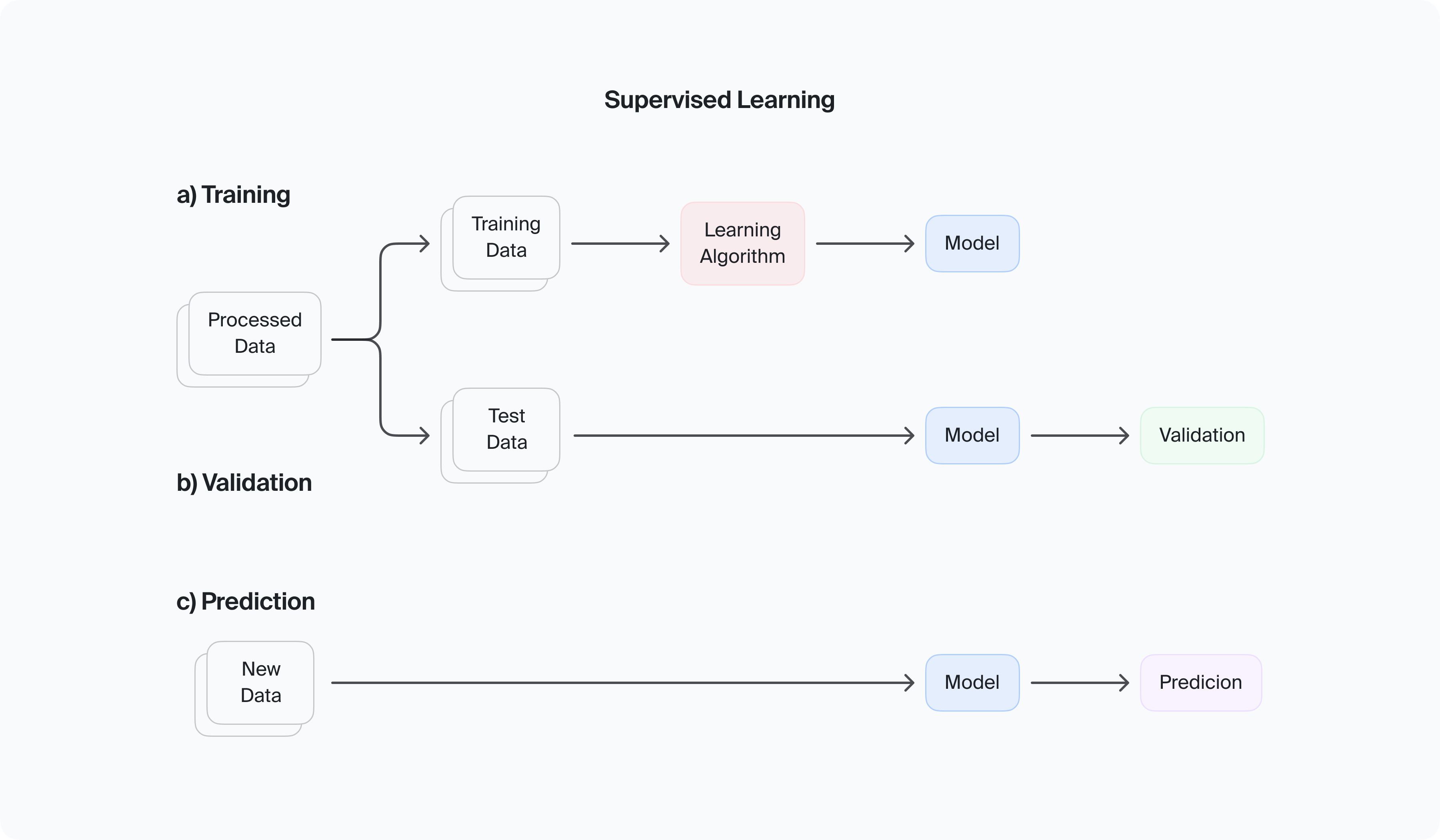
In supervised learning, original preprocessed data sets are divided into training data and test data. (Source: ResearchGate)
The data annotation process commences with the formulation of guidelines for human annotators, who extract project-relevant information from pre-collected data. Subsequently, a specialized team analyzes, categorizes, and provides comments, bounding boxes, and segmentation masks as necessary.
The manually annotated data serve as the project's benchmark, known as the 'ground truth,' upon which the accuracy of the ML model's predictions hinges. Therefore, ensuring the quality of data annotation through rigorous quality control measures is imperative for the success of any machine learning project and warrants consideration from its inception.
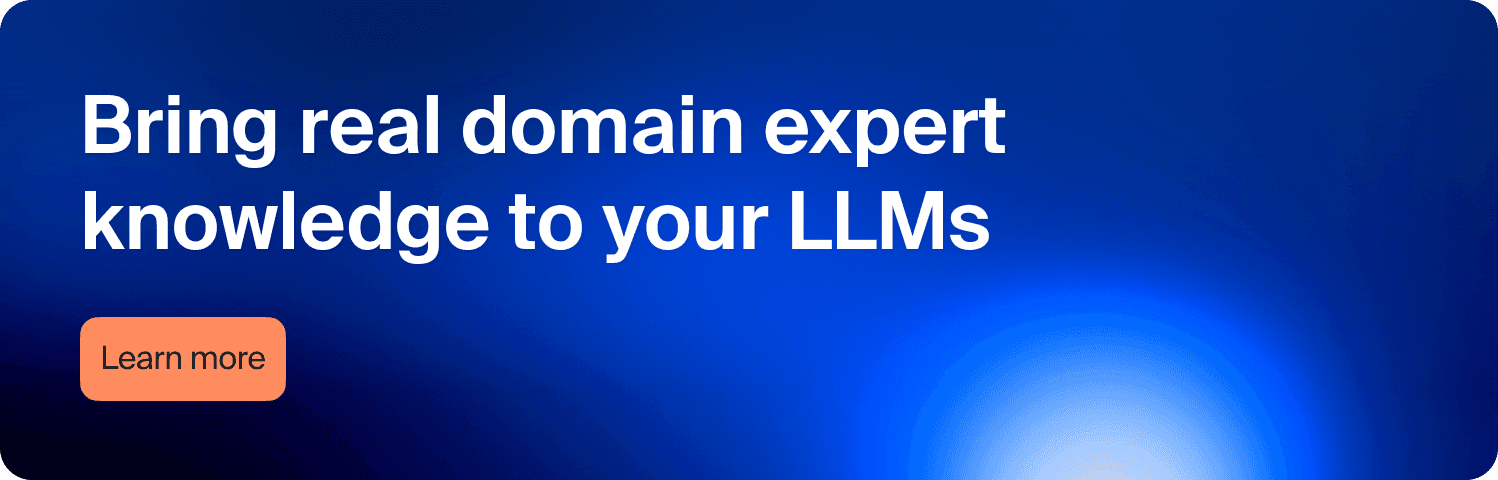
The Role of Data Annotators
Data annotators or data labelers (these terms are synonymical, although data annotation encompasses a broader spectrum of tasks) are responsible for categorizing and attributing initial data points according to predefined guidelines and standards.
These individuals come from diverse backgrounds, ranging from computer science and engineering to linguistics and biology. While some tasks may not require specialized knowledge, demanding only common sense and meticulous attention to detail, the data's complexity and the project's objectives often dictate a certain level of expertise data annotation professionals must possess.
Types of Data Annotation Tasks
Data annotation and labeling tasks vary depending on the format and complexity of the initial data. Most can be performed online, while some may require field research or physical presence at specific locations. For example, tasks may involve capturing images of parking meters in a particular area or verifying the opening hours of local grocery stores.
We will review some of the data annotation methods, categorizing projects based on the types of data they are based on.
Image Annotation
Image annotation comprises labeling entire pictures, regions within them, or separate objects, providing essential context for AI algorithms. The latter may be involved in object recognition and scene comprehension tasks and require different levels of immersion into the depicted scene.
Labelers may be entrusted with deciding which of the provided pictures better meets suggested guidelines, outlining explicit content, or simply tagging every image containing a bicycle. Other commonly utilized techniques, like bounding boxes, key points, polygons, and semantic segmentation, ensure accurate delineation of objects, enabling precise analysis.
Image Classification
This type of image annotation usually means that a labeler needs to tag an image as relevant or irrelevant to a description, containing or not containing a particular item, appropriate or inappropriate for children, etc.
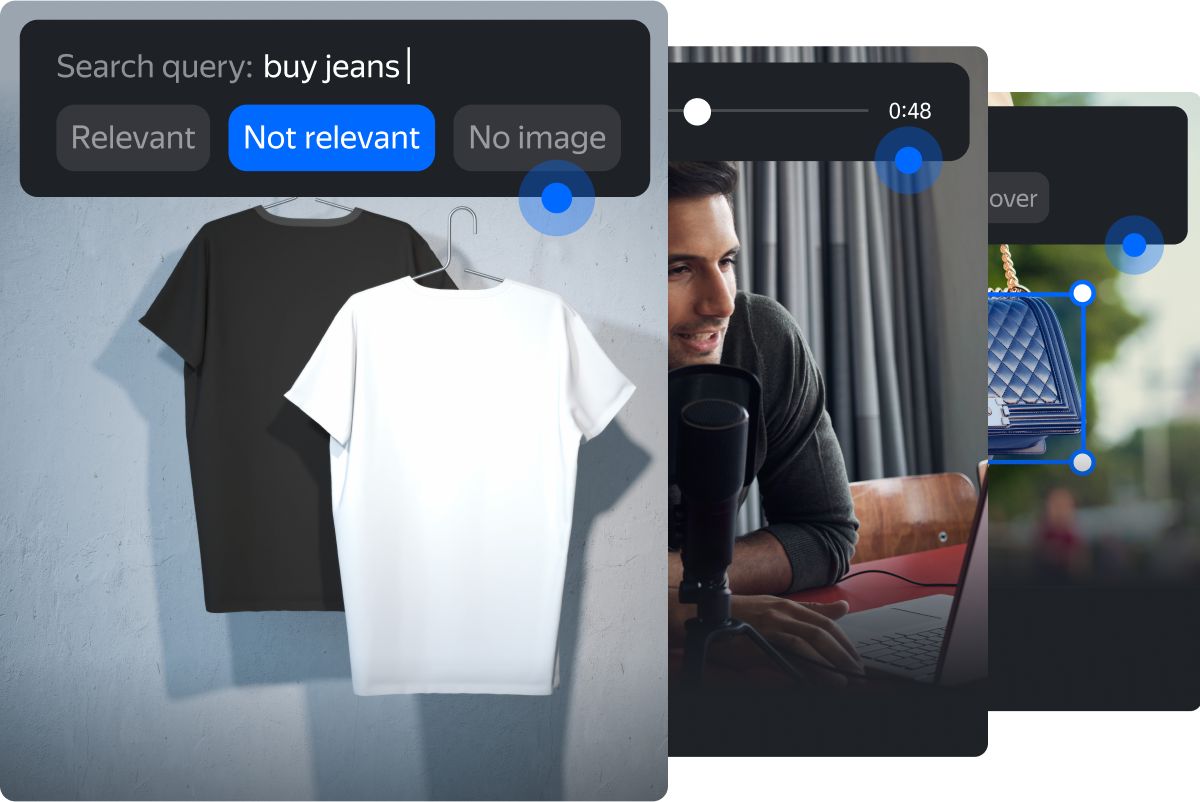
Typical image classification task. (Source: Toloka.ai)
In certain domains, image classification tasks may require high professional expertise. For instance, applying a tag and identifying abnormalities in X-rays, MRIs, and CT scans necessitates a labeler's medical education, usually the qualification of a radiologist.
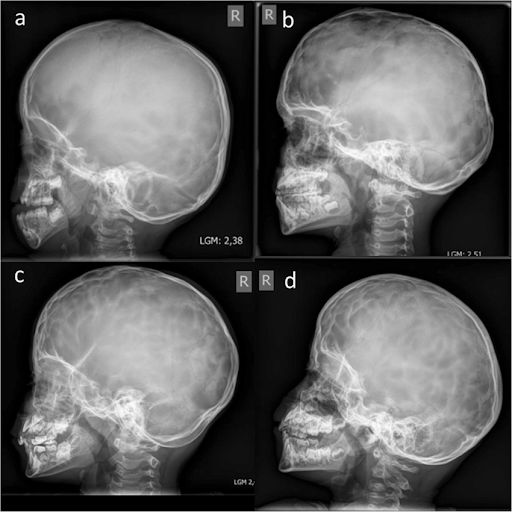
Examples of skull X-rays, grading from ‘No abnormalities’ (a) to ‘Severe abnormalities’ (d). The task of classifying such medical images can’t be fulfilled without extensive training and reliable certification. (Source: Child's Nervous System)
Object Recognition and Detection
This kind of visual data annotation encompasses more complex tasks of identifying various items and often includes locating them in the original image. Machine learning models for computer vision must be trained and tested on thousands of pictures similar to the input data the system will be processing in the future.
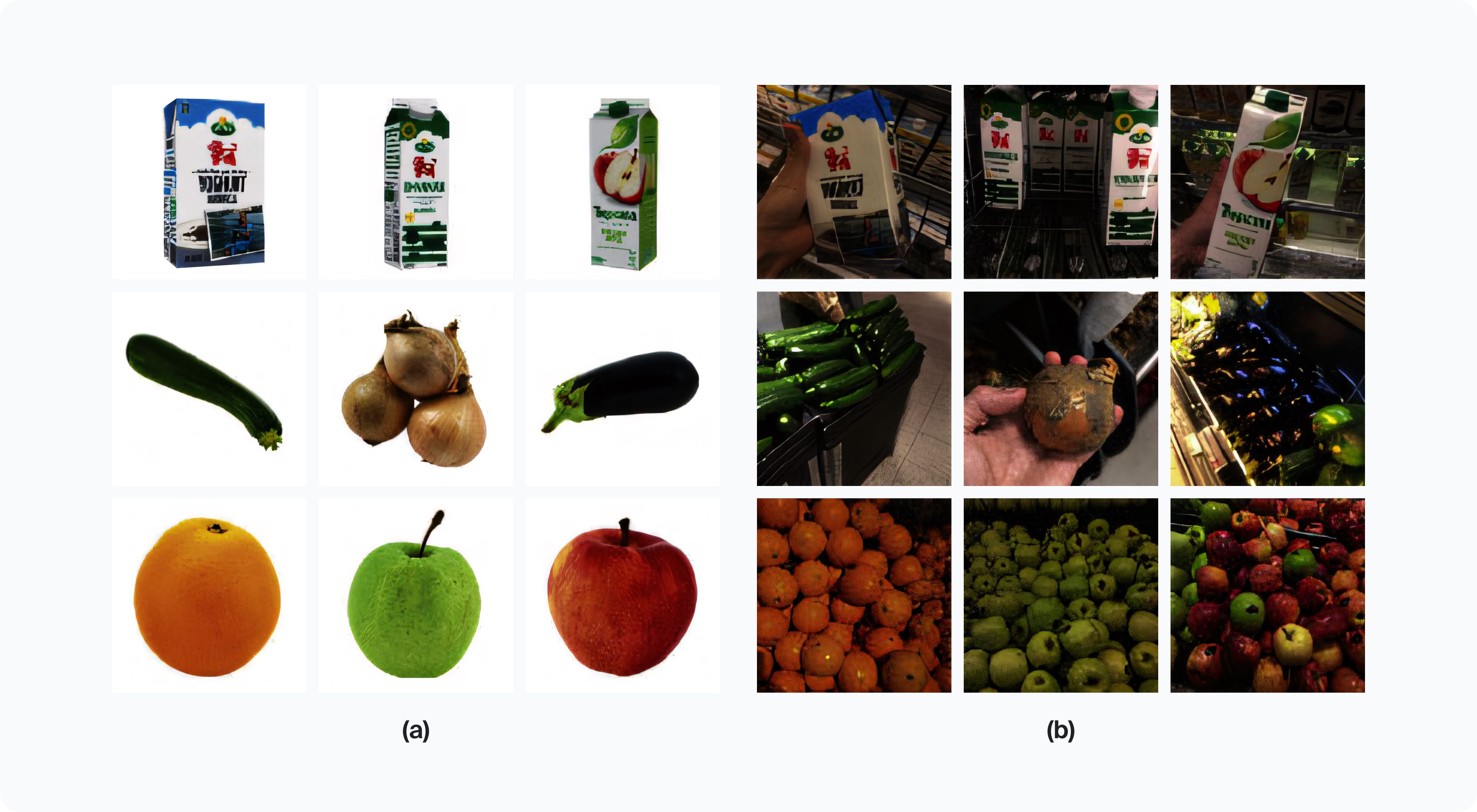
The difference between iconic samples in a training dataset and testing images made in real-life conditions. (Source: Semantic Scholar)
Data annotation specialists may tag, describe, and outline objects in massive datasets containing images of different qualities taken in various climate zones, weather conditions, and lighting.
Bounding Boxes, Polygons, Key Points, and Landmarks
Object detection tasks comprise multiple subcategories, such as defining shapes and sizes, as well as essential or edge points of particular items in an image. Depending on the project’s goals, this type of image annotation can take the form of marking all road signs in a picture with bounding boxes, outlining faces with polygons, or placing key points to detect athletes' poses.
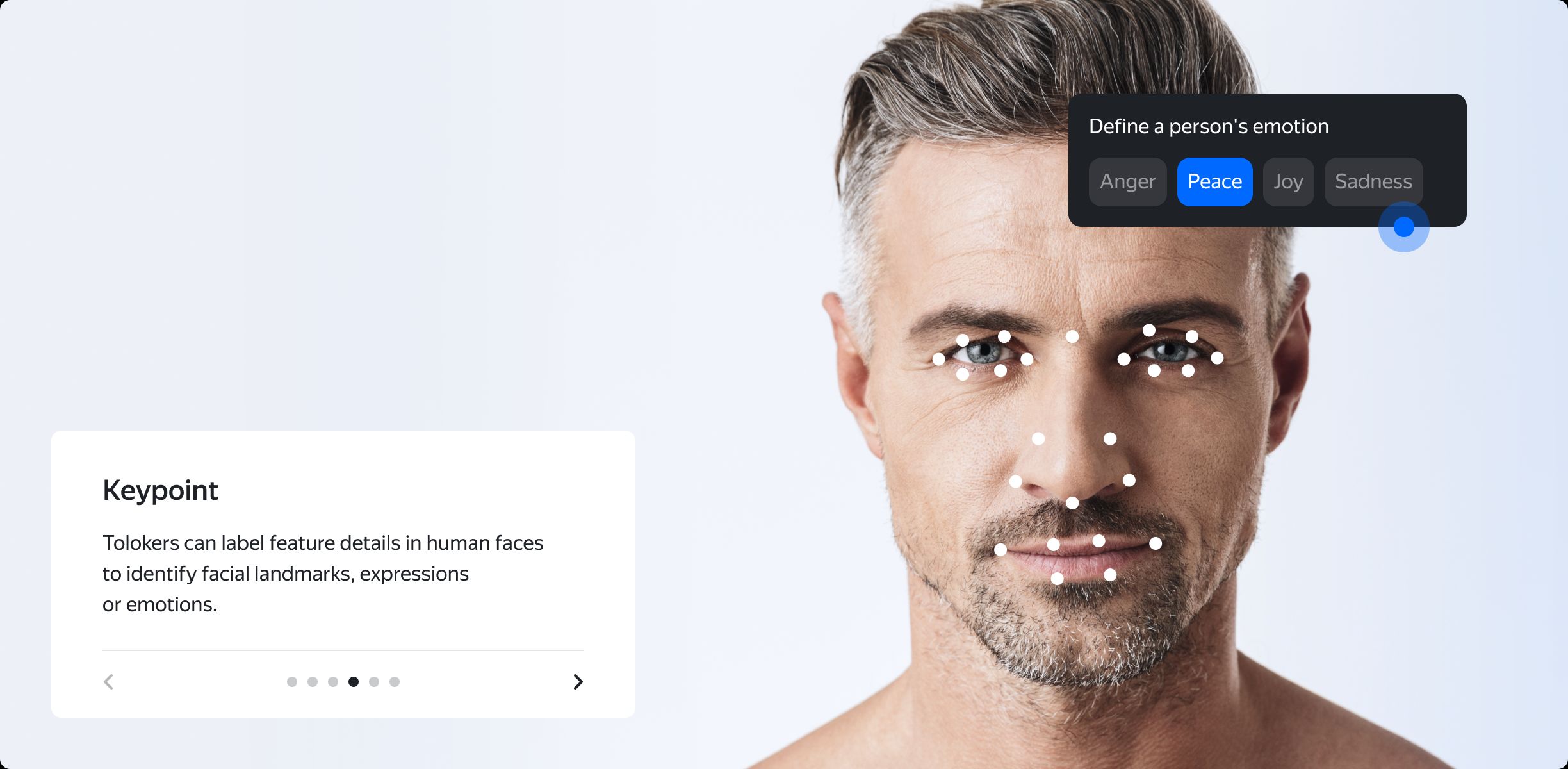
A complex image annotation task combining key point detection and image classification. (Source: Toloka.ai)
The complexity of such data annotation tasks varies, but low image quality and overlapping often make them much more difficult than one may suspect. Also, data labelers need to provide pixel-perfect precision and box-size consistency, which requires a certain experience.
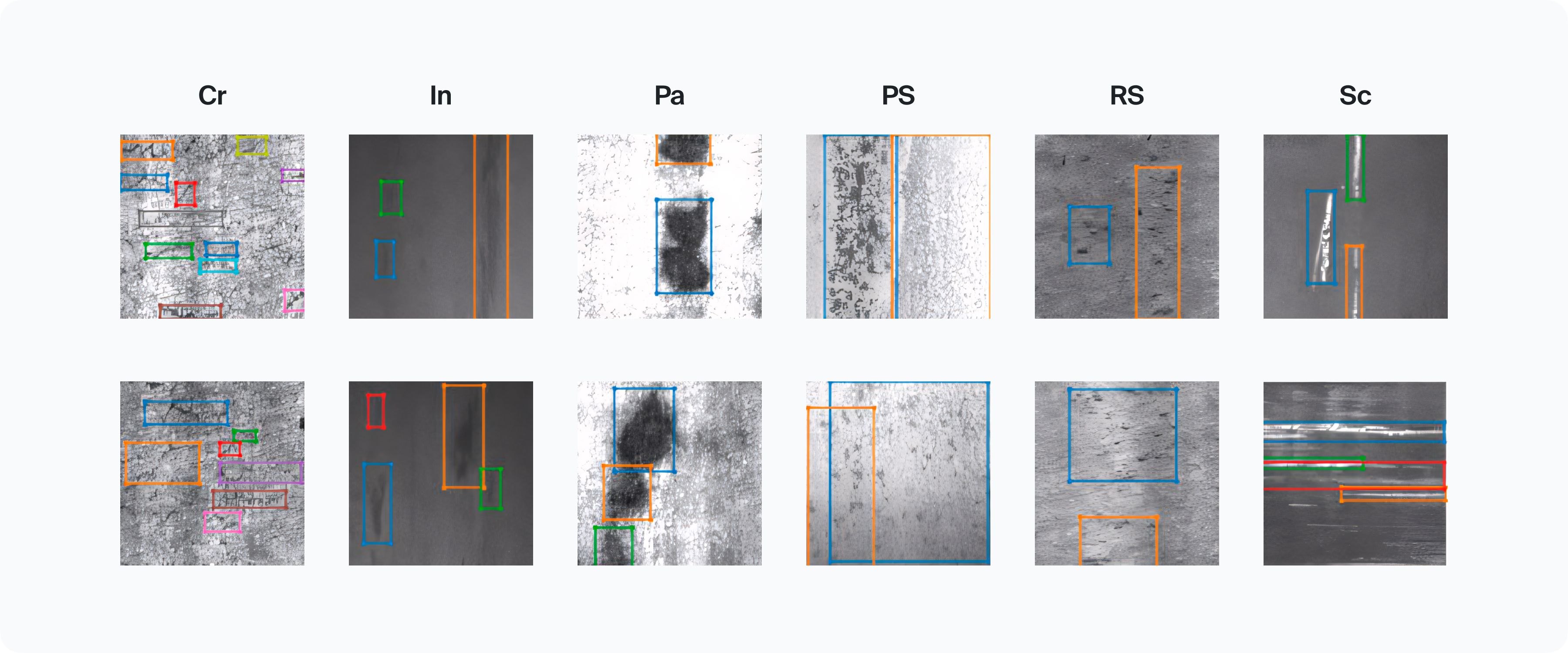
Examples of annotated steel strip defect images in the NEU-DET dataset. (Source: ResearchGate)
Other image annotation tasks strictly demand professional expertise. These include, for example, drawing bounding boxes of polygons around tumors on medical images or outlining defects on electronic components for quality control automation.
Semantic Segmentation
This kind of semantic annotation presumes image analysis at the pixel level, separating pictures into clusters of pixels relating to a corresponding object. Semantic segmentation divides images into multiple zones according to objects to which all these pixels belong.
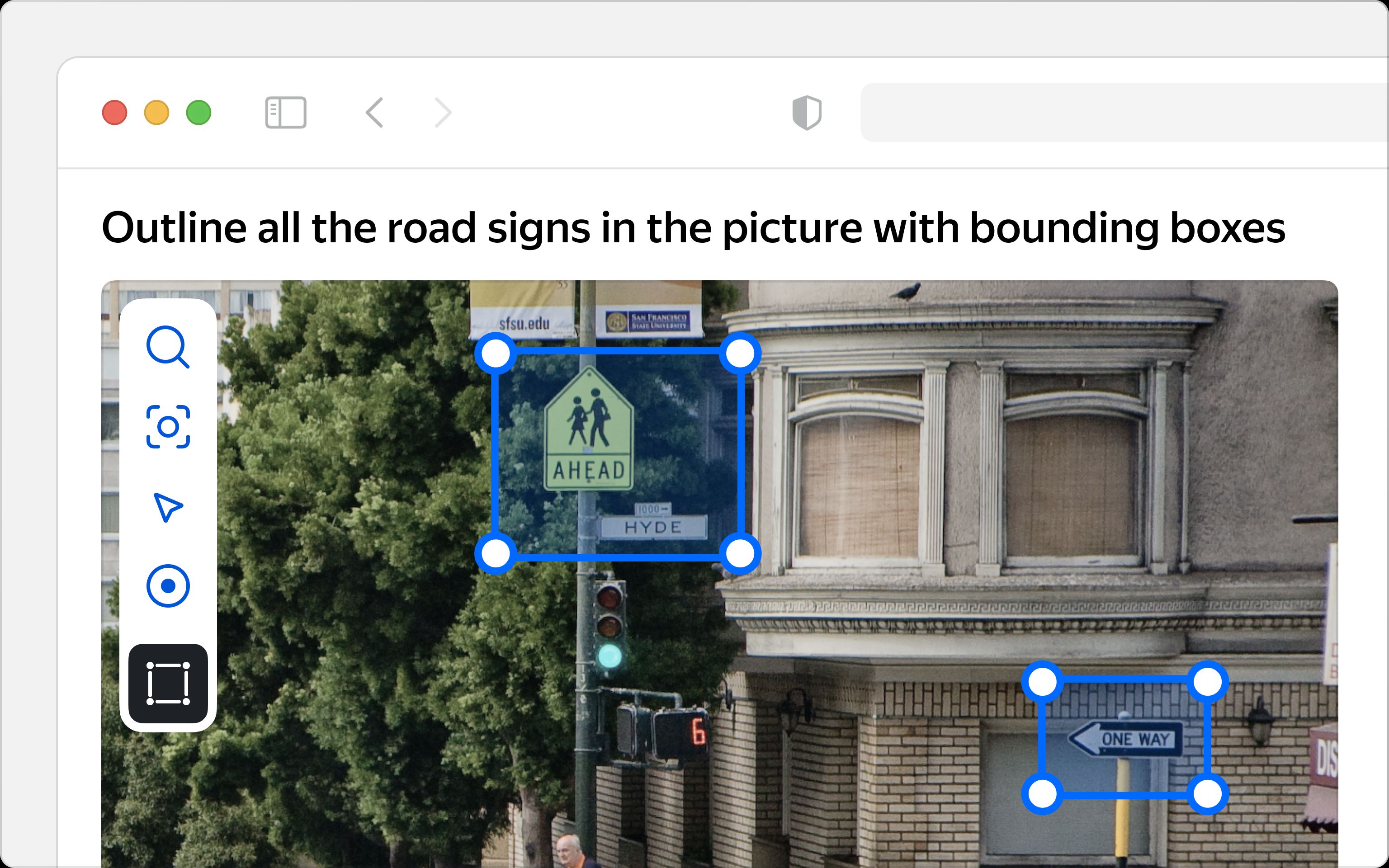
Semantic segmentation for training datasets is essential to complex computer vision models. (Source: Toloka.ai)
Such detailed image annotation allows machine learning models to get closer to understanding the natural environment. It makes semantic segmentation a crucial task for computer vision projects, including control systems for autonomous vehicles. To comprehend a scene, a computer must correlate all the objects in an image, making predictions of their potential interactions.
Side-by-Side Image Annotation
In this data annotation method, labelers typically receive pairs of images depicting similar scenes or objects with subtle differences. Their task involves marking corresponding features or objects in both images, aiding the machine learning model in comprehending the nuances between them.
This image annotation approach cultivates model resilience to variations in real-world scenarios, leading to robust and generalized performance across diverse conditions.
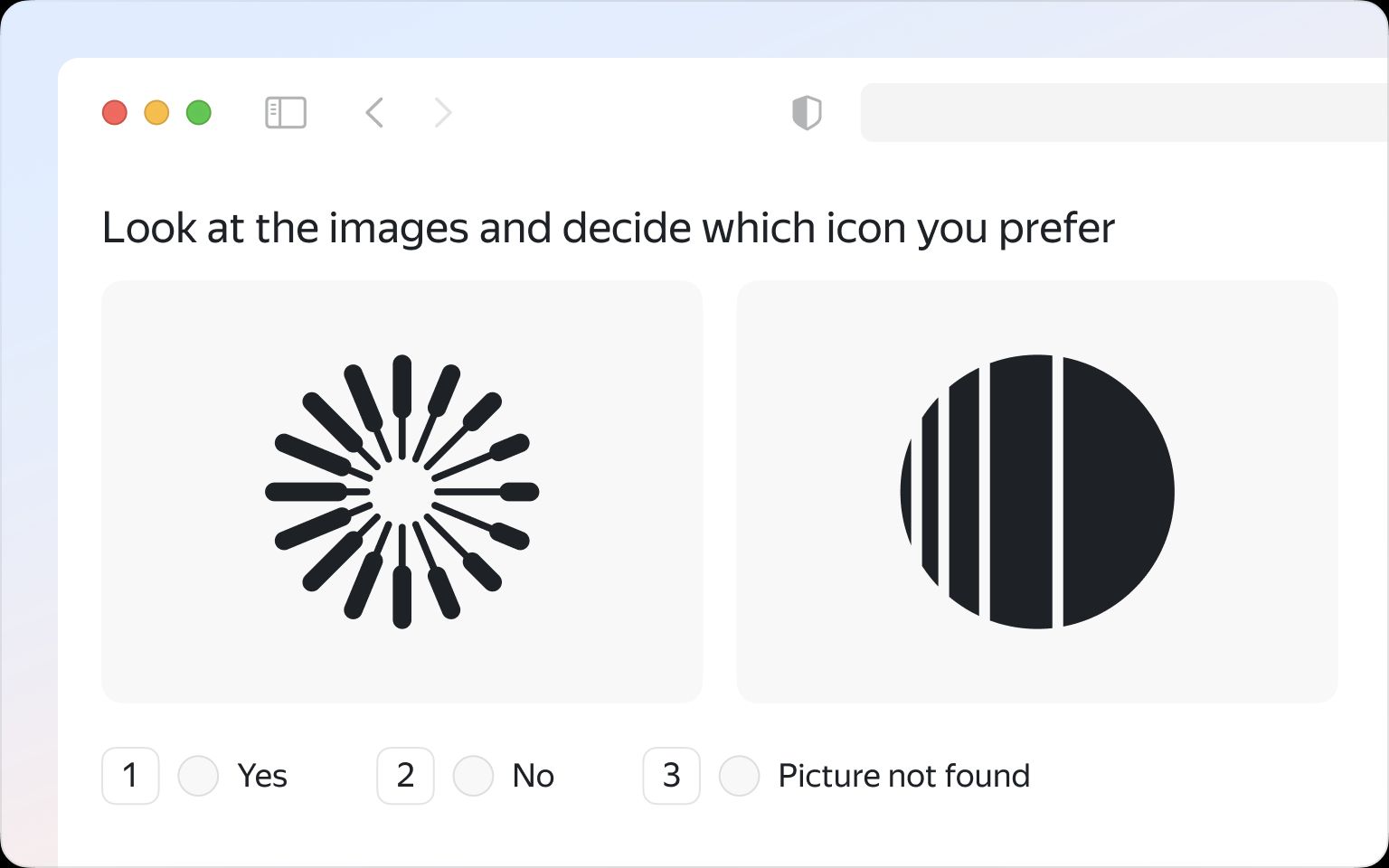
Side-by-side data labeling approach can improve the model's ability to anticipate user preferences or reactions. (Source: Toloka.ai)
Beyond mere feature identification, side-by-side annotation methods also encompass subjective judgment based on preferences or overall impressions. Training models on datasets annotated in this manner helps them understand human perceptions, enriching their capacity for tasks like content recommendation or targeted content creation.
Video Annotation
Video data annotation methods involve meticulously labeling entire frames, specific regions within frames, or individual objects, thus furnishing crucial context for a machine learning algorithm. These algorithms often engage in action recognition, scene understanding, or object tracking tasks.
Annotators may delineate salient features or tag specific actions or objects throughout the video stream. They may also be tasked with discerning the most suitable visual content according to predefined criteria.
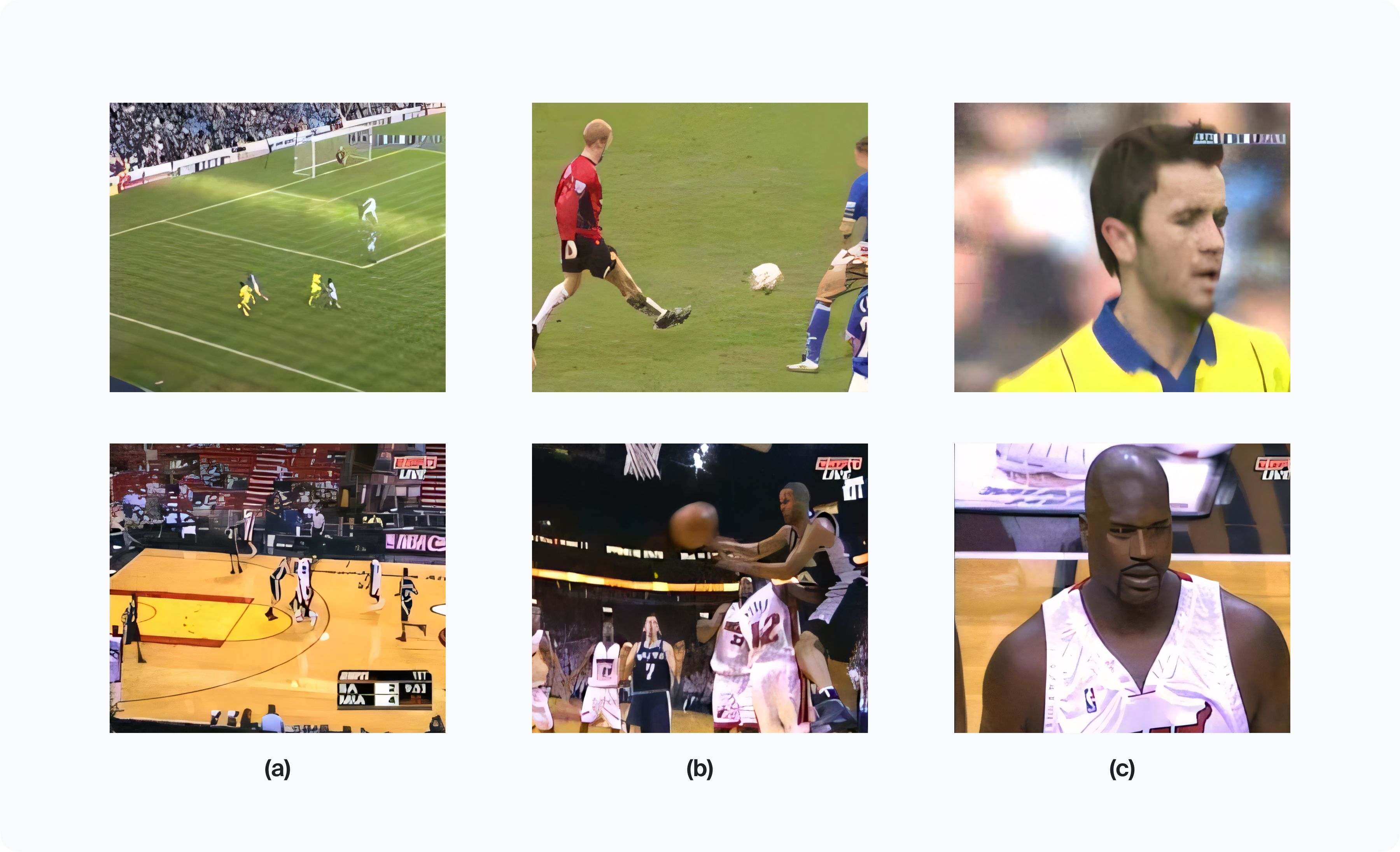
Video data annotation for sports analytics: examples of shot class. (a) Far view; (b) medium view; and (c) close-up. (Source: ResearchGate)
These annotations not only enhance algorithmic comprehension but also play a pivotal role in facilitating applications ranging from surveillance to action recognition in sports analytics. Video annotation tasks include but are not limited to those listed further.
Video Classification
Similar to image classification, this type of visual data annotation task requires attributing a video to a predefined category. It includes identifying inappropriate content for automated moderation or object detection for computer vision projects. Besides, it encompasses supplying footage with metadata, such as tags for event types or emotions.
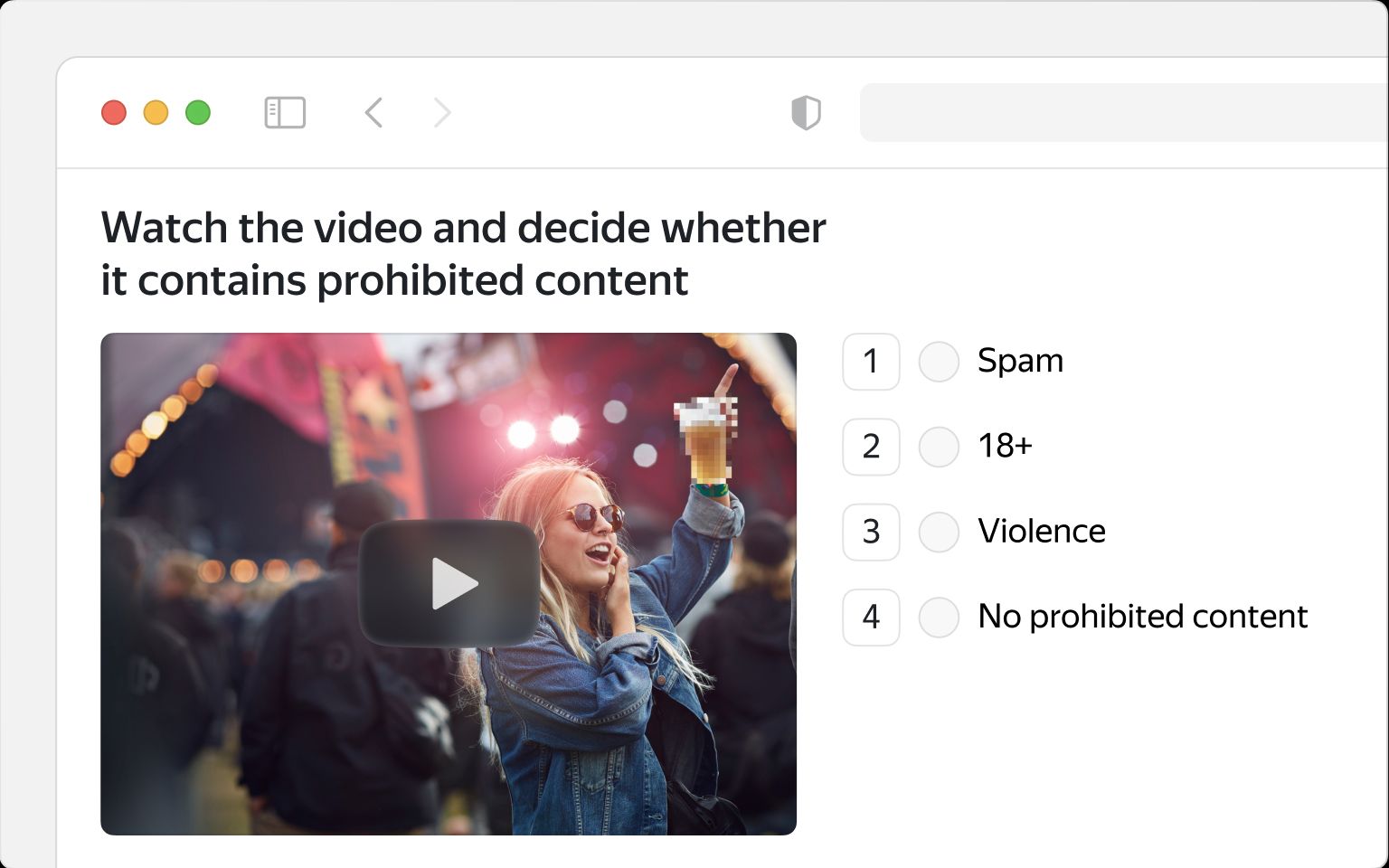
Typical video moderation task. (Source: Toloka.ai)
Video classification also comprises evaluating samples as relevant or irrelevant to a particular product or simply checking whether the video starts playing.
Instance Segmentation
This kind of video semantic annotation aims at the simultaneous detection, segmentation, and tracking of objects within videos. Annotators can split these objects by categories, for instance, for instance, to train the machine learning model to distinguish between a bicycle and a motorcycle, or tag them individually, for instance, to teach it recognize a particular athlete.
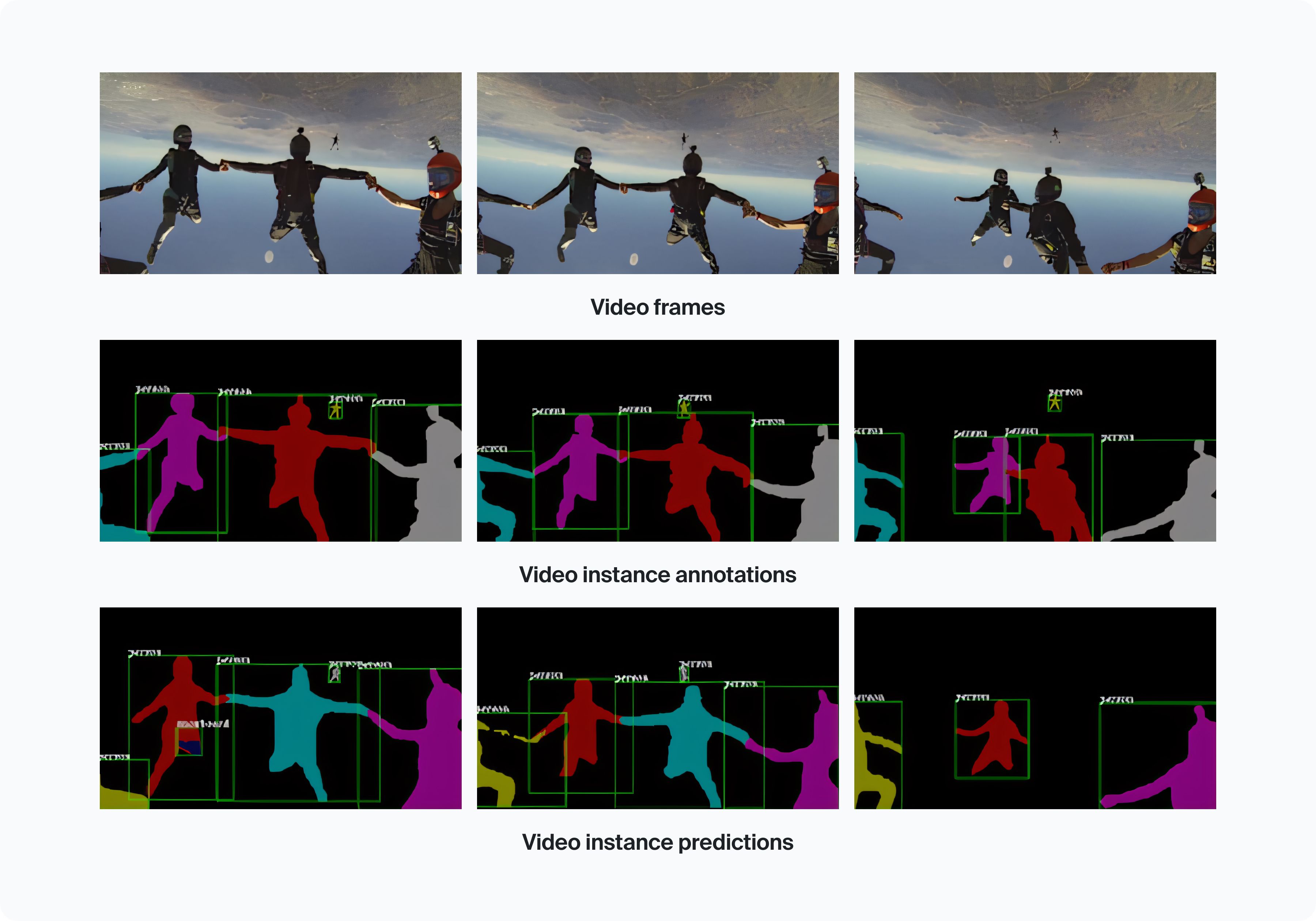
Video instance segmentation. (Source: Semantic Scholar)
Instance segmentation combines object segmentation tasks, aimed at tracking separate objects in motion, and object detection, focused on attributing these objects.
Lane Annotation
Lane annotation involves precisely marking lanes on roads within video footage, primarily aiding in autonomous vehicle navigation systems. Car manufacturers heavily rely on lane annotation to develop advanced driver assistance systems (ADAS) and autonomous driving technology.
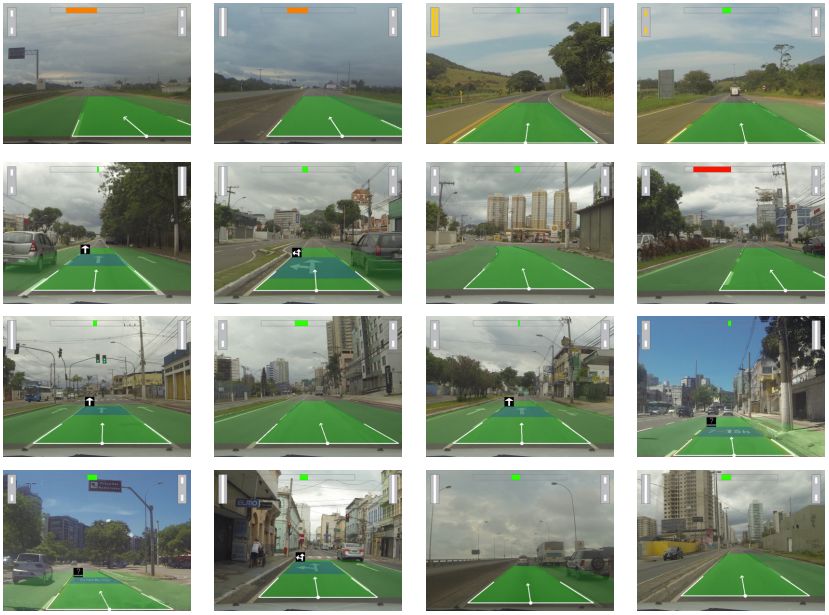
Samples from manually annotated ELAS dataset containing over 15,000 frames and considering various scenarios (urban road and highways, traffic, shadows, etc.). (Source: Papers with Code)
Accurate lane annotation ensures the proper understanding of road geometry and enhances the vehicle's ability to stay within lanes and navigate safely. It plays a pivotal role in improving the reliability and safety of self-driving cars and can also be applied to track rails or pipelines.
Cuboid Annotation
Cuboid, or 3D bounding box annotation, provides three-dimensional representations of objects within video footage. Unlike traditional 2D bounding boxes, cuboids offer insights into an object's depth, making them invaluable for projects requiring precise measurements and spatial understanding.
By annotating cuboids, systems can discern intricate features such as volume and spatial positioning within a three-dimensional space, enhancing object recognition and localization capabilities.
During cuboid annotation, labelers delineate rectangular boxes around target objects in three dimensions, defining key attributes such as length, width, and depth. Placing anchor points on the edges of an object may be tangled when the latter is only partially visible within a video frame.
Side-by-Side Video Annotation
Like any side-by-side tasks, those concerning videos are about identifying which of the two samples is more relevant or closer to guidelines.
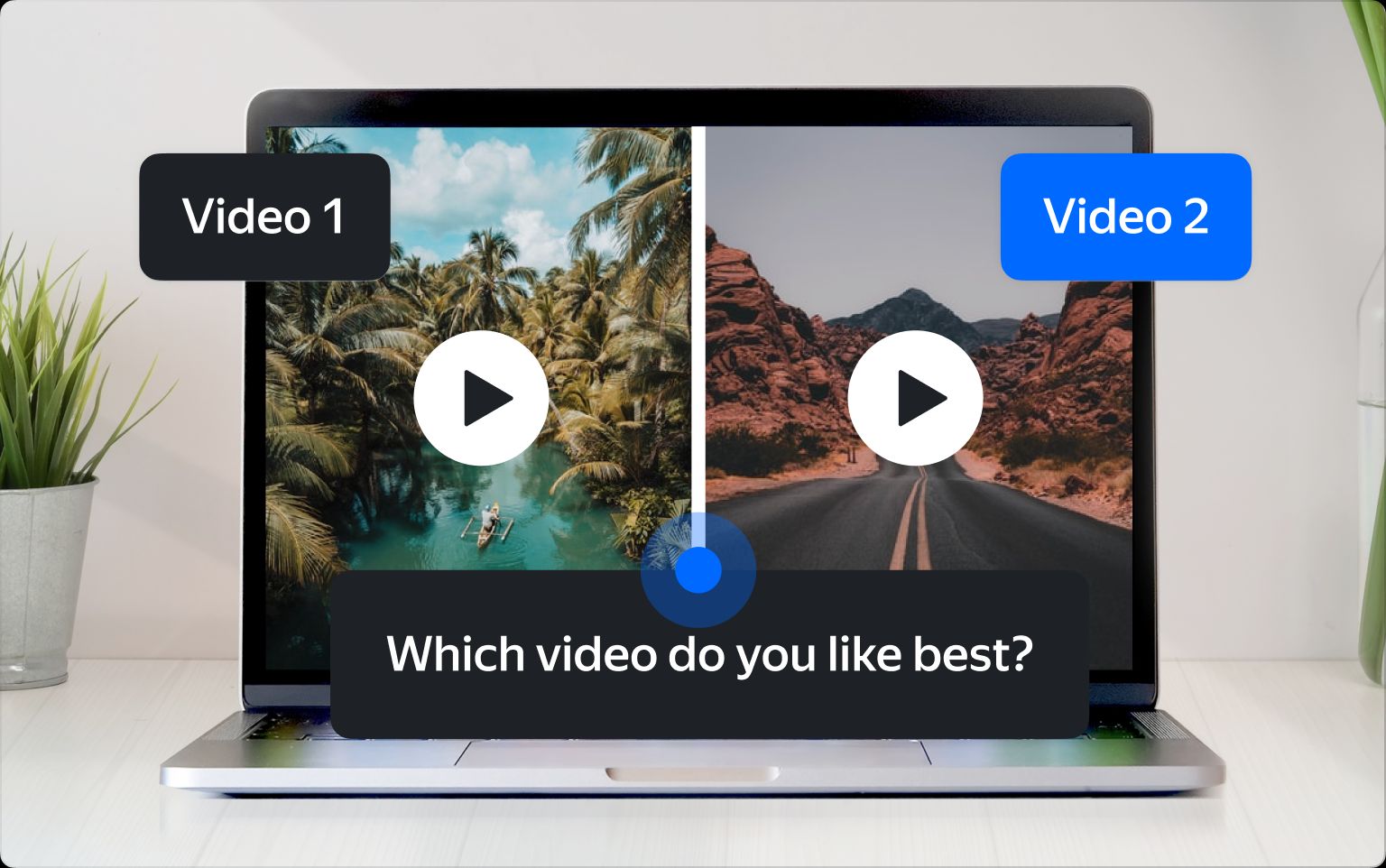
Usual side-by-side video annotation task. (Source: Toloka.ai)
Annotators can compare long footage and short animations, choose the best opening frames, identify which of the videos is in English, or decide which one looks more realistic.
Audio Annotation
Audio data labeling encompasses various tasks crucial for machine learning applications, enabling such models to comprehend various records and distinguish particular sounds.
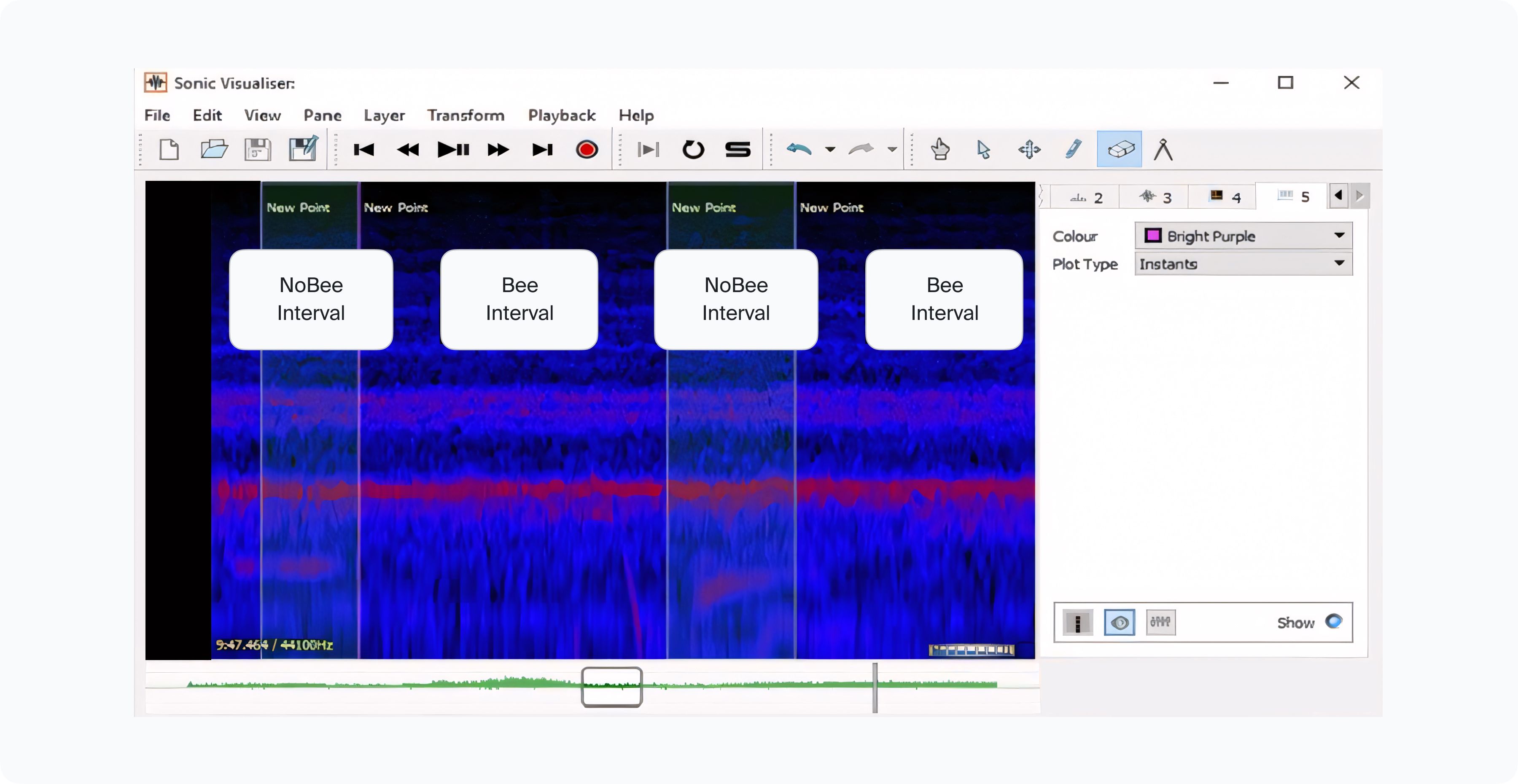
Annotation procedure in an experiment. aimed at identifying beehive sounds using deep learning. (Source: Research Gate)
Audio Classification
Annotators can assign recordings to predefined classes, categorizing language, connotation, speaker characteristics, background noises, intentions, or semantics. This classification enables machine learning model training for voice recognition or sentiment analysis.
Music Classification
It relies on sound annotation to categorize tracks by genre or instruments, facilitating personalized music recommendations and efficient organization of music libraries.
Audio Transcription
Converting audio files into text enables their further annotation and analysis. Despite challenges like background noise or language accents, transcription is vital for training machine learning models to comprehend and interpret human speech. It may also encompass distinguishing and tagging various sounds besides spoken words, such as sneezing or whistling a tune.
Text Annotation
Text annotation also encompasses attributing data into predefined categories, enabling sentiment analysis, text moderation, topic modeling, or spam detection. Besides that, annotating data in text copies include some specific tasks.
Named entity recognition
NER annotates text to identify and classify named entities such as people, organizations, locations, dates, or numerical expressions. This annotation task is crucial for applications like information extraction, question-answering systems, or language understanding tasks.
Text summarization annotation
Annotators identify and label the most significant phrases within a text, enabling the training of machine learning models to generate accurate summaries automatically.
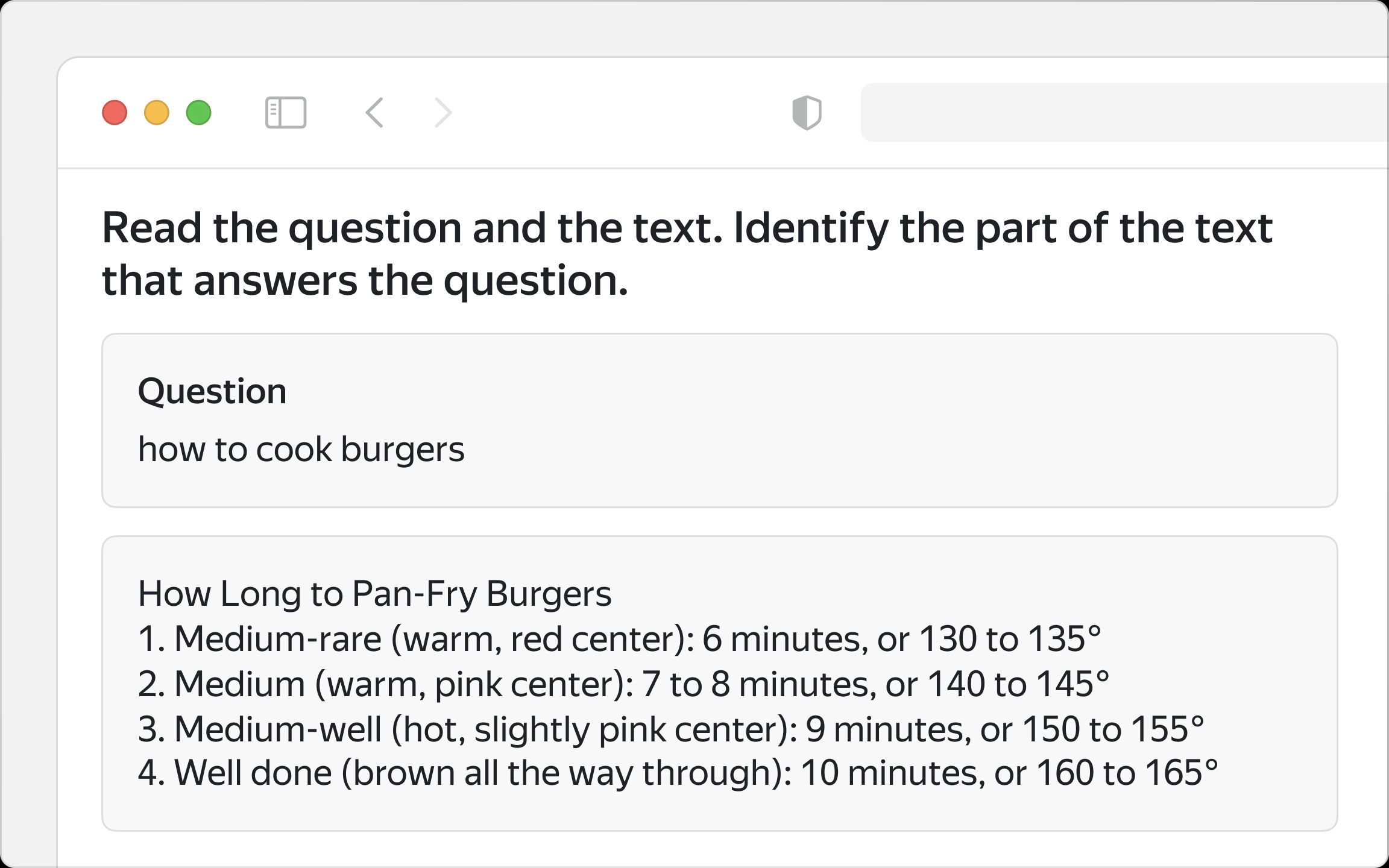
Question answering is another type of text-based data annotation tasks. (Source: Toloka.ai)
Data Annotators and Hybrid Labeling
Manual data annotation process for machine learning is complex and time-consuming. However, machine learning systems' heavy reliance on the quality of their training data often precludes fully automated labeling methods.
Nevertheless, adaptable strategies tailored to specific project objectives, including engagement with outsourcing partners allow businesses to streamline costs.
Large Language Models (LLMs), neural networks comprising over a billion parameters, have significantly influenced data annotation practices. Trained on vast unlabeled datasets, LLMs possess a capability similar to human annotators, facilitating the attribution of raw data for training new machine learning models. However, continuous validation of their output remains imperative.
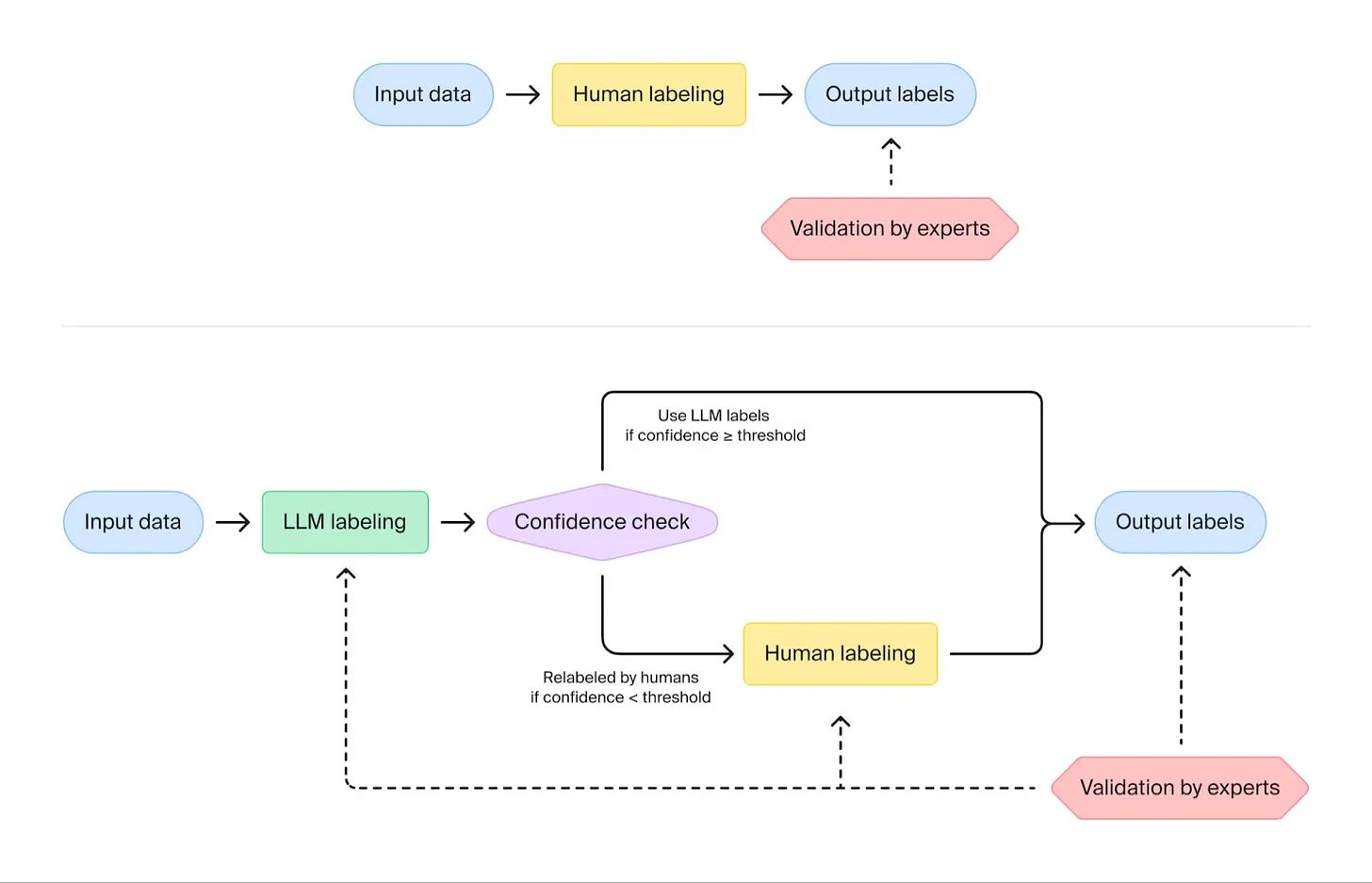
Hybrid labeling pipeline. (Source: Toloka.ai)
Hybrid labeling pipelines combine the efficiency of LLMs with the reliability of human annotators, often enhancing both aspects. Balancing these two methodologies necessitates substantial expertise but promises to reduce the cost of training data preparation while elevating quality standards.
Hybrid labeling takes advantage of LLMs' rapid tagging capabilities, incorporating a confidence level to outline the model's certainty in its decisions. Data labeled with low confidence levels are subsequently forwarded to human annotators for reevaluation, with the entire pipeline's flexibility adjusted to each project's unique requirements.
The prioritization between cost efficiency and precision in data annotation depends on the domain and the system's specific purpose. While substantial investment to elevate labeling quality may be indispensable in mission-critical sectors such as healthcare or transportation, applications in the entertainment industry may opt for cost-reduction strategies.
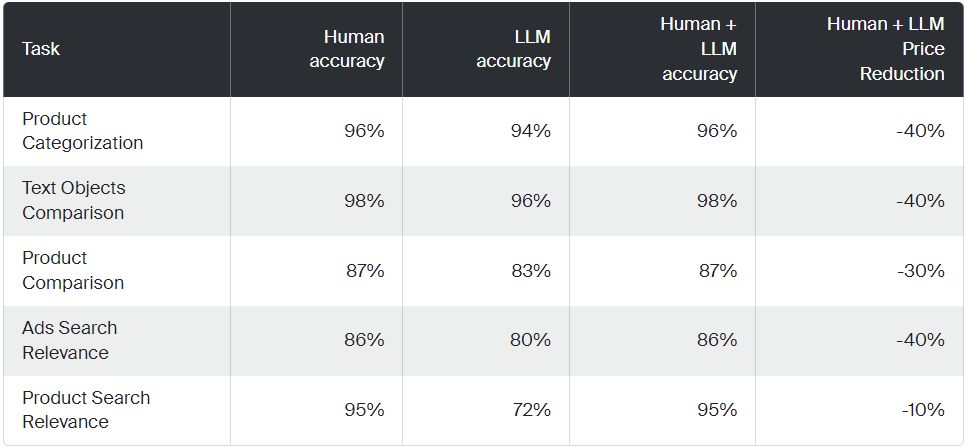
Results for cost-optimized pipelines with price reduction including only human labor and inference costs. (Source: Toloka.ai)
Opportunities and Demand for Annotators
While some data labeling tasks may only require basic computer literacy, others demand specialized knowledge and skills. For instance, annotating medical images or legal documents necessitates domain-specific expertise to ensure accuracy and reliability. Additionally, proficiency in relevant tools and technologies can be essential for the role.
The demand for professional services creates career opportunities for people involved in data annotation throughout the world. While entry level positions can be affected by further development of automated tools, the need for qualified annotators and domain experts will be growing together with the number of AI and ML projects launched.
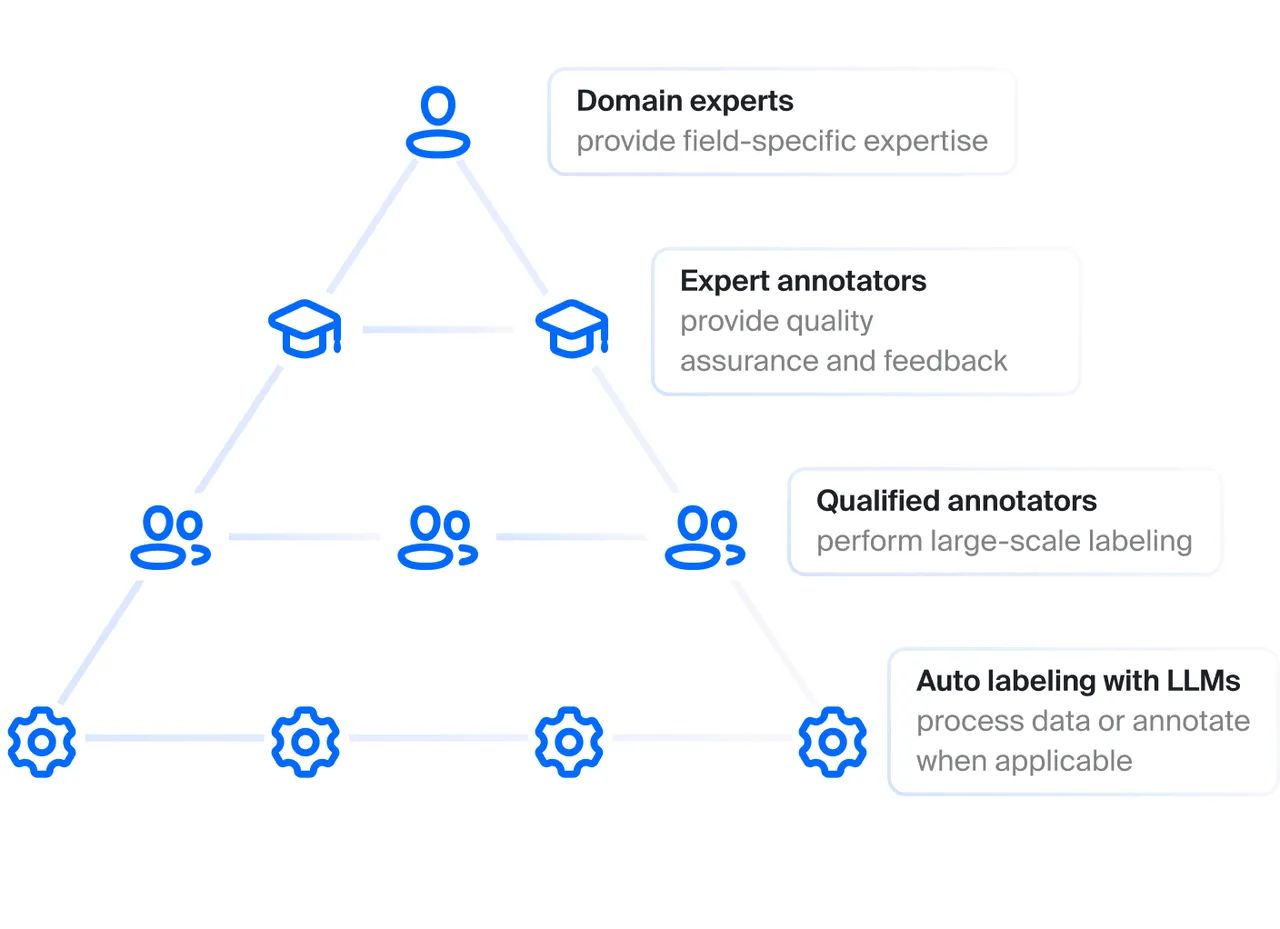
Toloka’s approach to human annotators’ involvement in data labeling. (Source: Toloka.ai)
Data annotation platforms like Toloka offer adaptable terms to individuals from diverse backgrounds and expertise levels. This approach appeals to those seeking new career opportunities and professionals interested in expanding their understanding of artificial intelligence while supplementing their income without diverting from their primary career trajectory.
Final Thoughts
Labeling specialists are pivotal in converting initial data collection into high quality training data, serving as the foundation for supervised machine learning projects. Their role is sometimes unnoticeable; however, the task of data annotation is complex, demanding meticulous attention to detail and often requiring domain-specific expertise.
Large Language Models (LLMs) have revolutionized data annotation practices, but validating their output remains crucial to ensure accuracy and reliability. Hybrid labeling pipelines achieve maximum results by integrating the efficiency of LLMs with the reliability of human annotators. And the professionalism of annotating experts is crucial for this approach.
In conclusion, data annotation professionals are instrumental in unlocking the transformative potential of data. As the demand for annotated datasets continues to grow, the role of data labelers remains indispensable in shaping the future of machine learning and artificial intelligence.